Dynamic Subspace Estimation with Grassmannian Geodesics
arXiv (Cornell University)(2023)
摘要
Dynamic subspace estimation, or subspace tracking, is a fundamental problem in statistical signal processing and machine learning. This paper considers a geodesic model for time-varying subspaces. The natural objective function for this model is non-convex. We propose a novel algorithm for minimizing this objective and estimating the parameters of the model from data with Grassmannian-constrained optimization. We show that with this algorithm, the objective is monotonically non-increasing. We demonstrate the performance of this model and our algorithm on synthetic data, video data, and dynamic fMRI data.
更多查看译文
关键词
dynamic subspace estimation
AI 理解论文
溯源树
样例
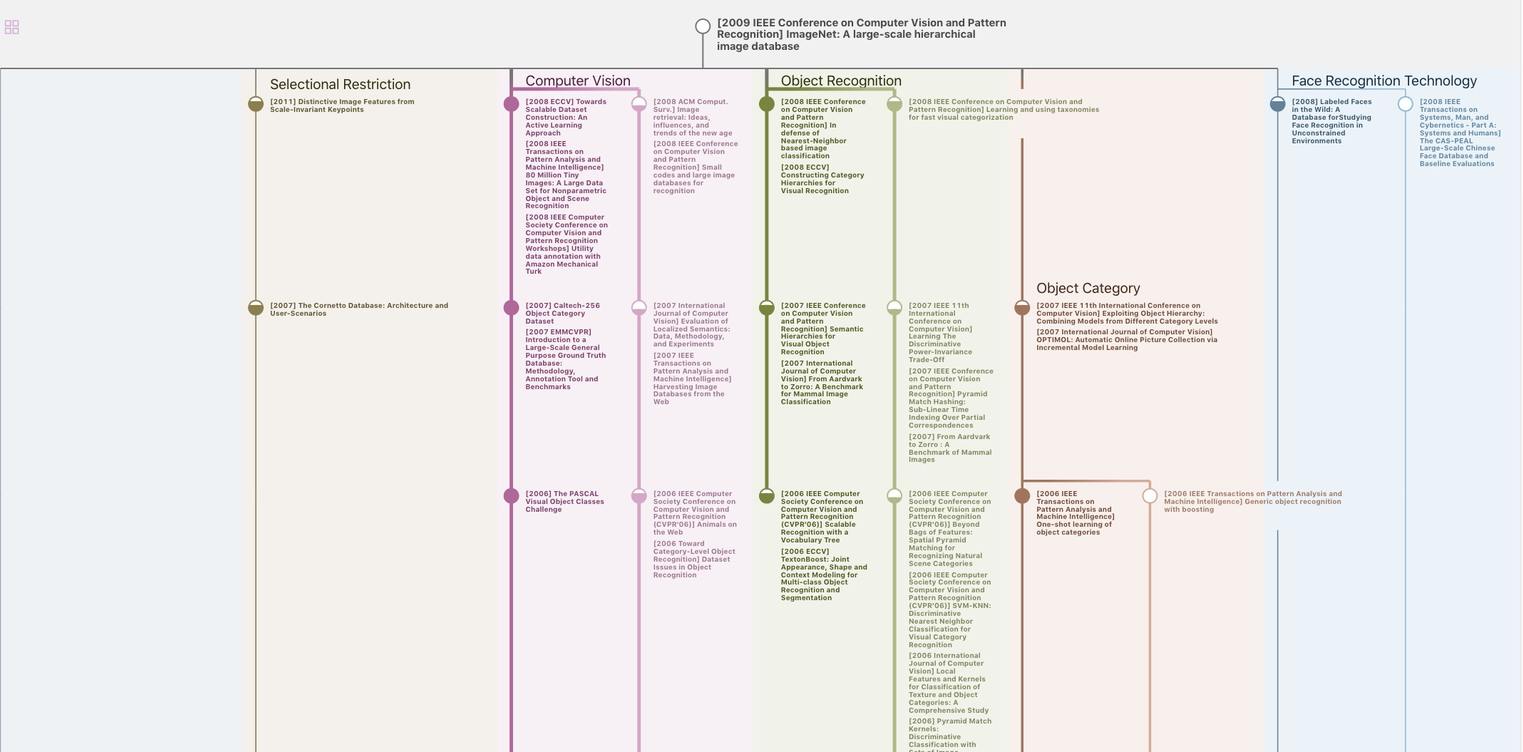
生成溯源树,研究论文发展脉络
Chat Paper
正在生成论文摘要