A Contrastive Learning Scheme with Transformer Innate Patches
ARTIFICIAL INTELLIGENCE XL, AI 2023(2023)
摘要
This paper presents Contrastive Transformer (CT), a contrastive learning scheme using the innate transformer patches. CT enables existing contrastive learning techniques, often used for image classification, to benefit dense downstream prediction tasks such as semantic segmentation. The scheme performs supervised patch-level contrastive learning, selecting the patches based on the ground truth mask, subsequently used for hard-negative and hard-positive sampling. The scheme applies to all patch-based vision-transformer architectures, is easy to implement, and introduces minimal additional memory footprint. Additionally, the scheme removes the need for huge batch sizes, as each patch is treated as an image. We apply and test CT for the case of aerial image segmentation, known for low-resolution data, large class imbalance, and similar semantic classes. We perform extensive experiments to show the efficacy of the CT scheme on the ISPRS Potsdam aerial image segmentation dataset. Additionally, we show the generalizability of our scheme by applying it to multiple inherently different transformer architectures. Ultimately, the results show a consistent increase in mean Intersection-over-Union (IoU) across all classes.
更多查看译文
关键词
Remote Sensing,Contrastive Learning,Deep Learning,Segmentation
AI 理解论文
溯源树
样例
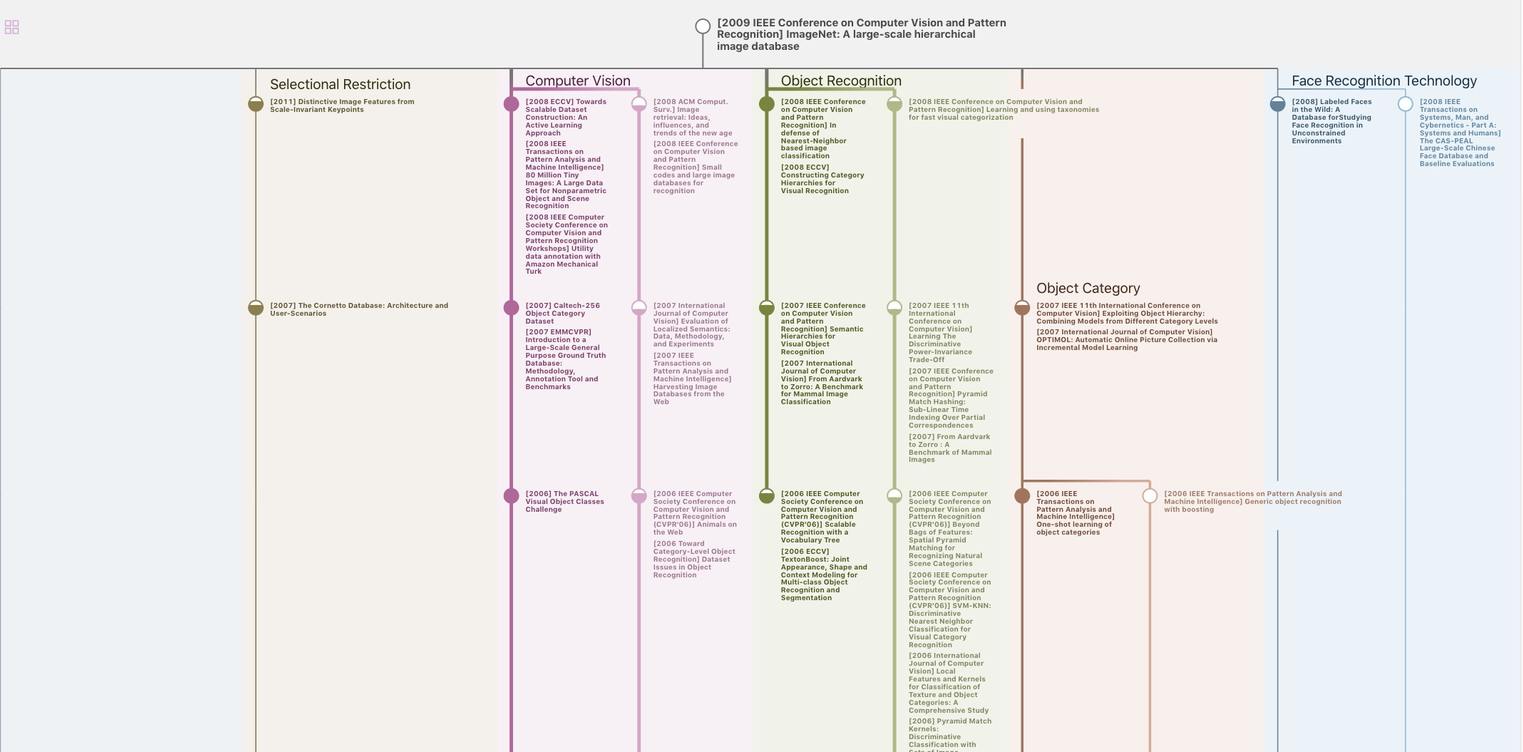
生成溯源树,研究论文发展脉络
Chat Paper
正在生成论文摘要