Federated Learning without Full Labels: A Survey
CoRR(2023)
摘要
Data privacy has become an increasingly important concern in real-world big data applications such as machine learning. To address the problem, federated learning (FL) has been a promising solution to building effective machine learning models from decentralized and private data. Existing federated learning algorithms mainly tackle the supervised learning problem, where data are assumed to be fully labeled. However, in practice, fully labeled data is often hard to obtain, as the participants may not have sufficient domain expertise, or they lack the motivation and tools to label data. Therefore, the problem of federated learning without full labels is important in real-world FL applications. In this paper, we discuss how the problem can be solved with machine learning techniques that leverage unlabeled data. We present a survey of methods that combine FL with semi-supervised learning, self-supervised learning, and transfer learning methods. We also summarize the datasets used to evaluate FL methods without full labels. Finally, we highlight future directions in the context of FL without full labels.
更多查看译文
关键词
federated learning,full labels
AI 理解论文
溯源树
样例
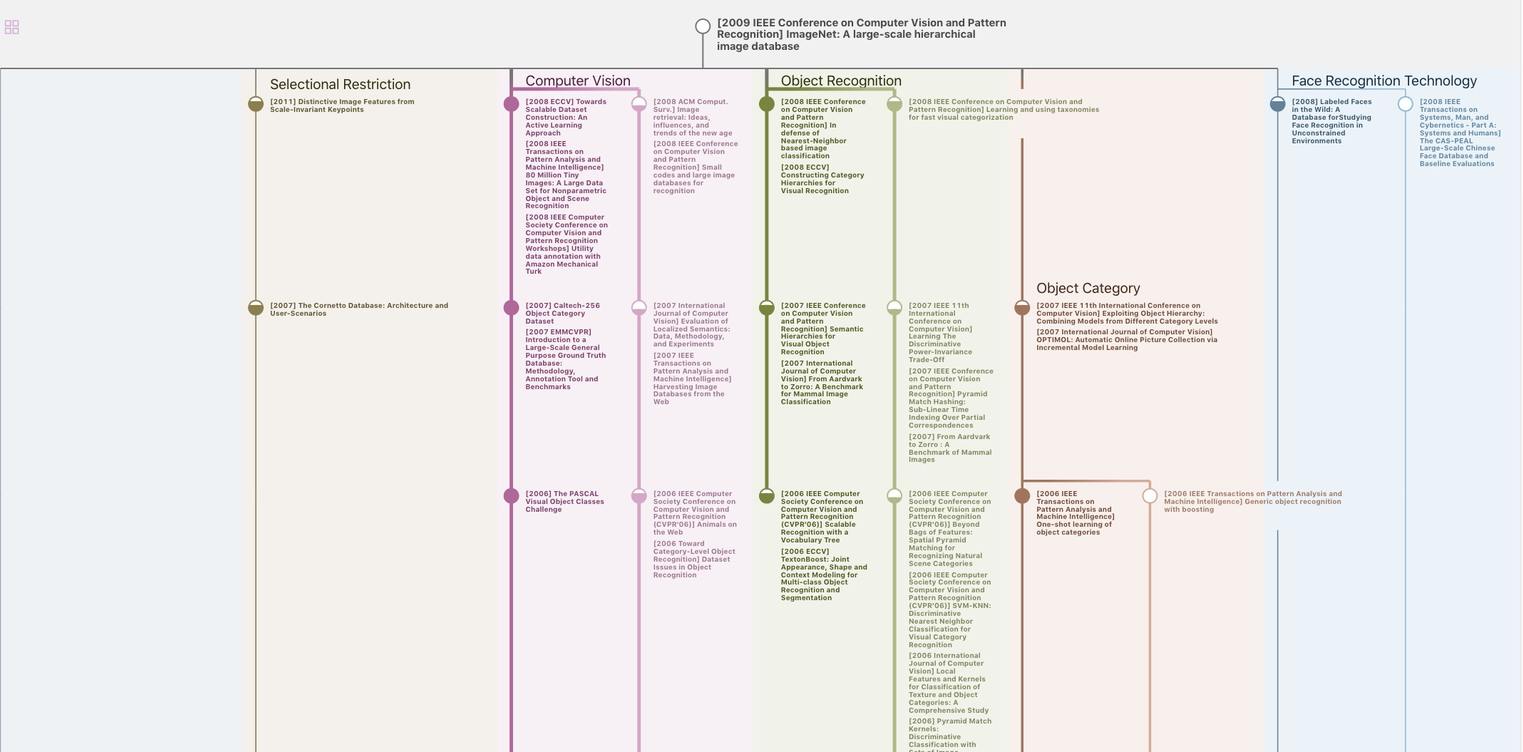
生成溯源树,研究论文发展脉络
Chat Paper
正在生成论文摘要