Heat flux for semi-local machine-learning potentials
CoRR(2023)
摘要
The Green-Kubo (GK) method is a rigorous framework for heat transport simulations in materials. However, it requires an accurate description of the potential-energy surface and carefully converged statistics. Machine-learning potentials can achieve the accuracy of first-principles simulations while allowing to reach well beyond their simulation time and length scales at a fraction of the cost. In this paper, we explain how to apply the GK approach to the recent class of message-passing machine-learning potentials, which iteratively consider semi-local interactions beyond the initial interaction cutoff. We derive an adapted heat flux formulation that can be implemented using automatic differentiation without compromising computational efficiency. The approach is demonstrated and validated by calculating the thermal conductivity of zirconium dioxide across temperatures.
更多查看译文
关键词
machine-learning machine-learning,heat,potentials,flux,semi-local
AI 理解论文
溯源树
样例
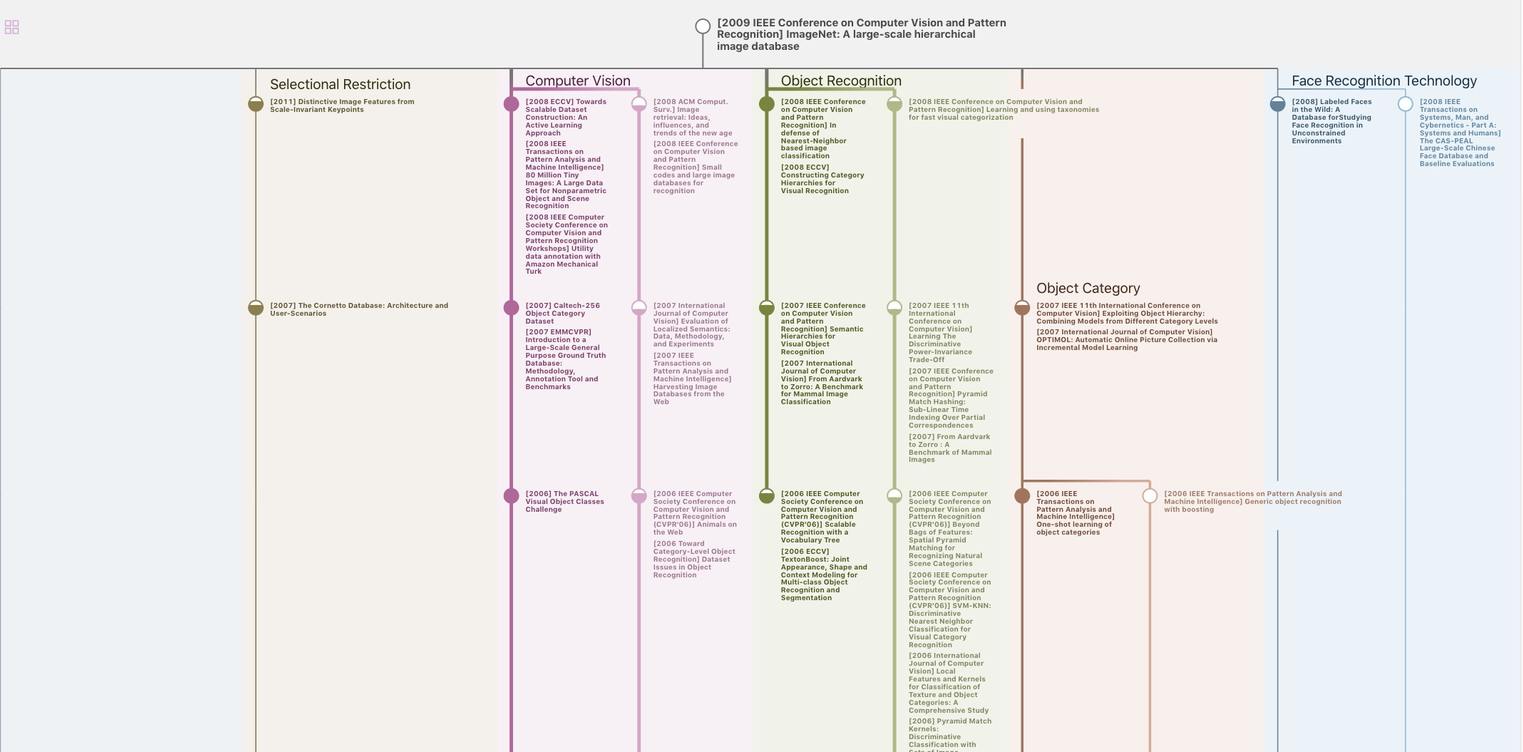
生成溯源树,研究论文发展脉络
Chat Paper
正在生成论文摘要