DoNet: Deep De-Overlapping Network for Cytology Instance Segmentation
CVPR 2023(2023)
摘要
Cell instance segmentation in cytology images has significant importance for biology analysis and cancer screening, while remains challenging due to 1) the extensive overlapping translucent cell clusters that cause the ambiguous boundaries, and 2) the confusion of mimics and debris as nuclei. In this work, we proposed a De-overlapping Network (DoNet) in a decompose-and-recombined strategy. A Dual-path Region Segmentation Module (DRM) explicitly decomposes the cell clusters into intersection and complement regions, followed by a Semantic Consistency-guided Recombination Module (CRM) for integration. To further introduce the containment relationship of the nucleus in the cytoplasm, we design a Mask-guided Region Proposal Strategy (MRP) that integrates the cell attention maps for inner-cell instance prediction. We validate the proposed approach on ISBI2014 and CPS datasets. Experiments show that our proposed DoNet significantly outperforms other state-of-the-art (SOTA) cell instance segmentation methods. The code is available at https://github.com/DeepDoNet/DoNet.
更多查看译文
关键词
Medical and biological vision,cell microscopy
AI 理解论文
溯源树
样例
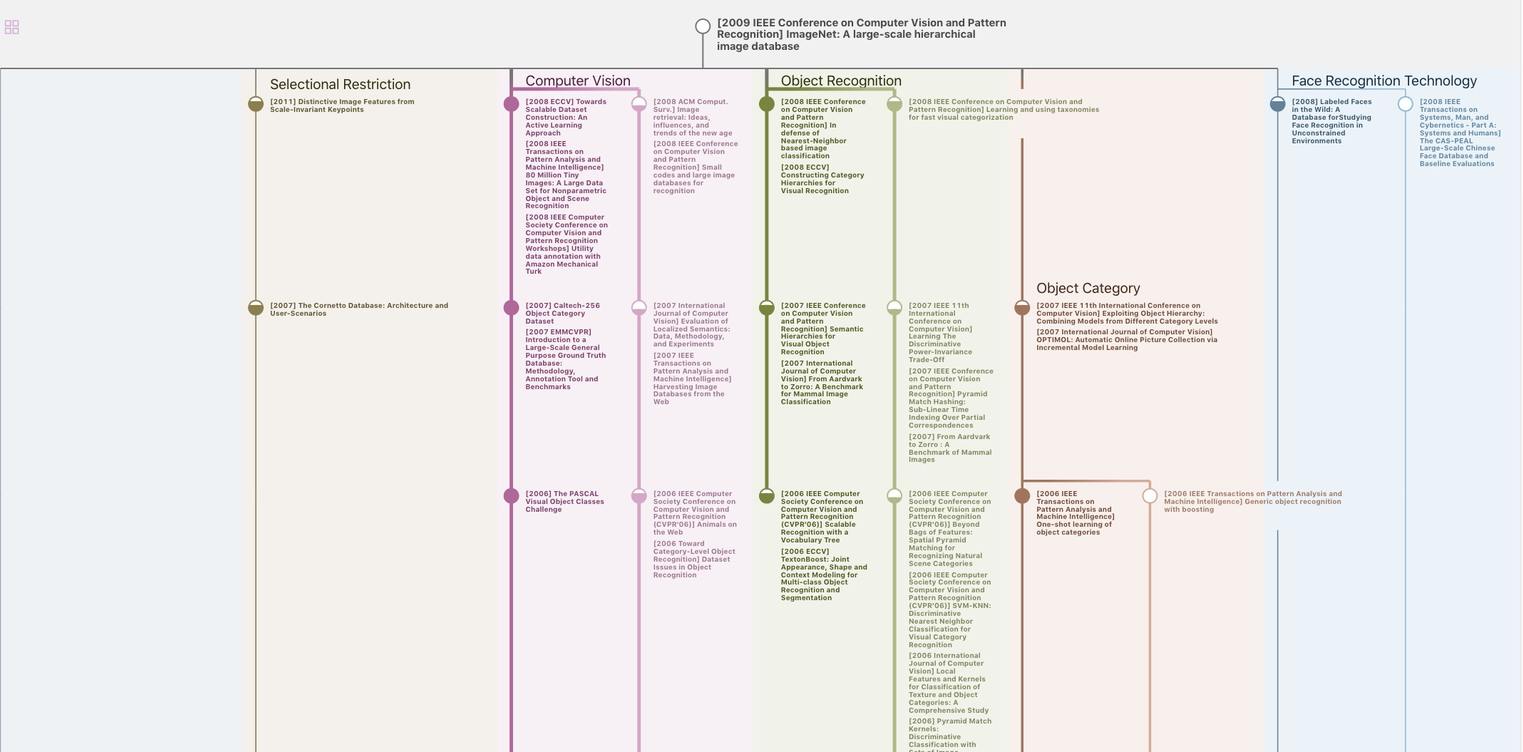
生成溯源树,研究论文发展脉络
Chat Paper
正在生成论文摘要