Towards Diverse and Coherent Augmentation for Time-Series Forecasting
CoRR(2023)
摘要
Time-series data augmentation mitigates the issue of insufficient training data for deep learning models. Yet, existing augmentation methods are mainly designed for classification, where class labels can be preserved even if augmentation alters the temporal dynamics. We note that augmentation designed for forecasting requires diversity as well as coherence with the original temporal dynamics. As time-series data generated by real-life physical processes exhibit characteristics in both the time and frequency domains, we propose to combine Spectral and Time Augmentation (STAug) for generating more diverse and coherent samples. Specifically, in the frequency domain, we use the Empirical Mode Decomposition to decompose a time series and reassemble the subcomponents with random weights. This way, we generate diverse samples while being coherent with the original temporal relationships as they contain the same set of base components. In the time domain, we adapt a mix-up strategy that generates diverse as well as linearly in-between coherent samples. Experiments on five real-world time-series datasets demonstrate that STAug outperforms the base models without data augmentation as well as state-of-the-art augmentation methods.
更多查看译文
关键词
Time Series,Data Augmentation,Forecasting,Decomposition,Spectral Analysis
AI 理解论文
溯源树
样例
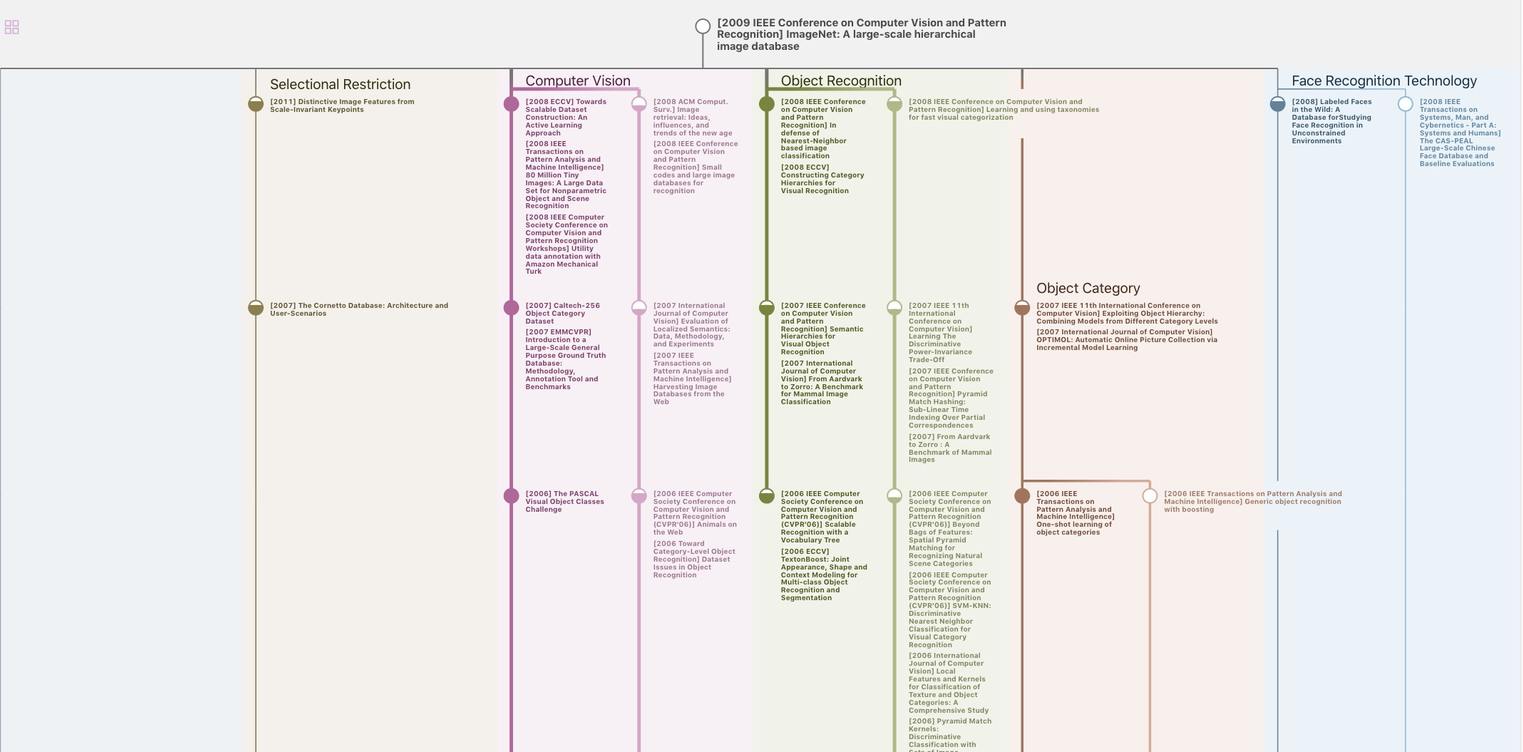
生成溯源树,研究论文发展脉络
Chat Paper
正在生成论文摘要