Crack Growth Rate Model Derived from Domain Knowledge-Guided Symbolic Regression
Chinese Journal of Mechanical Engineering(2023)
摘要
Machine learning (ML) has powerful nonlinear processing and multivariate learning capabilities, so it has been widely utilised in the fatigue field. However, most ML methods are inexplicable black-box models that are difficult to apply in engineering practice. Symbolic regression (SR) is an interpretable machine learning method for determining the optimal fitting equation for datasets. In this study, domain knowledge-guided SR was used to determine a new fatigue crack growth (FCG) rate model. Three terms of the variable subtree of Δ K , R -ratio, and Δ K th were obtained by analysing eight traditional semi-empirical FCG rate models. Based on the FCG rate test data from other literature, the SR model was constructed using Al-7055-T7511. It was subsequently extended to other alloys (Ti-10V-2Fe-3Al, Ti-6Al-4V, Cr-Mo-V, LC9cs, Al-6013-T651, and Al-2324-T3) using multiple linear regression. Compared with the three semi-empirical FCG rate models, the SR model yielded higher prediction accuracy. This result demonstrates the potential of domain knowledge-guided SR for building the FCG rate model.
更多查看译文
关键词
Fatigue crack growth rate,Stress intensity factor range,Threshold stress intensity factor range,R-ratio,Symbolic regression,Machine learning
AI 理解论文
溯源树
样例
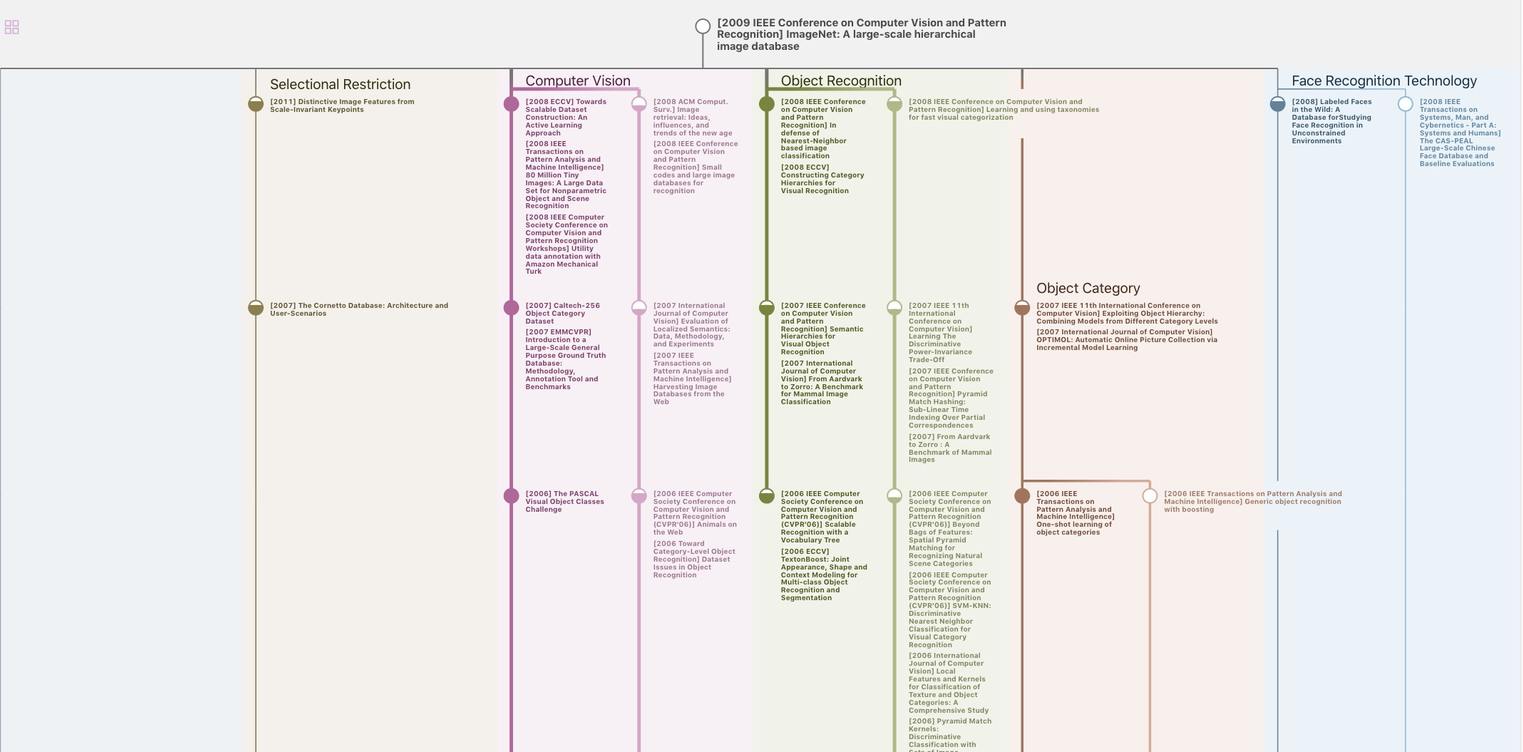
生成溯源树,研究论文发展脉络
Chat Paper
正在生成论文摘要