ADEPT: Anomaly Detection, Explanation and Processing for Time Series with a Focus on Energy Consumption Data
ECML/PKDD (6)(2023)
摘要
Anomaly detection techniques are applicable for recognizing excessive energy consumption and device failure, thereby contributing to the maintenance of operational and sustainable energy supply systems. In this context, human decision makers can benefit from receiving explanation attempts for detected anomalies as part of a semi-automated software solution. Therefore we introduce the framework ADEPT, which comprises interfaces for processing user-supplied time series data and interactively visualizing explanatory anomaly information. Our framework features several shallow and deep machine learning algorithms for anomaly detection and explanation. We demonstrate ADEPT using energy consumption data collected from our university campus.
更多查看译文
关键词
Anomaly detection, Energy consumption, Explainability
AI 理解论文
溯源树
样例
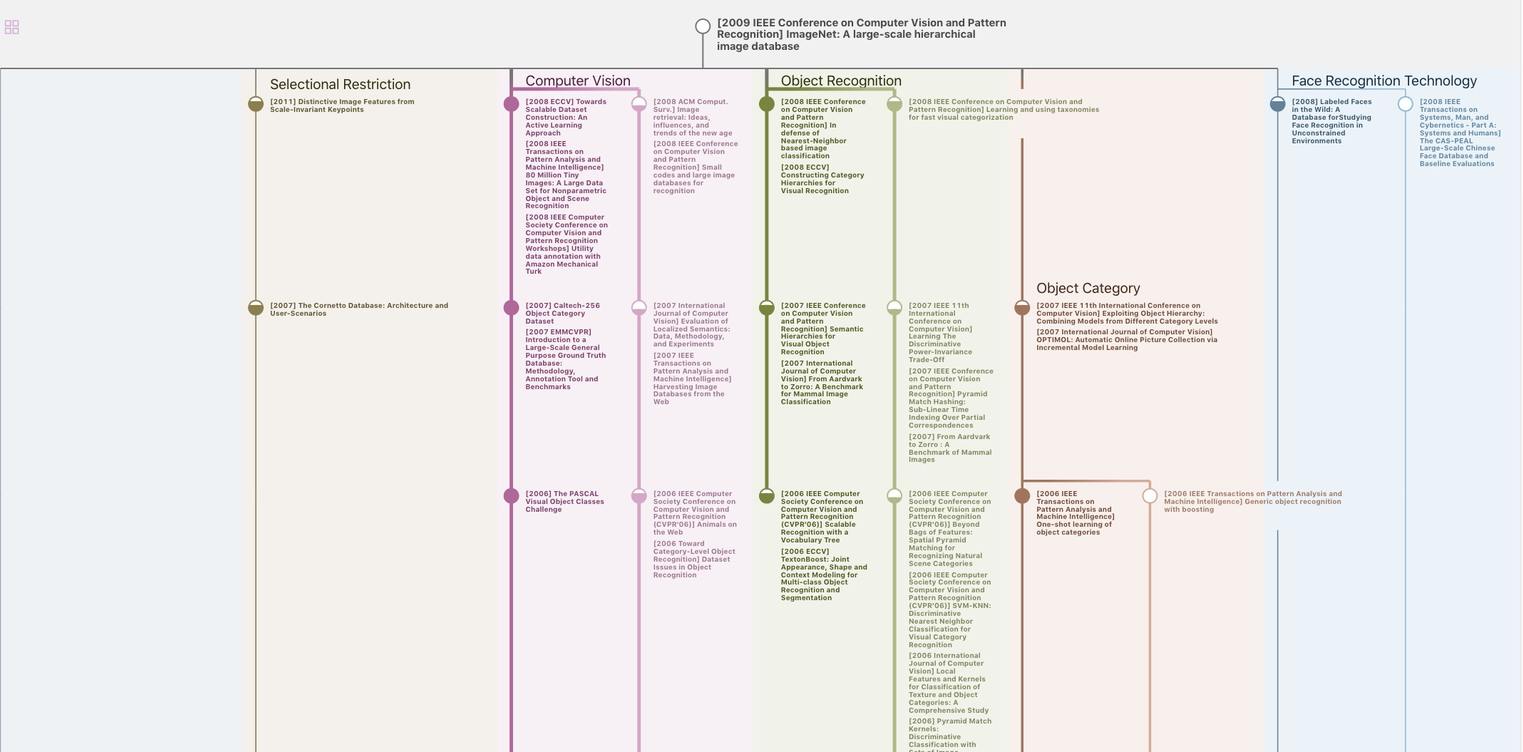
生成溯源树,研究论文发展脉络
Chat Paper
正在生成论文摘要