MepoGNN: Metapopulation Epidemic Forecasting with Graph Neural Networks
ECML/PKDD (6)(2023)
摘要
Epidemic prediction is a fundamental task for epidemic control and prevention. Many mechanistic models and deep learning models are built for this task. However, most mechanistic models have difficulty estimating the time/region-varying epidemiological parameters, while most deep learning models lack the guidance of epidemiological domain knowledge and interpretability of prediction results. In this study, we propose a novel hybrid model called MepoGNN for multi-step multi-region epidemic forecasting by incorporating Graph Neural Networks (GNNs) and graph learning mechanisms into Metapopulation SIR model. Our model can not only predict the number of confirmed cases but also explicitly learn the epidemiological parameters and the underlying epidemic propagation graph from heterogeneous data in an end-to-end manner. Experiment results demonstrate our model outperforms the existing mechanistic models and deep learning models by a large margin. Furthermore, the analysis on the learned parameters demonstrates the high reliability and interpretability of our model and helps better understanding of epidemic spread. Our model and data have already been public on GitHub
https://github.com/deepkashiwa20/MepoGNN.git
.
更多查看译文
关键词
metapopulation epidemic forecasting,graph,neural networks
AI 理解论文
溯源树
样例
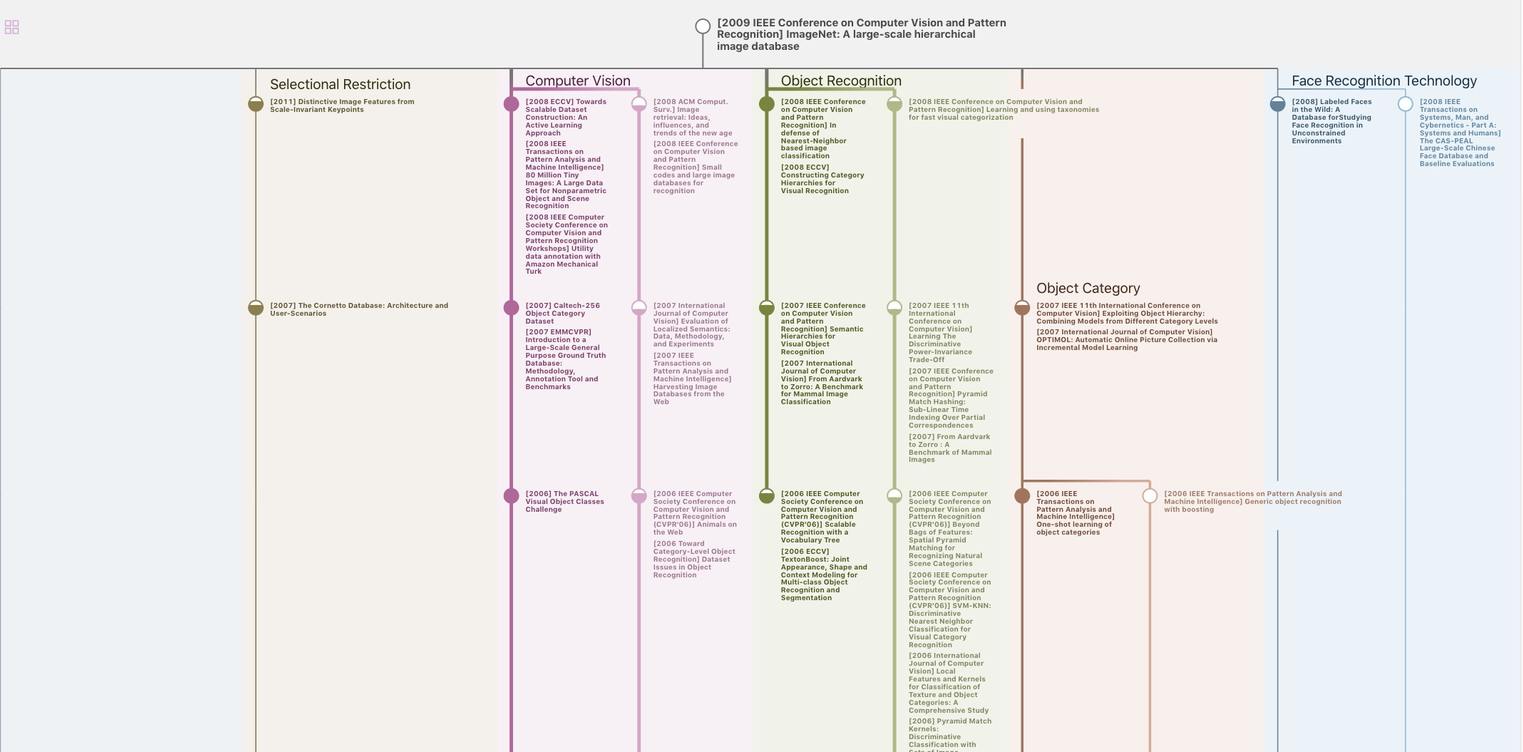
生成溯源树,研究论文发展脉络
Chat Paper
正在生成论文摘要