PathOracle: A Deep Learning Based Trip Planner for Daily Commuters
ECML/PKDD (6)(2023)
摘要
In this paper, we propose a novel data-driven approach for a trip planner, that finds the most popular multi-modal trip using public transport from historical trips, given a source, a destination, and userdefined constraints such as time, minimum switches, or preferred modes of transport. To solve the most popular trip and its variants, we propose a multi-stage deep learning architecture, PathOracle, that consists of two major components: KSNet to generate key stops, and MPTNet to generate popular path trips from a source to a destination passing through the key stops. We also introduce a unique representation of stops using Stop2Vec that considers both the neighborhood and trip popularity between stops to facilitate accurate path planning. We present an extensive experimental study with a large real-world public transport based commuting Myki dataset of Melbourne city, and demonstrate the effectiveness of our proposed approaches.
更多查看译文
关键词
planner,daily commuters,pathoracle,deep learning
AI 理解论文
溯源树
样例
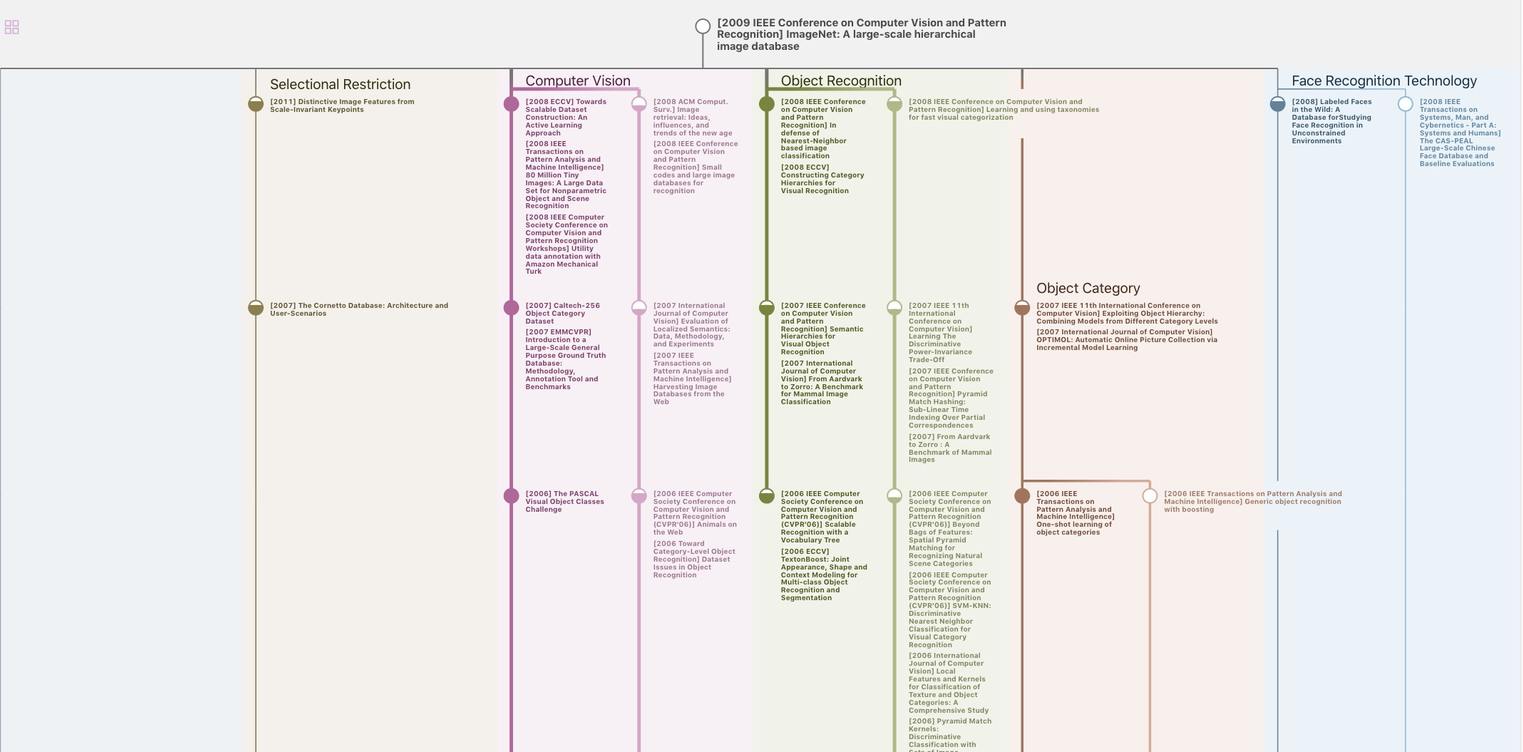
生成溯源树,研究论文发展脉络
Chat Paper
正在生成论文摘要