Linearized optimal transport on manifolds
arXiv (Cornell University)(2023)
摘要
Optimal transport is a geometrically intuitive, robust and flexible metric for sample comparison in data analysis and machine learning. Its formal Riemannian structure allows for a local linearization via a tangent space approximation. This in turn leads to a reduction of computational complexity and simplifies combination with other methods that require a linear structure. Recently this approach has been extended to the unbalanced Hellinger--Kantorovich (HK) distance. In this article we further extend the framework in various ways, including measures on manifolds, the spherical HK distance, a study of the consistency of discretization via the barycentric projection, and the continuity properties of the logarithmic map for the HK distance.
更多查看译文
关键词
optimal transport
AI 理解论文
溯源树
样例
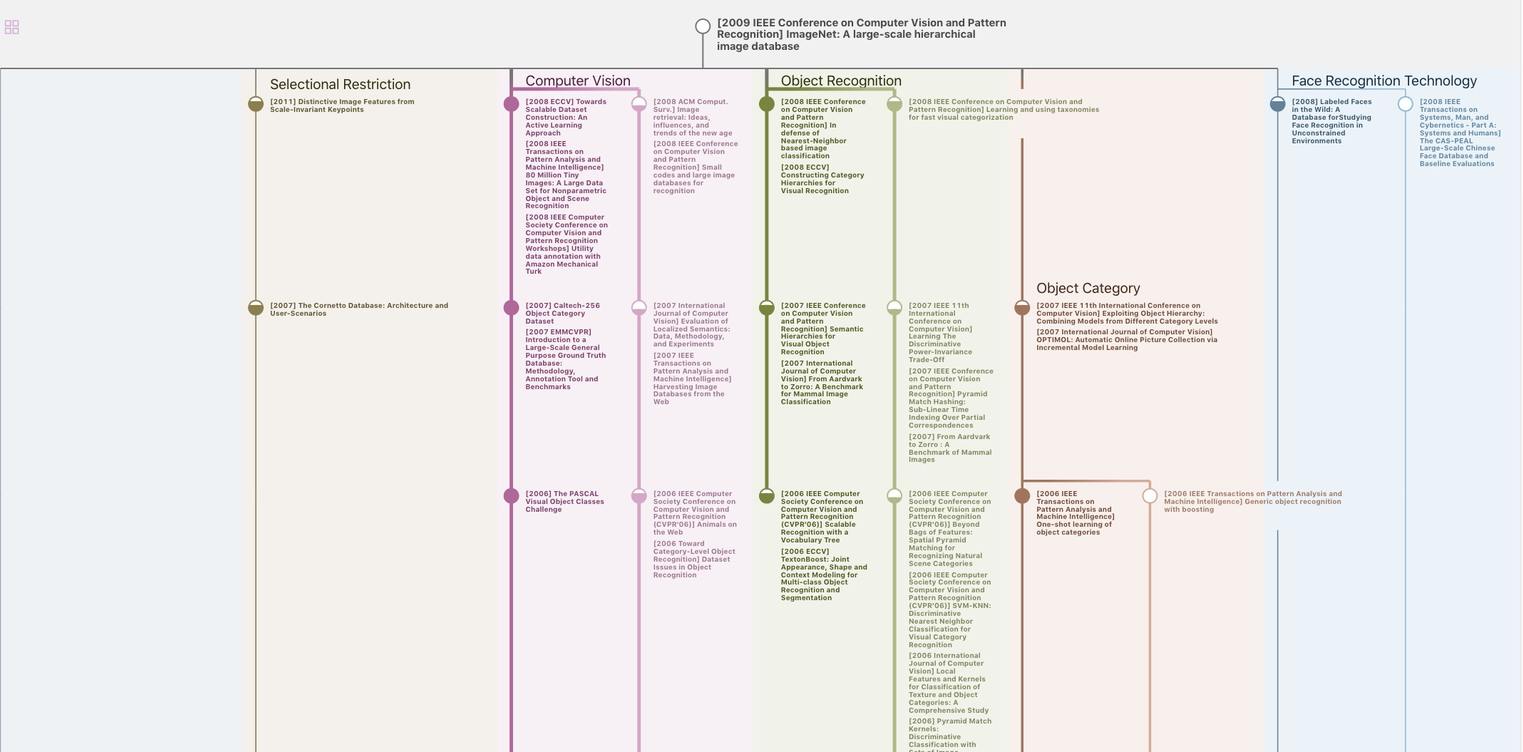
生成溯源树,研究论文发展脉络
Chat Paper
正在生成论文摘要