Communication-efficient Personalized Federated Edge Learning for Massive MIMO CSI Feedback
IEEE Transactions on Wireless Communications(2023)
摘要
Deep learning (DL)-based channel state information (CSI) feedback has received significant research attention in recent years. However, previous research has overlooked the potential privacy disclosure problem caused by the transmission of CSI datasets during the training process. In this work, we introduce a federated edge learning (FEEL)-based training framework for DL-based CSI feedback. This approach differs from the conventional centralized learning (CL)-based framework in which the CSI datasets are collected at the base station (BS) before training. Instead, each user equipment (UE) trains a local autoencoder network and exchanges model parameters with the BS. This approach provides better protection for data privacy compared to CL. To further reduce communication overhead in FEEL, we quantize uplink and downlink model transmission into different bits based on their influence on feedback performance. Additionally, since the heterogeneity of CSI datasets in different UEs can degrade the performance of the FEEL-based framework, we introduce a personalization strategy to improve feedback performance. This strategy allows for local fine-tuning to adapt the global model to the channel characteristics of each UE. Simulation results indicate that the proposed personalized FEEL-based training framework can significantly improve the performance of DL-based CSI feedback while reducing communication overhead.
更多查看译文
关键词
Massive MIMO,CSI feedback,federated edge learning,neural network quantization,personalization
AI 理解论文
溯源树
样例
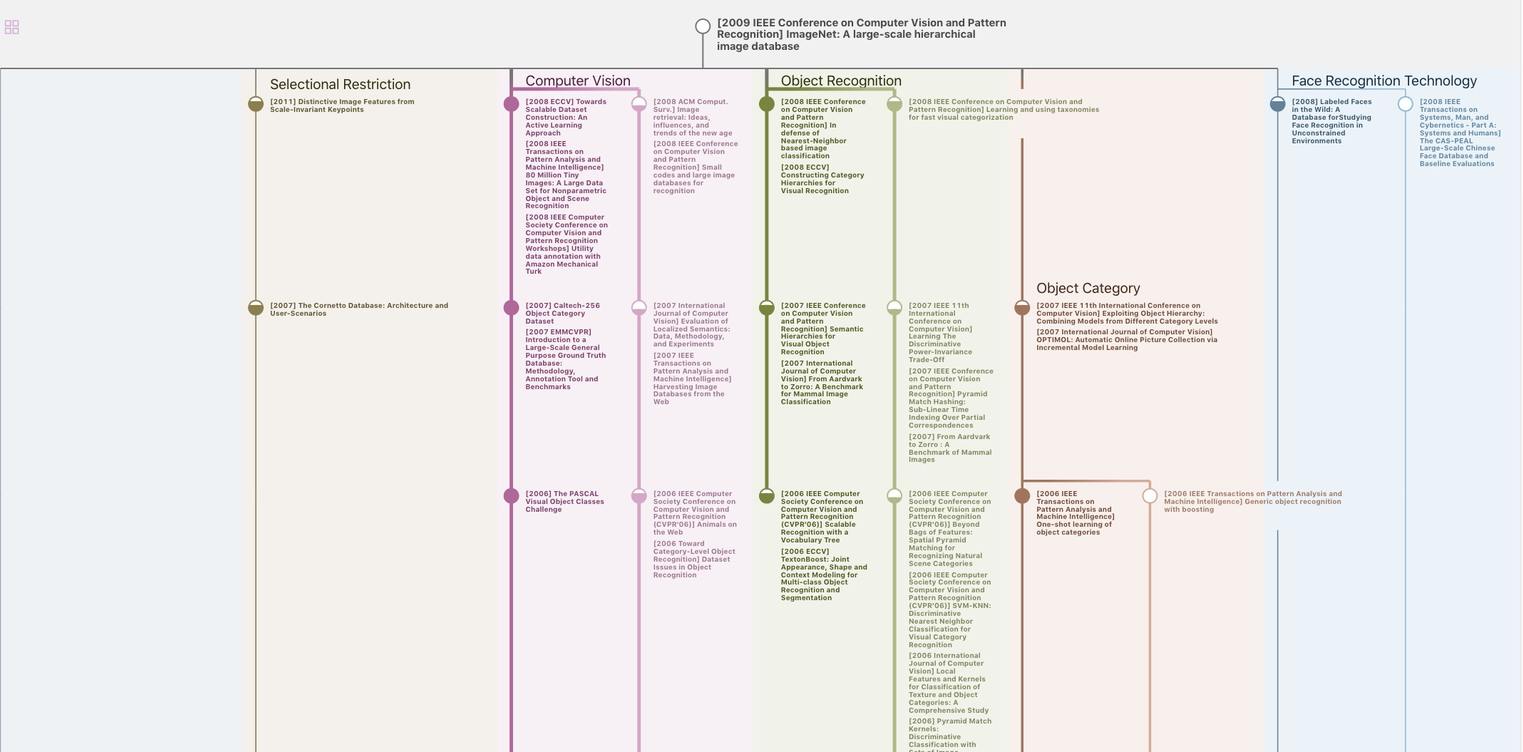
生成溯源树,研究论文发展脉络
Chat Paper
正在生成论文摘要