Clustering based on Mixtures of Sparse Gaussian Processes
CoRR(2023)
摘要
Creating low dimensional representations of a high dimensional data set is an important component in many machine learning applications. How to cluster data using their low dimensional embedded space is still a challenging problem in machine learning. In this article, we focus on proposing a joint formulation for both clustering and dimensionality reduction. When a probabilistic model is desired, one possible solution is to use the mixture models in which both cluster indicator and low dimensional space are learned. Our algorithm is based on a mixture of sparse Gaussian processes, which is called Sparse Gaussian Process Mixture Clustering (SGP-MIC). The main advantages to our approach over existing methods are that the probabilistic nature of this model provides more advantages over existing deterministic methods, it is straightforward to construct non-linear generalizations of the model, and applying a sparse model and an efficient variational EM approximation help to speed up the algorithm.
更多查看译文
关键词
sparse gaussian processes,gaussian processes,clustering,mixtures
AI 理解论文
溯源树
样例
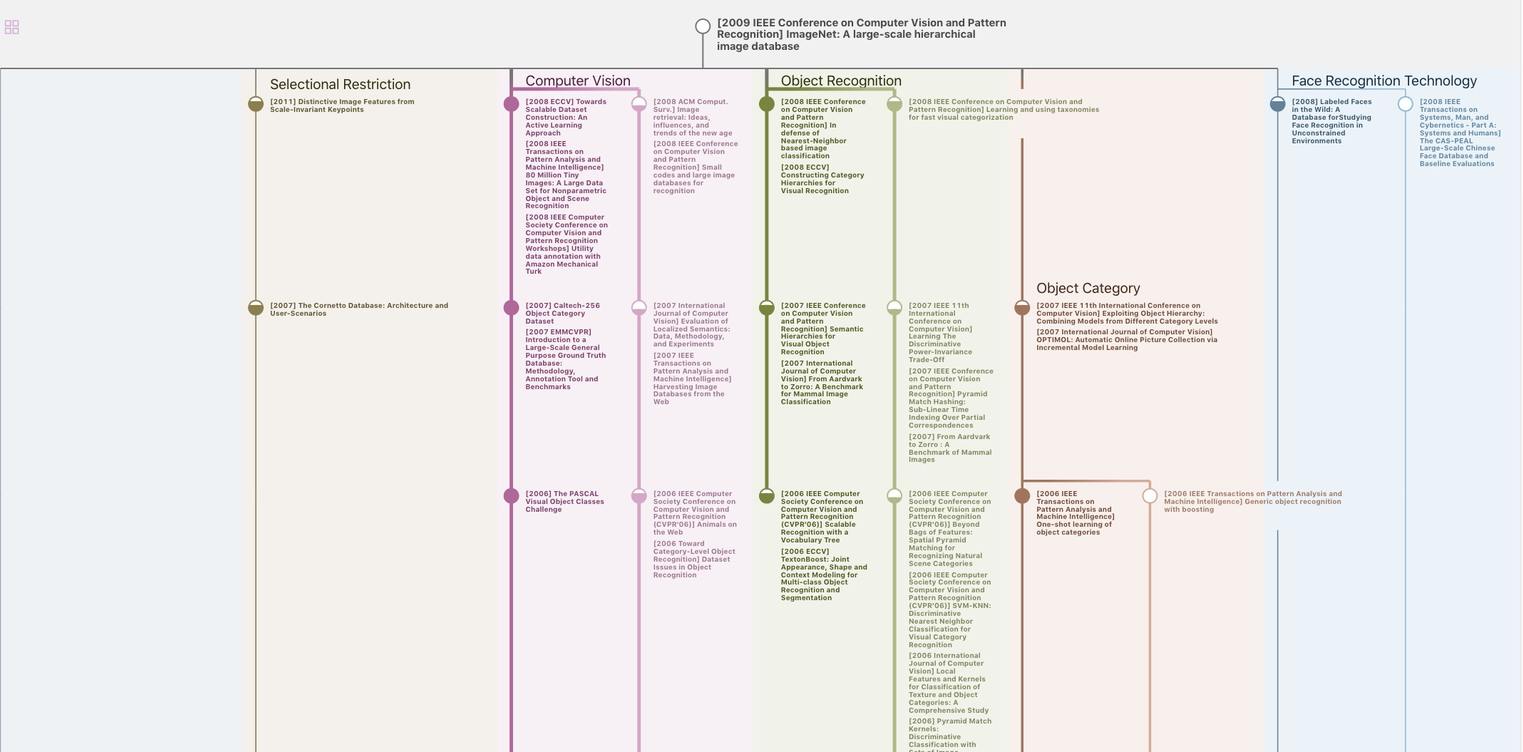
生成溯源树,研究论文发展脉络
Chat Paper
正在生成论文摘要