Adversarial Robustness and Feature Impact Analysis for Driver Drowsiness Detection
CoRR(2023)
摘要
Drowsy driving is a major cause of road accidents, but drivers are dismissive of the impact that fatigue can have on their reaction times. To detect drowsiness before any impairment occurs, a promising strategy is using Machine Learning (ML) to monitor Heart Rate Variability (HRV) signals. This work presents multiple experiments with different HRV time windows and ML models, a feature impact analysis using Shapley Additive Explanations (SHAP), and an adversarial robustness analysis to assess their reliability when processing faulty input data and perturbed HRV signals. The most reliable model was Extreme Gradient Boosting (XGB) and the optimal time window had between 120 and 150 seconds. Furthermore, SHAP enabled the selection of the 18 most impactful features and the training of new smaller models that achieved a performance as good as the initial ones. Despite the susceptibility of all models to adversarial attacks, adversarial training enabled them to preserve significantly higher results, especially XGB. Therefore, ML models can significantly benefit from realistic adversarial training to provide a more robust driver drowsiness detection.
更多查看译文
关键词
driver drowsiness detection,feature impact analysis
AI 理解论文
溯源树
样例
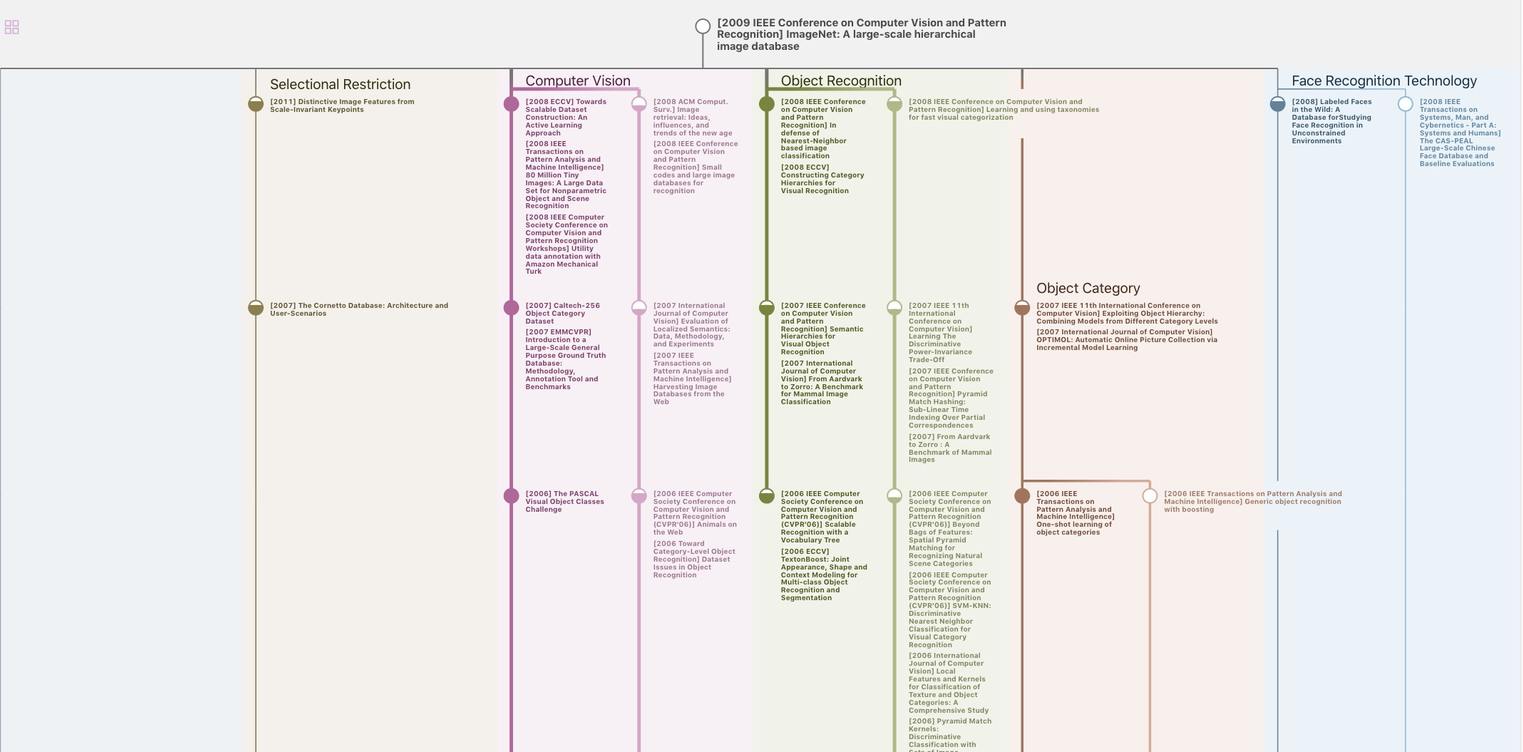
生成溯源树,研究论文发展脉络
Chat Paper
正在生成论文摘要