Clinically Relevant Latent Space Embedding of Cancer Histopathology Slides through Variational Autoencoder Based Image Compression
arxiv(2023)
摘要
In this paper, we introduce a Variational Autoencoder (VAE) based training approach that can compress and decompress cancer pathology slides at a compression ratio of 1:512, which is better than the previously reported state of the art (SOTA) in the literature, while still maintaining accuracy in clinical validation tasks. The compression approach was tested on more common computer vision datasets such as CIFAR10, and we explore which image characteristics enable this compression ratio on cancer imaging data but not generic images. We generate and visualize embeddings from the compressed latent space and demonstrate how they are useful for clinical interpretation of data, and how in the future such latent embeddings can be used to accelerate search of clinical imaging data.
更多查看译文
关键词
Histopathology cancer slides,autoencoder,image compression,latent space,clinical image search
AI 理解论文
溯源树
样例
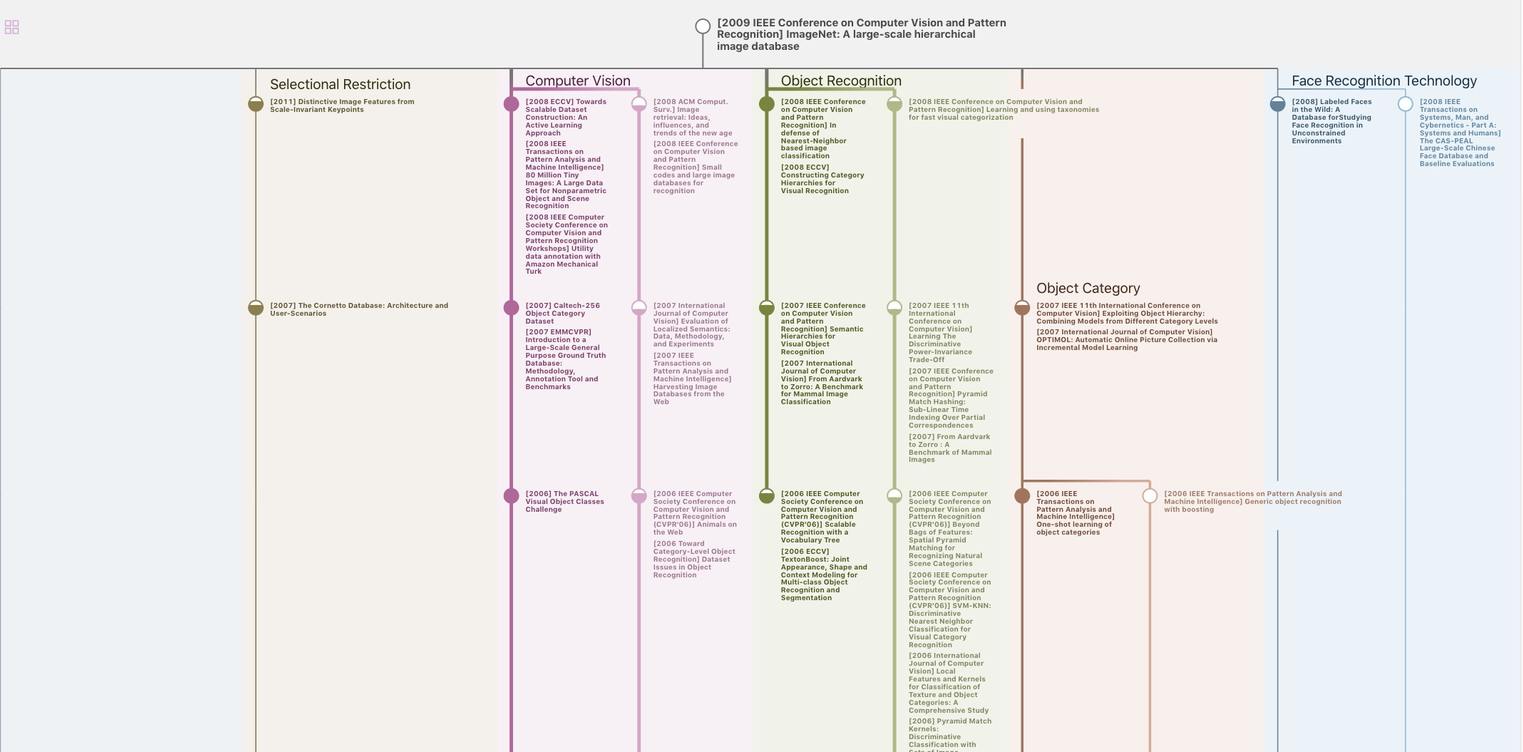
生成溯源树,研究论文发展脉络
Chat Paper
正在生成论文摘要