Functional-Coefficient Quantile Regression for Panel Data with Latent Group Structure
JOURNAL OF BUSINESS & ECONOMIC STATISTICS(2023)
摘要
This article considers estimating functional-coefficient models in panel quantile regression with individual effects, allowing the cross-sectional and temporal dependence for large panel observations. A latent group structure is imposed on the heterogeneous quantile regression models so that the number of nonparametric functional coefficients to be estimated can be reduced considerably. With the preliminary local linear quantile estimates of the subject-specific functional coefficients, a classic agglomerative clustering algorithm is used to estimate the unknown group structure and an easy-to-implement ratio criterion is proposed to determine the group number. The estimated group number and structure are shown to be consistent. Furthermore, a post-grouping local linear smoothing method is introduced to estimate the group-specific functional coefficients, and the relevant asymptotic normal distribution theory is derived with a normalization rate comparable to that in the literature. The developed methodologies and theory are verified through a simulation study and showcased with an application to house price data from U.K. local authority districts, which reveals different homogeneity structures at different quantile levels.
更多查看译文
关键词
Cluster analysis,Functional-coefficient models,Incidental parameter,Latent groups,Local linear estimation,Panel data,Quantile regression
AI 理解论文
溯源树
样例
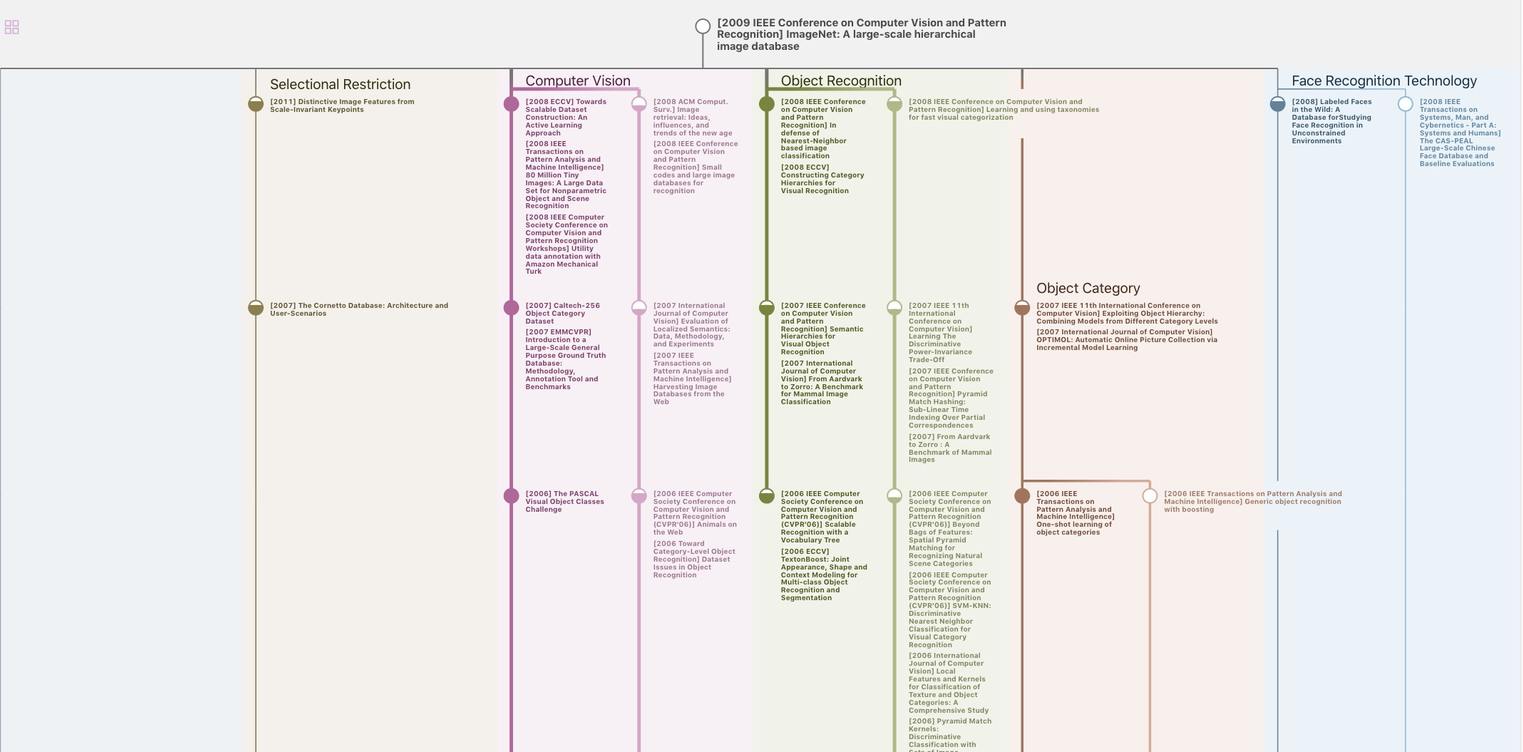
生成溯源树,研究论文发展脉络
Chat Paper
正在生成论文摘要