Adversarially Contrastive Estimation of Conditional Neural Processes
arxiv(2023)
摘要
Conditional Neural Processes~(CNPs) formulate distributions over functions and generate function observations with exact conditional likelihoods. CNPs, however, have limited expressivity for high-dimensional observations, since their predictive distribution is factorized into a product of unconstrained (typically) Gaussian outputs. Previously, this could be handled using latent variables or autoregressive likelihood, but at the expense of intractable training and quadratically increased complexity. Instead, we propose calibrating CNPs with an adversarial training scheme besides regular maximum likelihood estimates. Specifically, we train an energy-based model (EBM) with noise contrastive estimation, which enforces EBM to identify true observations from the generations of CNP. In this way, CNP must generate predictions closer to the ground-truth to fool EBM, instead of merely optimizing with respect to the fixed-form likelihood. From generative function reconstruction to downstream regression and classification tasks, we demonstrate that our method fits mainstream CNP members, showing effectiveness when unconstrained Gaussian likelihood is defined, requiring minimal computation overhead while preserving foundation properties of CNPs.
更多查看译文
关键词
contrastive estimation
AI 理解论文
溯源树
样例
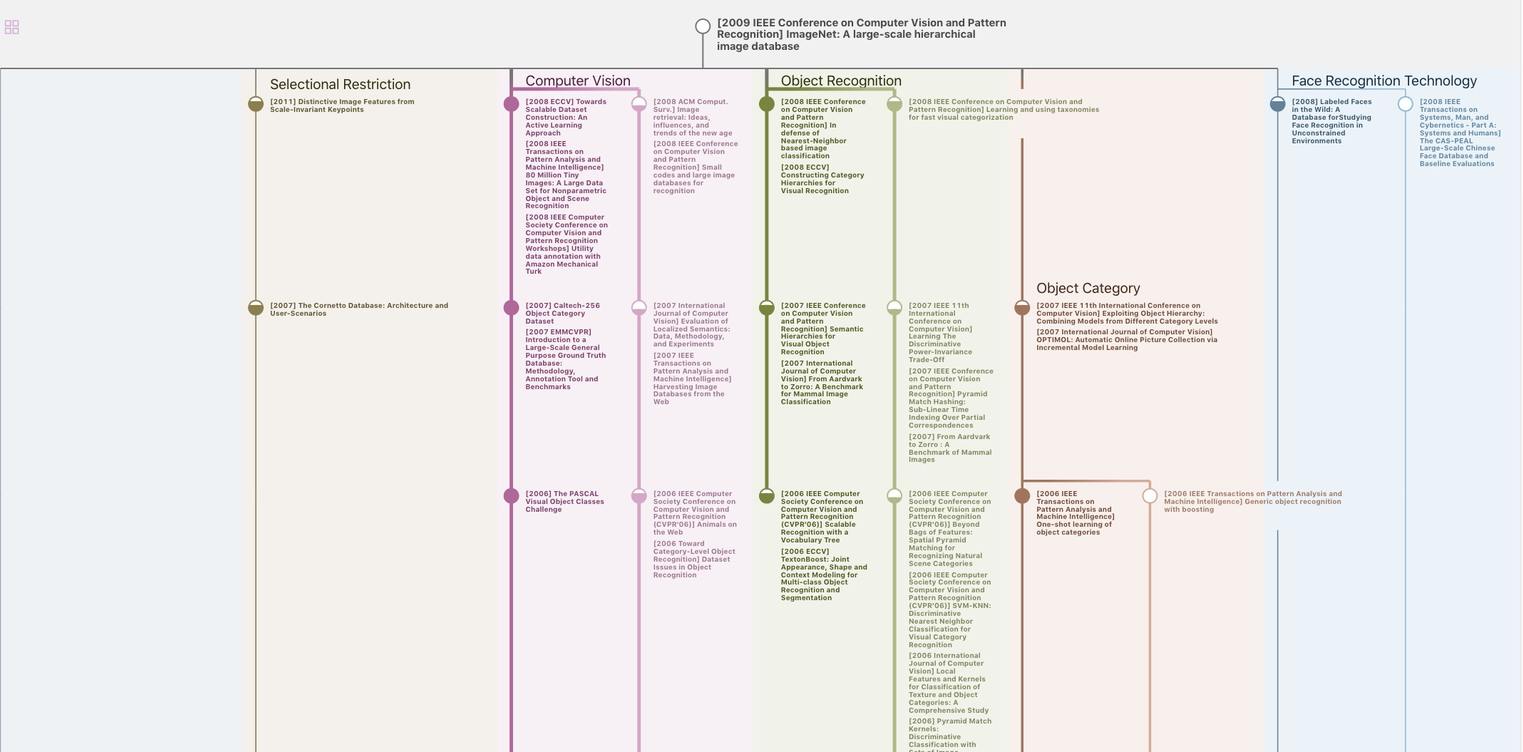
生成溯源树,研究论文发展脉络
Chat Paper
正在生成论文摘要