Active Learning Sensitivity Analysis of $\gamma^\prime$(L1$_2$) Precipitate Morphology of Ternary Co-Based Superalloys
arxiv(2023)
摘要
To better understand the equilibrium $\gamma^\prime$(L1$_2$) precipitate morphology in Co-based superalloys, a phase field modeling sensitivity analysis is conducted to examine how four phase-field parameters [initial Co concentration ($c_0$), double-well barrier height ($\omega$), gradient energy density coefficient ($\kappa$), and lattice misfit strain ($\epsilon_{\rm misfit}$)] influence the $\gamma^\prime$(L1$_2$) precipitate size and morphology. Gaussian Process Regression (GPR) models are used to fit the sample points and to generate surrogate models for both precipitate size and morphology. In an Active Learning approach, a Bayesian Optimization algorithm is coupled with the GPR models to suggest new sample points to calculate and efficiently update the models based on a reduction of uncertainty. The algorithm has a user-defined objective, which controls the balance between exploration and exploitation for new suggested points. Our methodology provides a qualitative and quantitative relationship between the $\gamma^\prime$(L1$_2$) precipitate size and morphology and the four phase-field parameters, and concludes that the most sensitive phase-field parameter for precipitate size and morphology is the initial Co concentration ($c_0$) and the double-well barrier height ($\omega$), respectively. We note that the GPR model for precipitate morphology required adding a noise tolerance in order to avoid overfitting due to irregularities in some of the simulated equilibrium $\gamma^\prime$(L1$_2$) precipitate morphology.
更多查看译文
关键词
superalloys,precipitate morphology,co-based
AI 理解论文
溯源树
样例
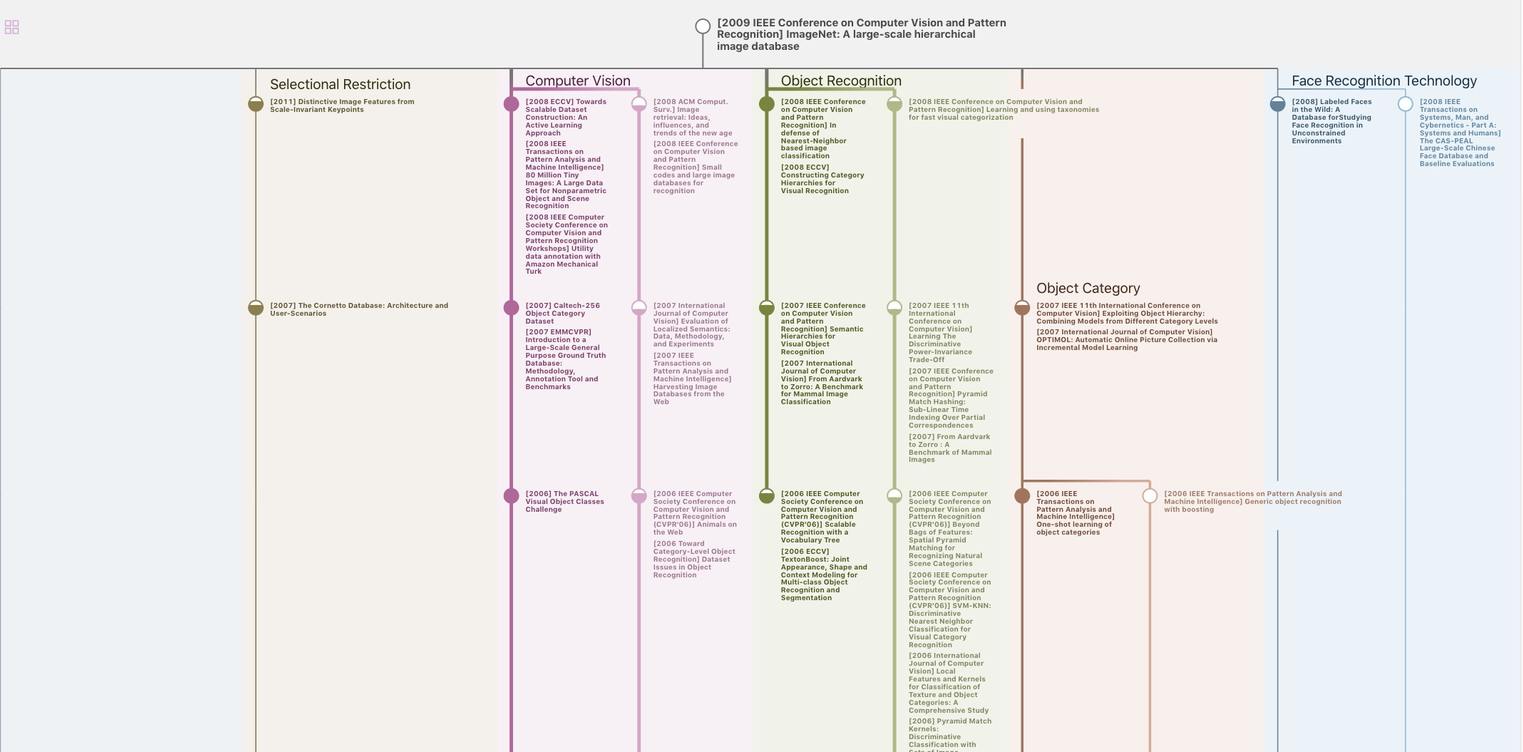
生成溯源树,研究论文发展脉络
Chat Paper
正在生成论文摘要