Sub-volume-based Denoising Diffusion Probabilistic Model for Cone-beam CT Reconstruction from Incomplete Data
arxiv(2023)
摘要
Deep learning (DL) has emerged as a new approach in the field of computed tomography (CT) with many applicaitons. A primary example is CT reconstruction from incomplete data, such as sparse-view image reconstruction. However, applying DL to sparse-view cone-beam CT (CBCT) remains challenging. Many models learn the mapping from sparse-view CT images to the ground truth but often fail to achieve satisfactory performance. Incorporating sinogram data and performing dual-domain reconstruction improve image quality with artifact suppression, but a straightforward 3D implementation requires storing an entire 3D sinogram in memory and many parameters of dual-domain networks. This remains a major challenge, limiting further research, development and applications. In this paper, we propose a sub-volume-based 3D denoising diffusion probabilistic model (DDPM) for CBCT image reconstruction from down-sampled data. Our DDPM network, trained on data cubes extracted from paired fully sampled sinograms and down-sampled sinograms, is employed to inpaint down-sampled sinograms. Our method divides the entire sinogram into overlapping cubes and processes them in parallel on multiple GPUs, successfully overcoming the memory limitation. Experimental results demonstrate that our approach effectively suppresses few-view artifacts while preserving textural details faithfully.
更多查看译文
关键词
ct reconstruction,denoising diffusion probabilistic model,sub-volume-based,cone-beam
AI 理解论文
溯源树
样例
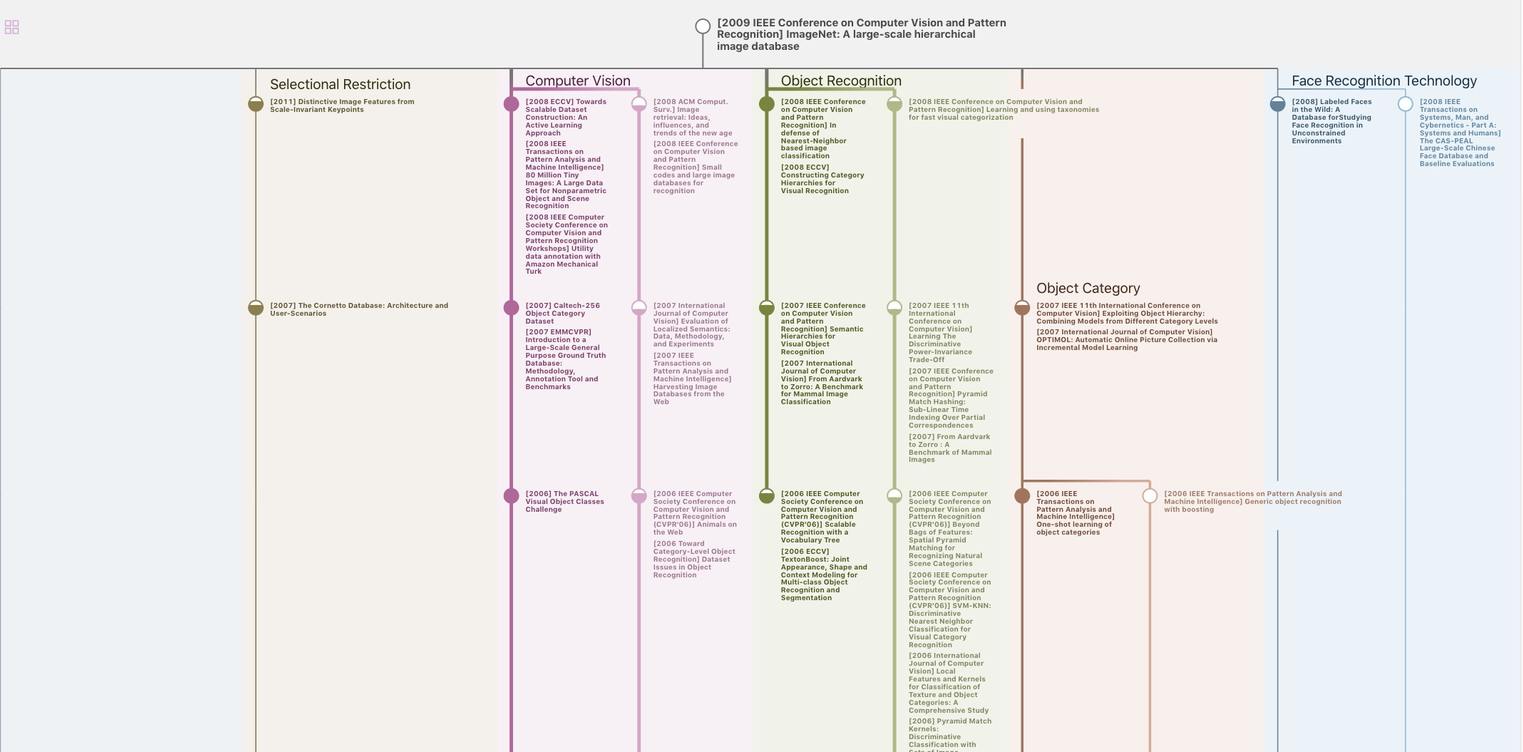
生成溯源树,研究论文发展脉络
Chat Paper
正在生成论文摘要