Time Series as Images: Vision Transformer for Irregularly Sampled Time Series
NeurIPS(2023)
摘要
Irregularly sampled time series are becoming increasingly prevalent in various domains, especially in medical applications. Although different highly-customized methods have been proposed to tackle irregularity, how to effectively model their complicated dynamics and high sparsity is still an open problem. This paper studies the problem from a whole new perspective: transforming irregularly sampled time series into line graph images and adapting powerful vision transformers to perform time series classification in the same way as image classification. Our approach largely simplifies algorithm designs without assuming prior knowledge and can be potentially extended as a general-purpose framework. Despite its simplicity, we show that it substantially outperforms state-of-the-art specialized algorithms on several popular healthcare and human activity datasets. Especially in the challenging leave-sensors-out setting where a subset of variables is masked during testing, the performance improvement is up to 54.0\% in absolute F1 score points. Our code and data are available at \url{https://github.com/Leezekun/ViTST}.
更多查看译文
AI 理解论文
溯源树
样例
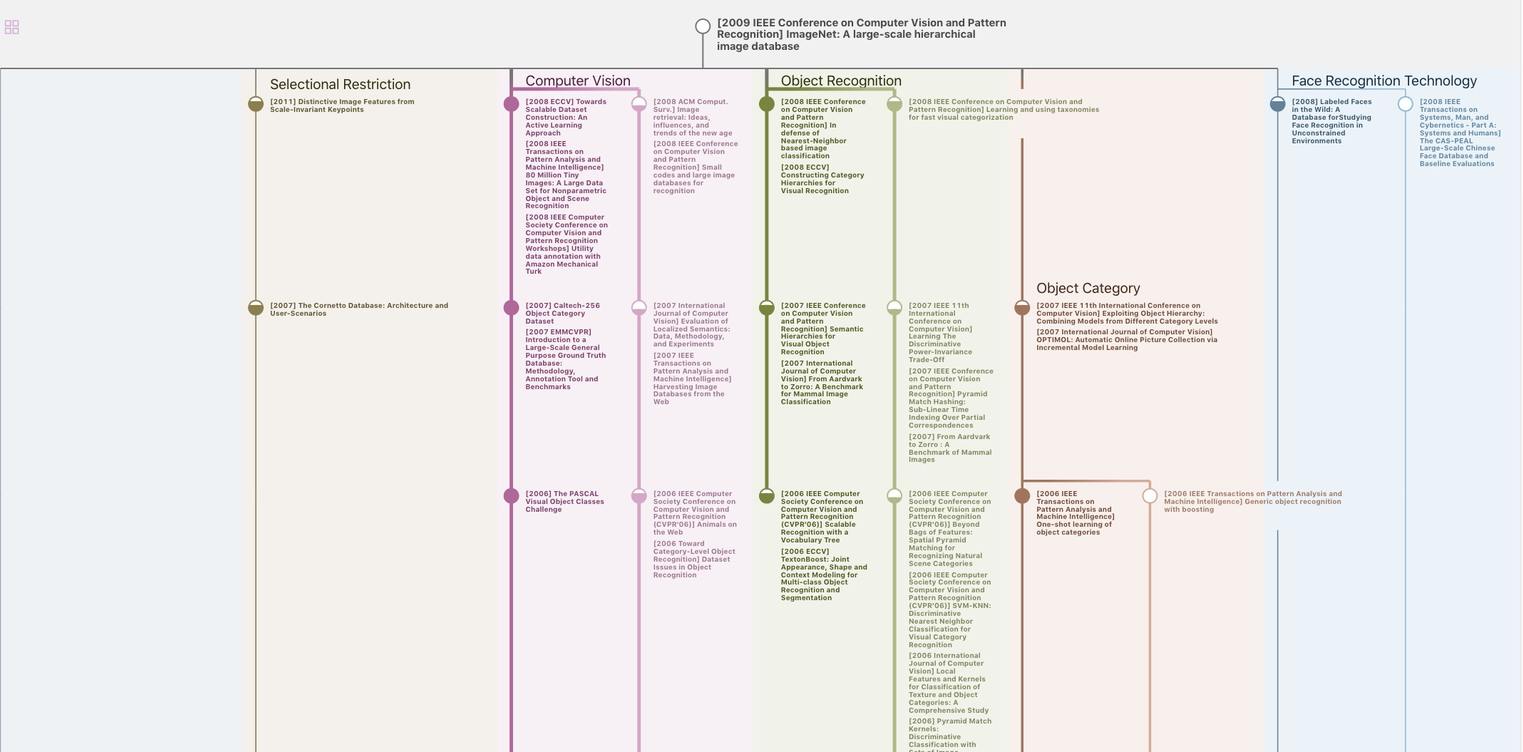
生成溯源树,研究论文发展脉络
Chat Paper
正在生成论文摘要