Ensemble models for circuit topology estimation, fault detection and classification in distribution systems
Sustainable Energy, Grids and Networks(2023)
摘要
This paper presents a methodology for simultaneous fault detection, classification, and topology estimation for adaptive protection of distribution systems. The methodology estimates the probability of the occurrence of each one of these events by using a hybrid structure that combines three sub-systems, a convolutional neural network for topology estimation, a fault detection based on predictive residual analysis, and a standard support vector machine with probabilistic output for fault classification. The input to all these sub-systems is the local voltage and current measurements. A convolutional neural network uses these local measurements in the form of sequential data to extract features and estimate the topology conditions. The fault detector is constructed with a Bayesian stage (a multitask Gaussian process) that computes a predictive distribution (assumed to be Gaussian) of the residuals using the input. Since the distribution is known, these residuals can be transformed into a Standard distribution, whose values are then introduced into a one-class support vector machine. The structure allows using a one-class support vector machine without parameter cross-validation, so the fault detector is fully unsupervised. Finally, a support vector machine uses the input to perform the classification of the fault types. All three sub-systems can work in a parallel setup for both performance and computation efficiency. We test all three sub-systems included in the structure on a modified IEEE123 bus system, and we compare and evaluate the results with standard approaches.
更多查看译文
关键词
Distribution systems,Adaptive protection,Topology estimation,Fault detection,Convolutional neural networks,Support vector machines
AI 理解论文
溯源树
样例
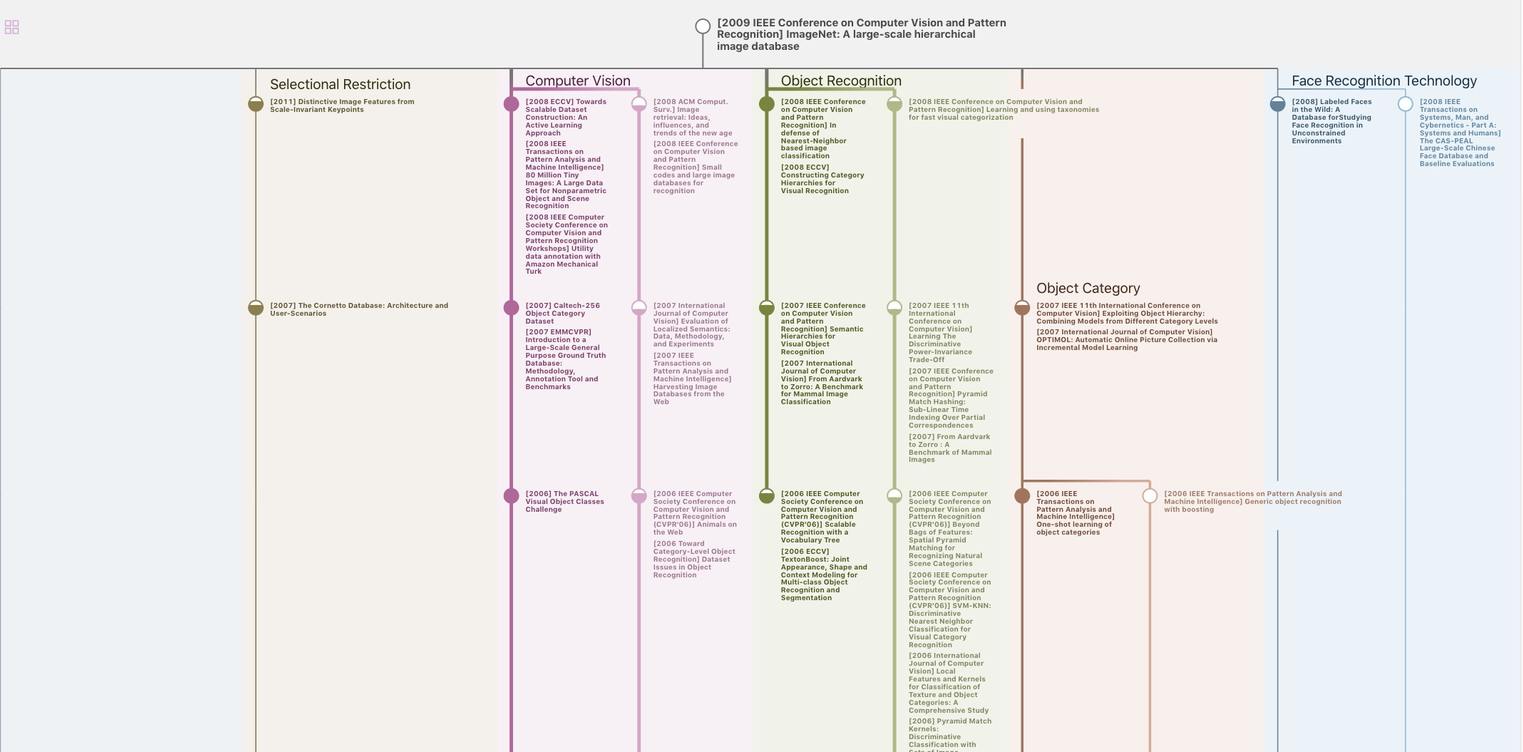
生成溯源树,研究论文发展脉络
Chat Paper
正在生成论文摘要