P3O: Transferring Visual Representations for Reinforcement Learning via Prompting
arxiv(2023)
摘要
It is important for deep reinforcement learning (DRL) algorithms to transfer their learned policies to new environments that have different visual inputs. In this paper, we introduce Prompt based Proximal Policy Optimization ((PO)-O-3), a three-stage DRL algorithm that transfers visual representations from a target to a source environment by applying prompting. The process of (PO)-O-3 consists of three stages: pre-training, prompting, and predicting. In particular, we specify a prompt-transformer for representation conversion and propose a two-step training process to train the prompt-transformer for the target environment, while the rest of the DRL pipeline remains unchanged. We implement (PO)-O-3 and evaluate it on the OpenAI CarRacing video game. The experimental results show that (PO)-O-3 outperforms the state-of-the-art visual transferring schemes. In particular, (PO)-O-3 allows the learned policies to perform well in environments with different visual inputs, which is much more effective than retraining the policies in these environments.
更多查看译文
关键词
reinforcement learning
AI 理解论文
溯源树
样例
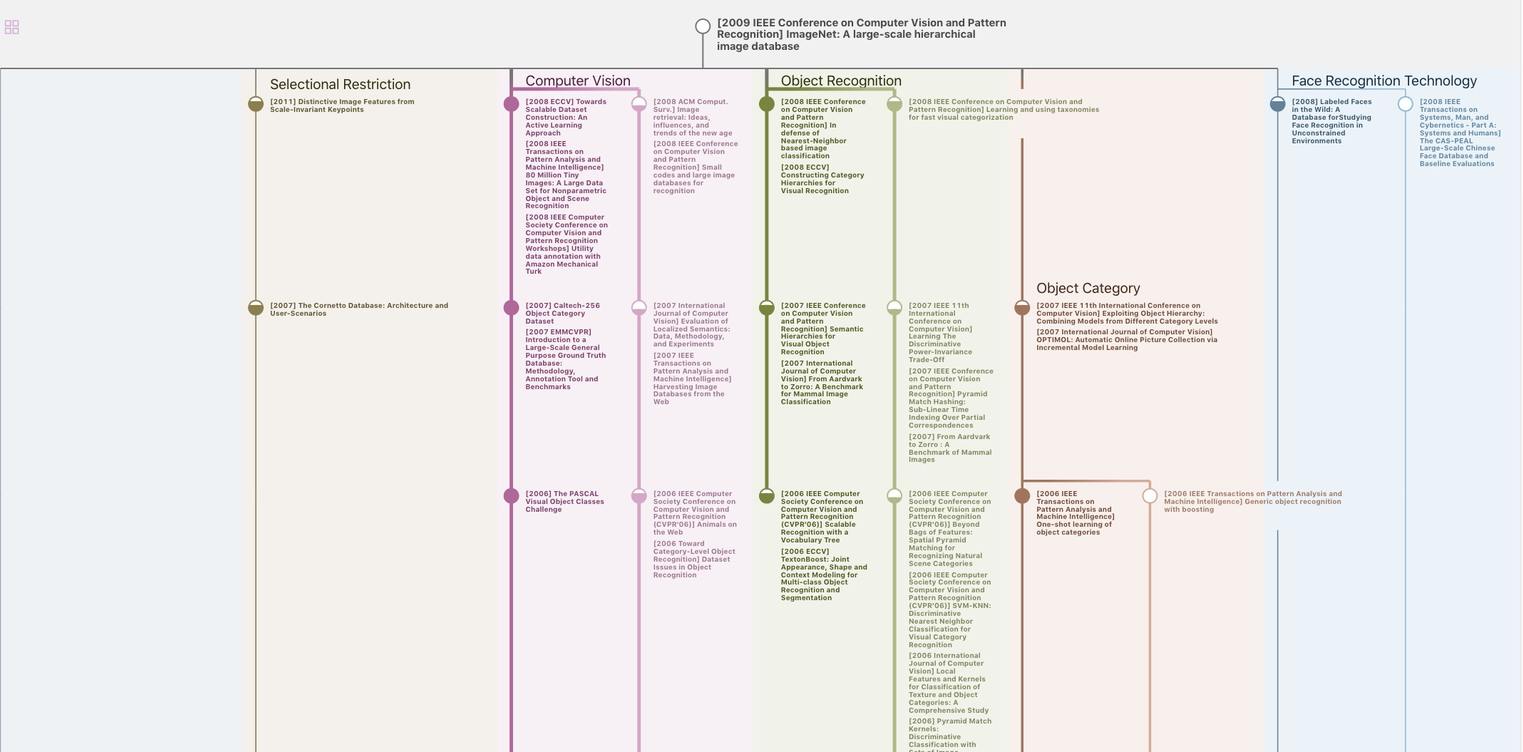
生成溯源树,研究论文发展脉络
Chat Paper
正在生成论文摘要