LD-ZNet: A Latent Diffusion Approach for Text-Based Image Segmentation
arxiv(2023)
摘要
We present a technique for segmenting real and AI-generated images using latent diffusion models (LDMs) trained on internet-scale datasets. First, we show that the latent space of LDMs (z-space) is a better input representation compared to other feature representations like RGB images or CLIP encodings for text-based image segmentation. By training the segmentation models on the latent z-space, which creates a compressed representation across several domains like different forms of art, cartoons, illustrations, and photographs, we are also able to bridge the domain gap between real and AI-generated images. We show that the internal features of LDMs contain rich semantic information and present a technique in the form of LD-ZNet to further boost the performance of text-based segmentation. Overall, we show up to 6% improvement over standard baselines for text-to-image segmentation on natural images. For AI-generated imagery, we show close to 20% improvement compared to state-of-the-art techniques.
更多查看译文
关键词
image segmentation,latent diffusion approach,ld-znet,text-based
AI 理解论文
溯源树
样例
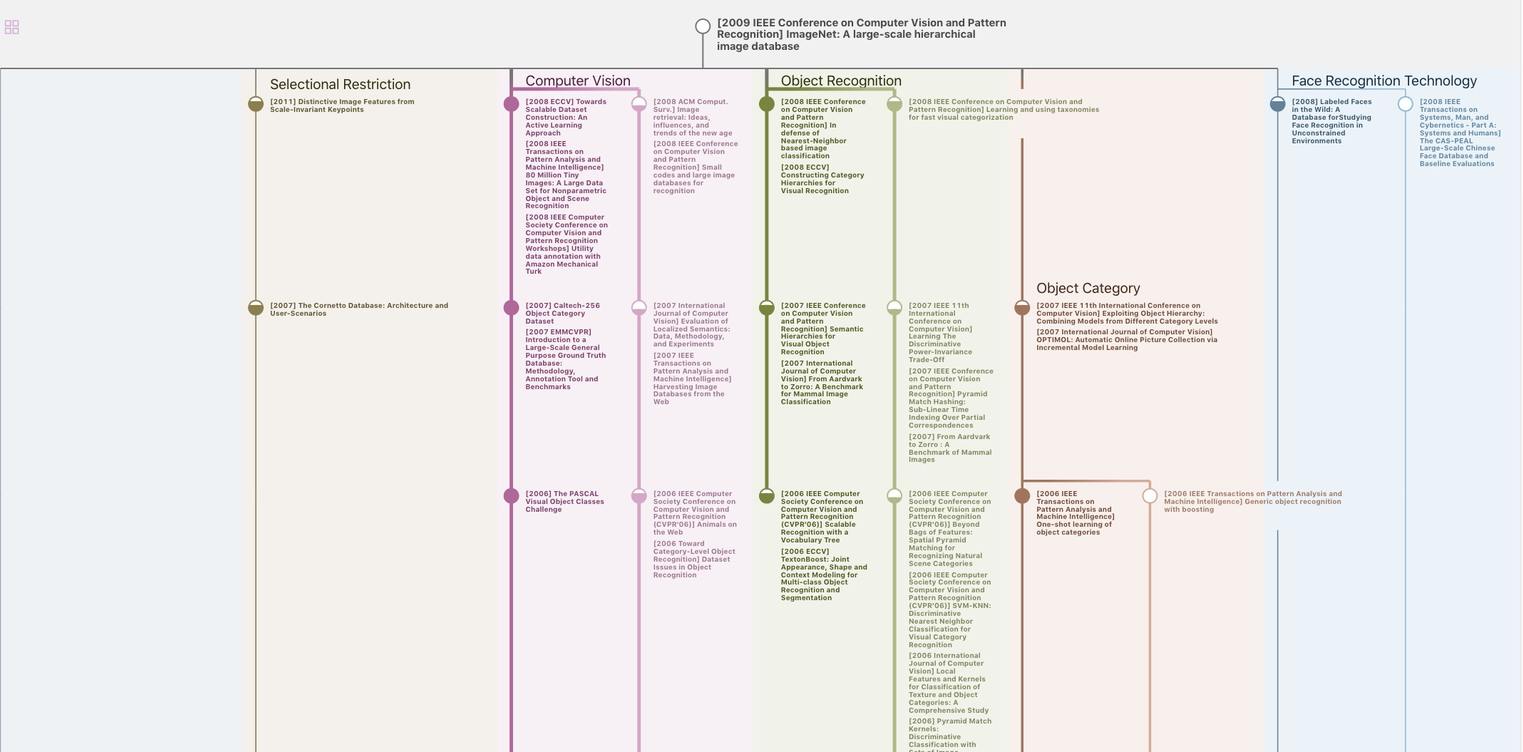
生成溯源树,研究论文发展脉络
Chat Paper
正在生成论文摘要