Challenges and opportunities for machine learning in multiscale computational modeling
arxiv(2023)
摘要
Many mechanical engineering applications call for multiscale computational modeling and simulation. However, solving for complex multiscale systems remains computationally onerous due to the high dimensionality of the solution space. Recently, machine learning (ML) has emerged as a promising solution that can either serve as a surrogate for, accelerate or augment traditional numerical methods. Pioneering work has demonstrated that ML provides solutions to governing systems of equations with comparable accuracy to those obtained using direct numerical methods, but with significantly faster computational speed. These high-speed, high-fidelity estimations can facilitate the solving of complex multiscale systems by providing a better initial solution to traditional solvers. This paper provides a perspective on the opportunities and challenges of using ML for complex multiscale modeling and simulation. We first outline the current state-of-the-art ML approaches for simulating multiscale systems and highlight some of the landmark developments. Next, we discuss current challenges for ML in multiscale computational modeling, such as the data and discretization dependence, interpretability, and data sharing and collaborative platform development. Finally, we suggest several potential research directions for the future.
更多查看译文
关键词
machine learning,computational,modeling
AI 理解论文
溯源树
样例
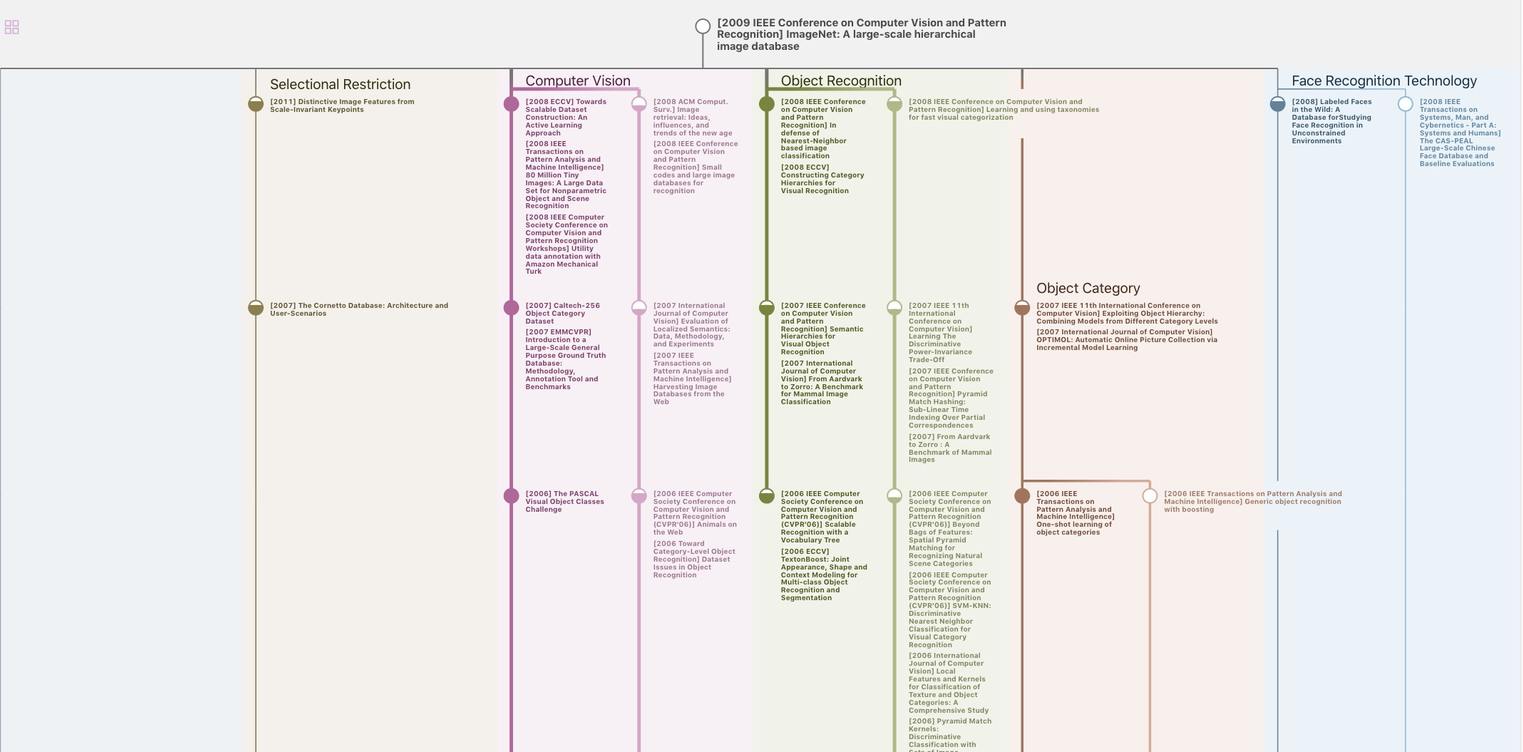
生成溯源树,研究论文发展脉络
Chat Paper
正在生成论文摘要