MCT-Net: Multi-hierarchical cross transformer for hyperspectral and multispectral image fusion
Knowledge-Based Systems(2023)
摘要
Taking into account the limitations of optical imaging, image acquisition equipment is usually designed to make a trade-off between spatial information and spectral information. Hyperspectral image(HSI) can finely identify and classify imaging objects owing to its rich spectral information, while multispectral image(MSI) can provide fine geometric features because of its sufficient spatial information. Hence, fusing HSI and MSI to achieve information complementarity has become a prevalent manner, which increases the reliability and accuracy of the information obtained. However, unlike traditional optical multi-focus image fusion and pan-sharpening of MSI, existing HSI and MSI fusion methods still face with problems in achieving cross-modality information interaction and lack effective utilization of spatial location information. To solve the above problems and to achieve more effective information integration between HSI and MSI, this paper proposes a novel multi-hierarchical cross transformer for hyperspectral and multispectral image fusion (MCT-Net). The proposed MCT-Net consists of two components: (1) a multi-hierarchical cross-modality interacting module (MCIM), which first extracts the deep multi-scale features of HSI and MSI, and then performs cross-modality information interaction between them at identical scales by applying a multi-hierarchical cross transformer (MCT), to reconstruct the spectral information lacking in MSI and the spatial information lacking in HSI; (2) a feature aggregation reconstruction module (FARM) which combines features from MCIM, uses strip convolution to further restore edge features, and reconstructs the fusion results through cascaded upsampling. We conduct comparative experiments on five mainstream HSI datasets to prove the effectiveness and superiority of the proposed method, including the Pavia Center, Pavia University, Urban, Botswana, and Washington DC Mall. For instance, on the Washington DC Mall dataset, compared with the state-of-the-art(SOTA) method in the comparison algorithms, our method improves PSNR by 18.52% and reduces RMSE, ERGAS and SAM by 56.63%, 56.90% and 58.58%, respectively. The source code for MCT-Net can be downloaded from https://github.com/wxy11-27/MCT-Net.
更多查看译文
关键词
Transformer,Hyperspectral,Multispectral,Image fusion,Deep multi-scale features
AI 理解论文
溯源树
样例
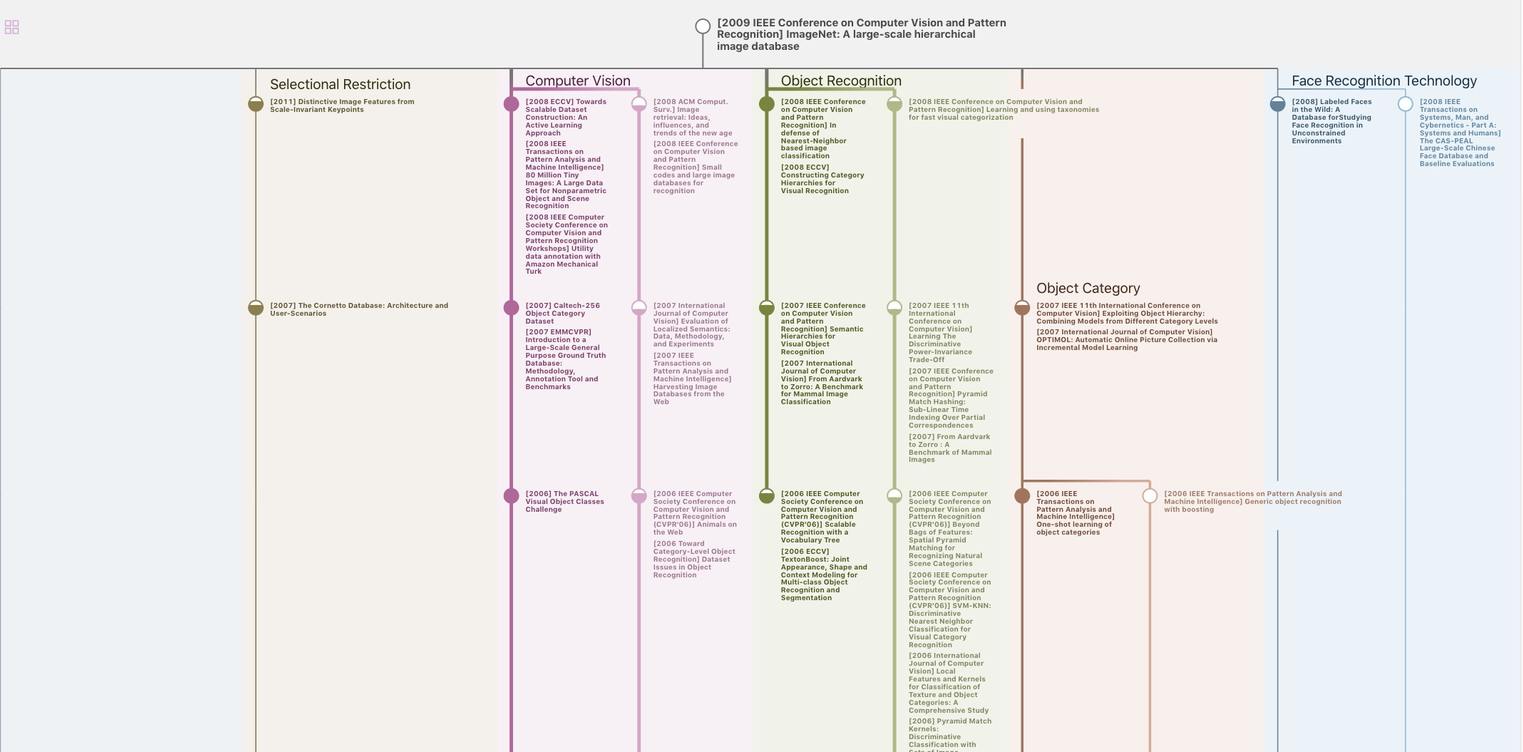
生成溯源树,研究论文发展脉络
Chat Paper
正在生成论文摘要