TaxonPrompt: Taxonomy-aware curriculum prompt learning for few-shot event classification
Knowledge-Based Systems(2023)
摘要
Event classification (EC) aims to assign the event labels to unlabeled sentences and tends to struggle in real-world applications when only a few annotated samples are available. Previous studies have mainly focused on using meta-learning to overcome the low-resource problem where label data from other tasks are still required for model learning and selection. Accordingly, prompt learning-based approaches are proposed to address the low-resource issue. However, such approaches generally ignore task-specific information and adopt demonstration learning for fine-tuning, which fails to leverage the most informative examples for training and hurts performance. Thus, we propose a taxonomy-aware prompt learning framework TaxonPrompt that trains the language model with samples from easy to hard by imitating the human curricula, which effectively alleviates the classification bottleneck caused by insufficient data. We first design an event prompt generation (EPG) for automatically generating task-specific templates using sentences, labels, and trigger words. Then, we propose a Fisher information-based demonstration filtering (FDF) to dynamically select the most informative support examples for each query to train the model. We have conducted extensive experiments on two EC datasets: FewEvent and RAMS. The experimental results demonstrate the superiority of the proposed model over state-of-the-art baselines. In particular, our approach works well in the scenario of an extremely small number of available task resources and therefore constitutes a solution for few-shot event classification.
更多查看译文
关键词
Event classification,Few-shot learning,Prompt tuning,Pre-trained language model,Template generation,Information extraction
AI 理解论文
溯源树
样例
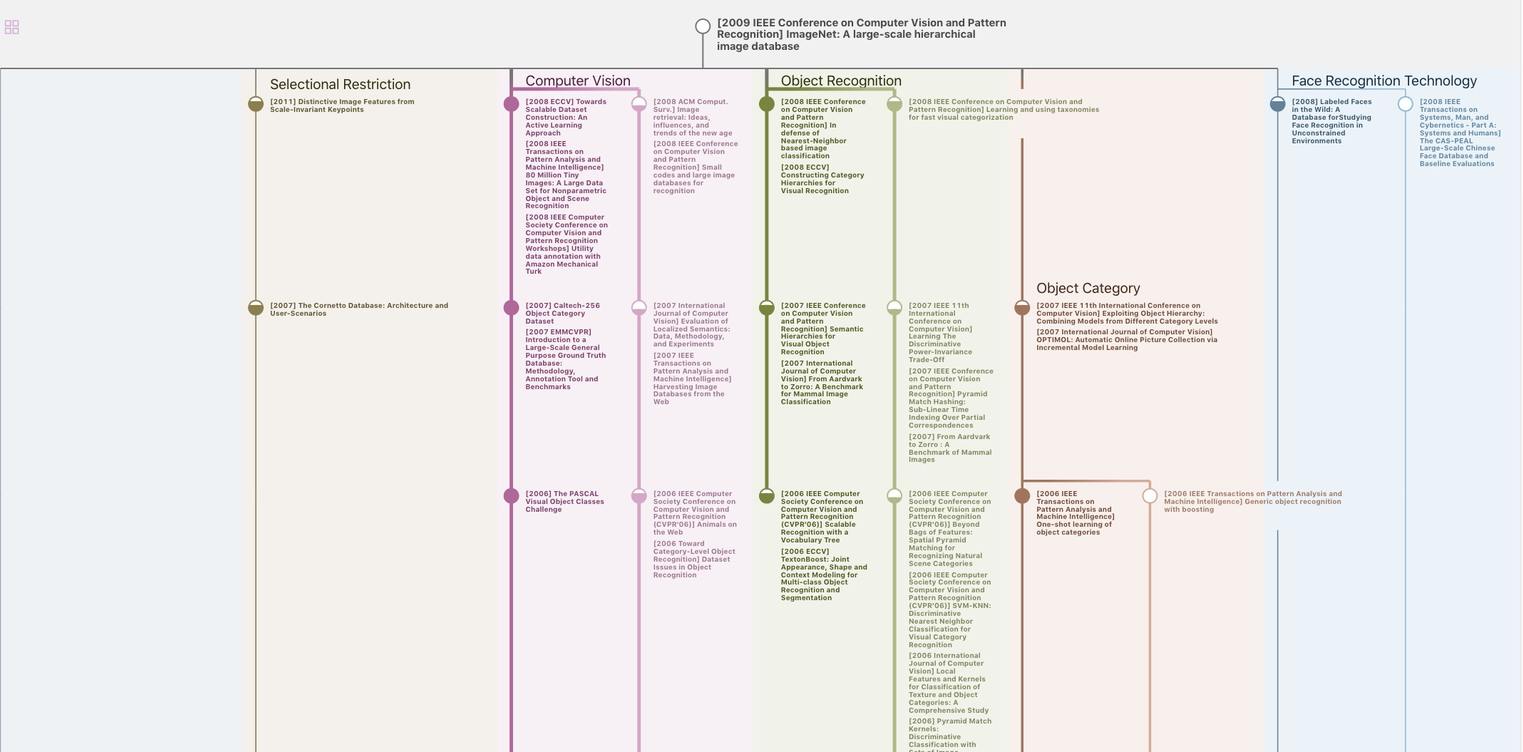
生成溯源树,研究论文发展脉络
Chat Paper
正在生成论文摘要