An improved arithmetic optimization algorithm for training feedforward neural networks under dynamic environments
Knowledge-Based Systems(2023)
摘要
This paper proposes an improved Arithmetic Optimization Algorithm (AOA) to train artificial neural networks (ANNs) under dynamic environments. Despite many successful applications of metaheuristic training of ANNs, these studies assume static environments, which might not be realistic in real-world nonstationary processes. In this study, the training of ANNs is modeled as a dynamic optimization problem, and the proposed AOA is used to optimize connection weights and biases of the ANN under the presence of concept drift. The proposed method is designed to work for classification tasks. The performance of the proposed algorithm has been tested on twelve dynamic classification problems. Comparative analysis with state-of-the-art metaheuristic optimization algorithms has been provided. The superiority of the compared algorithms has been verified using nonparametric statistical tests. The results show that the improved AOA outperforms compared algorithms in training ANNs under dynamic environments. The findings demonstrate the potential of improved AOA for dynamic data-driven applications.
更多查看译文
关键词
Arithmetic optimization algorithm,Artificial neural networks,Concept drift,Dynamic optimization
AI 理解论文
溯源树
样例
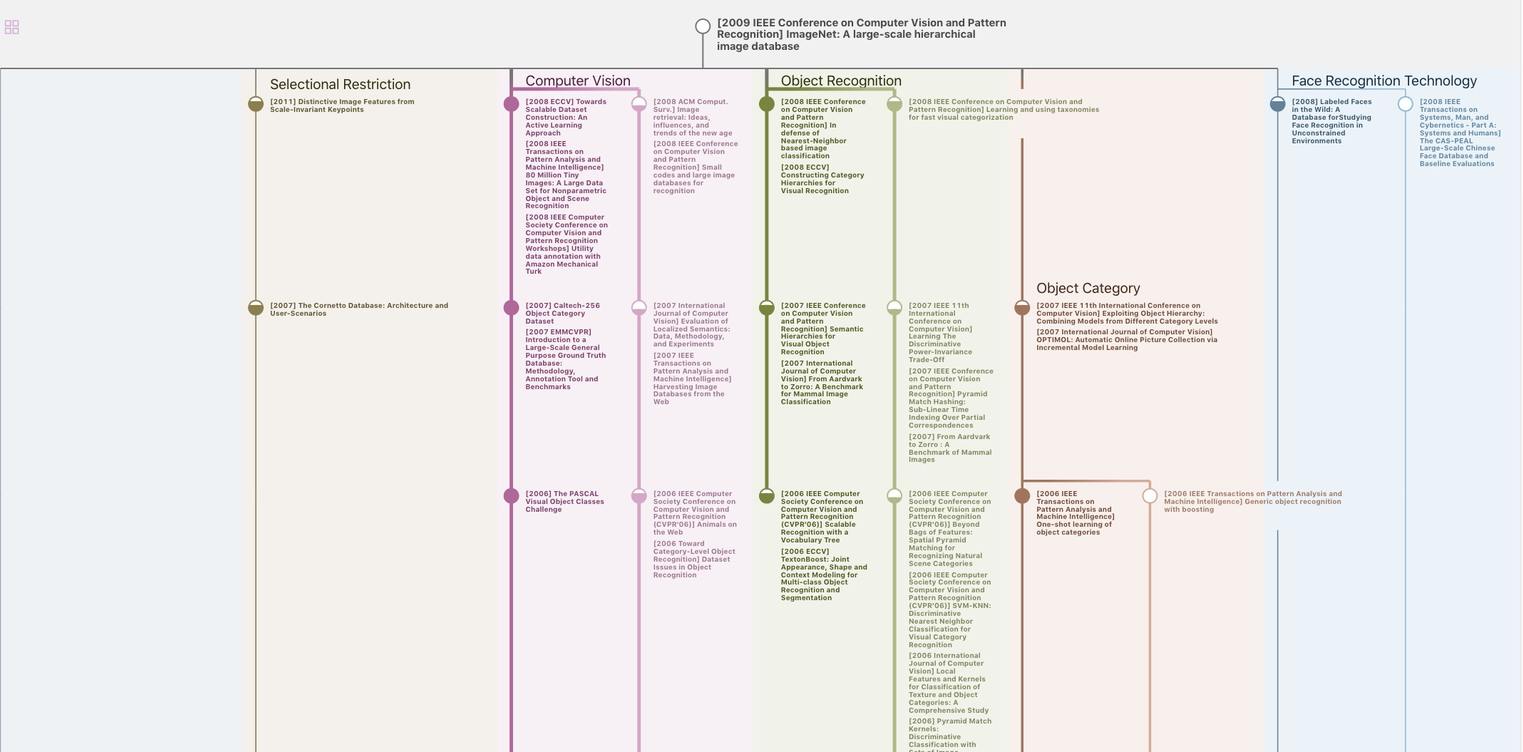
生成溯源树,研究论文发展脉络
Chat Paper
正在生成论文摘要