Random forest-based surrogates for transforming the behavioral predictions of laminated composite plates and shells from FSDT to Elasticity solutions
Composite Structures(2023)
摘要
In the present work, a surrogate model based on the Random Forest (RF) machine learning is employed for transforming the First-order Shear Deformation Theory (FSDT) based solutions to elasticity based solutions. The bending behavior of laminated composite plates and shells is investigated to demonstrate the capability of such surrogate-assisted computational bridging. In the proposed approach, the surrogate model predicts the difference in stress and displacement between the values obtained using FSDT and Elasticity, which are thereby adjusted to the FSDT predictions for obtaining more accurate values. It leads to an accuracy of elasticity solutions, while having the computational expense of FSDT. The number of layers, thickness, the orientation of each layer, material properties, and geometric properties of plates and shells are considered as input variables used for training RF-based surrogate model. The accuracy of the proposed methodology has been determined by comparing the upgraded results with those available in the literature. The RF-based surrogate model can upgrade the FSDT-based governing behavior to more accurate 3D Elasticity based solutions, thus setting a milestone in coupling ML with composite theories to predict the behavior of laminated composite plates and shells more accurately with a low level of computational expenses.
更多查看译文
关键词
Laminated composite plates and shells,Random Forest,Machine learning,Bending,Surrogate modeling
AI 理解论文
溯源树
样例
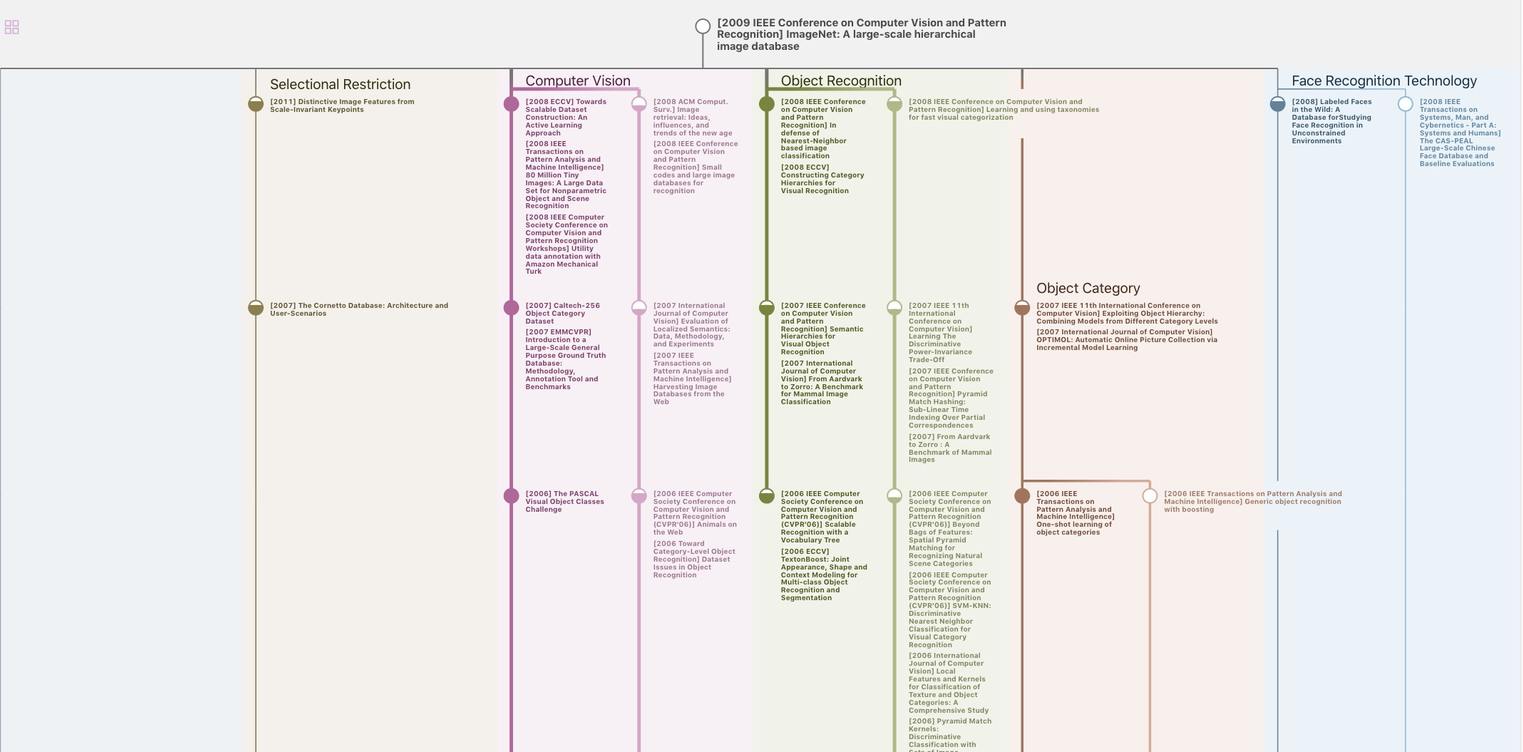
生成溯源树,研究论文发展脉络
Chat Paper
正在生成论文摘要