Learning Optical Flow and Scene Flow with Bidirectional Camera-LiDAR Fusion
IEEE Trans. Pattern Anal. Mach. Intell.(2023)
摘要
In this paper, we study the problem of jointly estimating the optical flow
and scene flow from synchronized 2D and 3D data. Previous methods either employ
a complex pipeline that splits the joint task into independent stages, or fuse
2D and 3D information in an “early-fusion” or “late-fusion” manner. Such
one-size-fits-all approaches suffer from a dilemma of failing to fully utilize
the characteristic of each modality or to maximize the inter-modality
complementarity. To address the problem, we propose a novel end-to-end
framework, which consists of 2D and 3D branches with multiple bidirectional
fusion connections between them in specific layers. Different from previous
work, we apply a point-based 3D branch to extract the LiDAR features, as it
preserves the geometric structure of point clouds. To fuse dense image features
and sparse point features, we propose a learnable operator named bidirectional
camera-LiDAR fusion module (Bi-CLFM). We instantiate two types of the
bidirectional fusion pipeline, one based on the pyramidal coarse-to-fine
architecture (dubbed CamLiPWC), and the other one based on the recurrent
all-pairs field transforms (dubbed CamLiRAFT). On FlyingThings3D, both CamLiPWC
and CamLiRAFT surpass all existing methods and achieve up to a 47.9% reduction
in 3D end-point-error from the best published result. Our best-performing
model, CamLiRAFT, achieves an error of 4.26% on the KITTI Scene Flow
benchmark, ranking 1st among all submissions with much fewer parameters.
Besides, our methods have strong generalization performance and the ability to
handle non-rigid motion. Code is available at
https://github.com/MCG-NJU/CamLiFlow.
更多查看译文
AI 理解论文
溯源树
样例
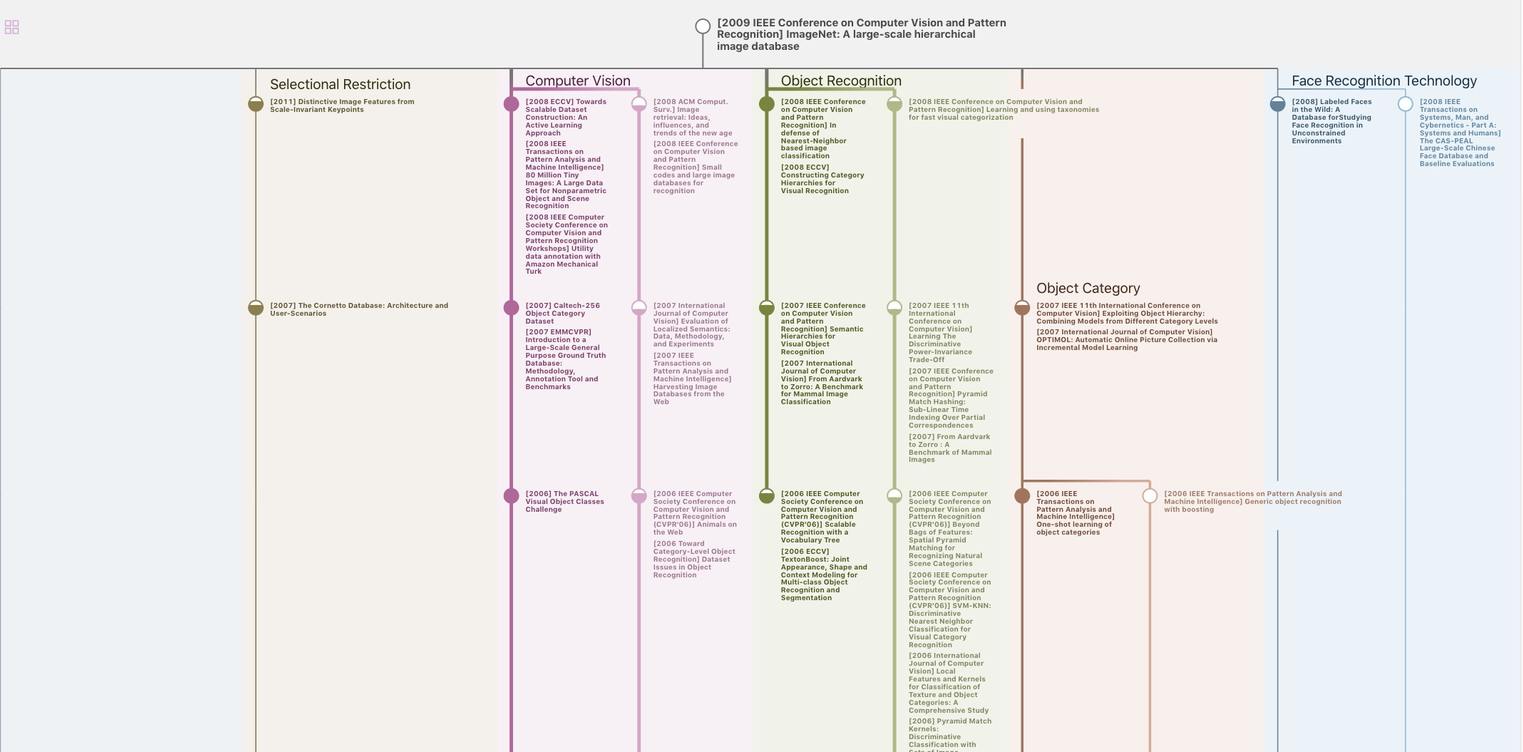
生成溯源树,研究论文发展脉络
Chat Paper
正在生成论文摘要