Convergence of variational Monte Carlo simulation and scale-invariant pre-training
arxiv(2023)
摘要
We provide theoretical convergence bounds for the variational Monte Carlo
(VMC) method as applied to optimize neural network wave functions for the
electronic structure problem. We study both the energy minimization phase and
the supervised pre-training phase that is commonly used prior to energy
minimization. For the energy minimization phase, the standard algorithm is
scale-invariant by design, and we provide a proof of convergence for this
algorithm without modifications. The pre-training stage typically does not
feature such scale-invariance. We propose using a scale-invariant loss for the
pretraining phase and demonstrate empirically that it leads to faster
pre-training.
更多查看译文
关键词
stochastic gradient descent,variational monte carlo simulation,parameterized sphere,monte carlo simulation
AI 理解论文
溯源树
样例
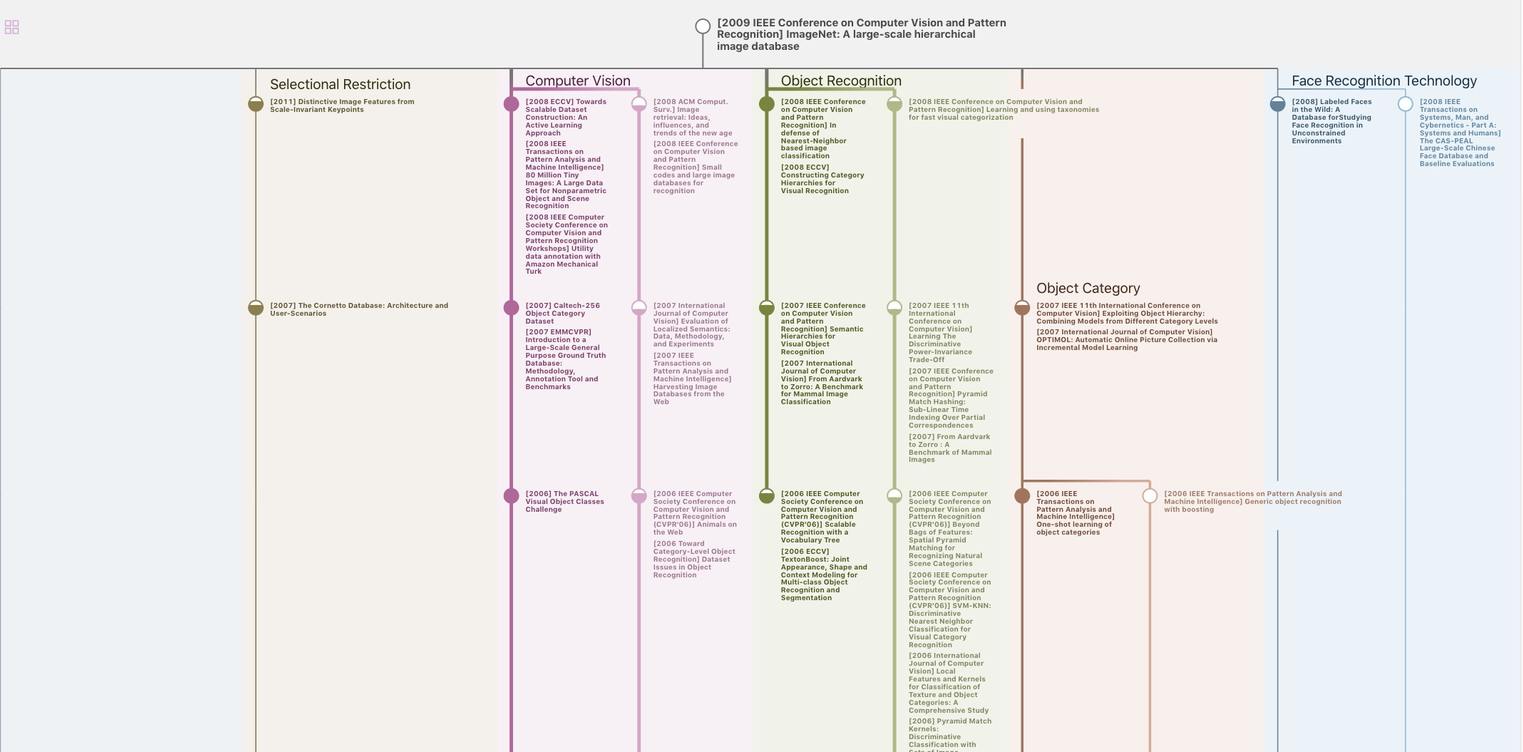
生成溯源树,研究论文发展脉络
Chat Paper
正在生成论文摘要