Bounding System-Induced Biases in Recommender Systems with A Randomized Dataset
arxiv(2023)
摘要
Debiased recommendation with a randomized dataset has shown very promising results in mitigating the system-induced biases. However, it still lacks more theoretical insights or an ideal optimization objective function compared with the other more well studied route without a randomized dataset. To bridge this gap, we study the debiasing problem from a new perspective and propose to directly minimize the upper bound of an ideal objective function, which facilitates a better potential solution to the system-induced biases. Firstly, we formulate a new ideal optimization objective function with a randomized dataset. Secondly, according to the prior constraints that an adopted loss function may satisfy, we derive two different upper bounds of the objective function, i.e., a generalization error bound with the triangle inequality and a generalization error bound with the separability. Thirdly, we show that most existing related methods can be regarded as the insufficient optimization of these two upper bounds. Fourthly, we propose a novel method called debiasing approximate upper bound with a randomized dataset (DUB), which achieves a more sufficient optimization of these upper bounds. Finally, we conduct extensive experiments on a public dataset and a real product dataset to verify the effectiveness of our DUB.
更多查看译文
关键词
System-induced bias,recommender systems,randomized dataset,upper bound minimization
AI 理解论文
溯源树
样例
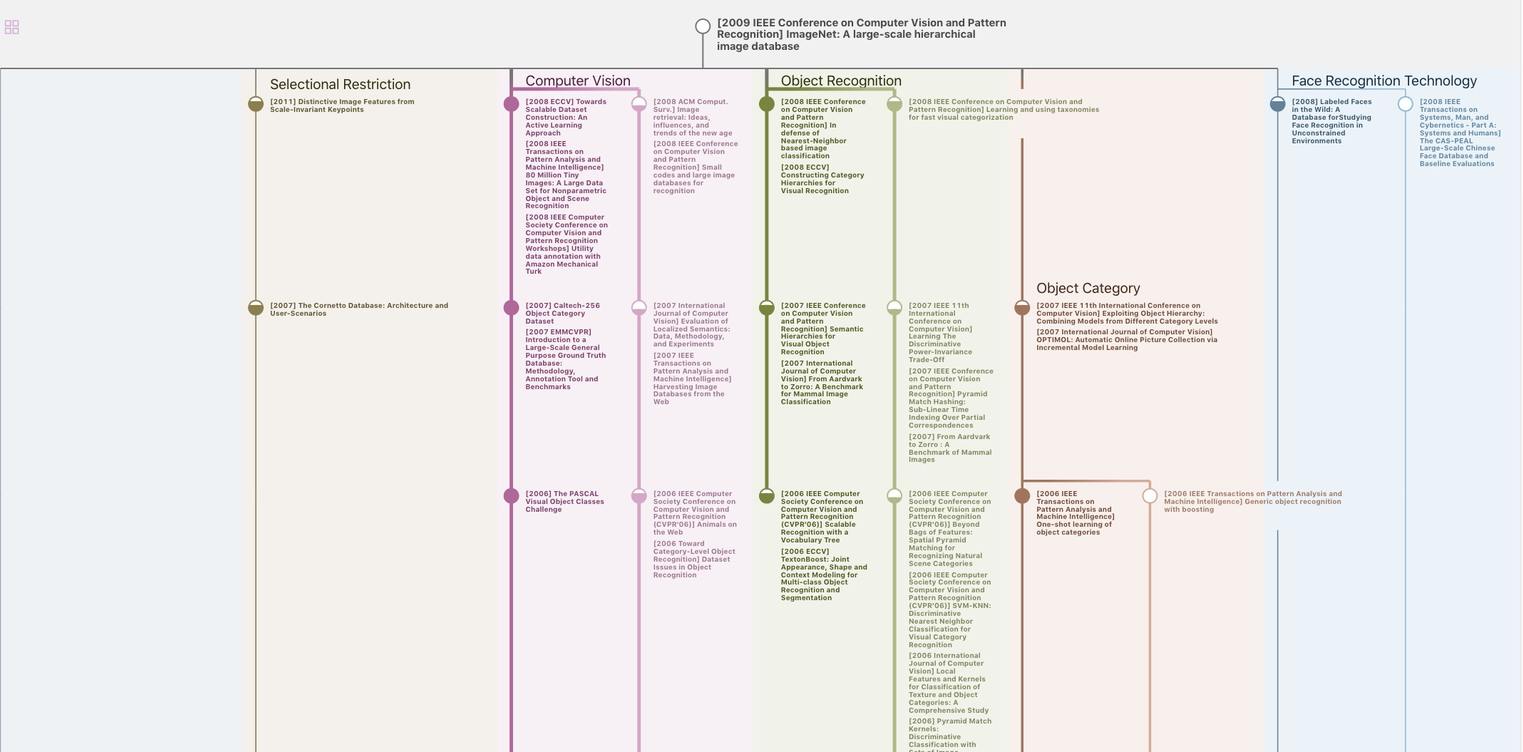
生成溯源树,研究论文发展脉络
Chat Paper
正在生成论文摘要