How (Implicit) Regularization of ReLU Neural Networks Characterizes the Learned Function -- Part II: the Multi-D Case of Two Layers with Random First Layer
arxiv(2023)
摘要
Randomized neural networks (randomized NNs), where only the terminal layer's weights are optimized constitute a powerful model class to reduce computational time in training the neural network model. At the same time, these models generalize surprisingly well in various regression and classification tasks. In this paper, we give an exact macroscopic characterization (i.e., a characterization in function space) of the generalization behavior of randomized, shallow NNs with ReLU activation (RSNs). We show that RSNs correspond to a generalized additive model (GAM)-typed regression in which infinitely many directions are considered: the infinite generalized additive model (IGAM). The IGAM is formalized as solution to an optimization problem in function space for a specific regularization functional and a fairly general loss. This work is an extension to multivariate NNs of prior work, where we showed how wide RSNs with ReLU activation behave like spline regression under certain conditions and if the input is one-dimensional.
更多查看译文
关键词
relu neural networks,learned function,regularization,layers,layers
AI 理解论文
溯源树
样例
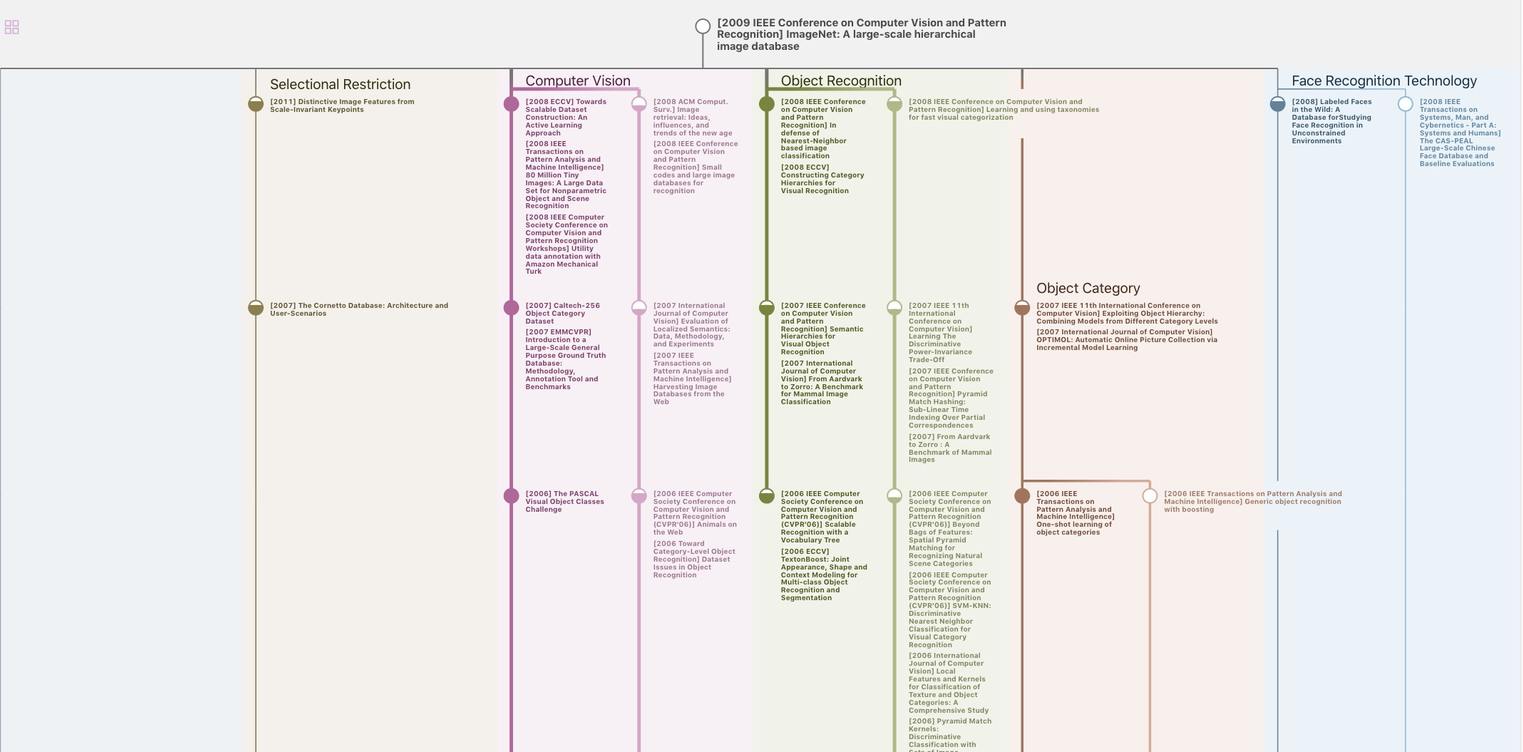
生成溯源树,研究论文发展脉络
Chat Paper
正在生成论文摘要