Composite Analysis-Based Machine Learning for Prediction of Tropical Cyclone-Induced Sea Surface Height Anomaly
IEEE J. Sel. Top. Appl. Earth Obs. Remote. Sens.(2023)
摘要
Sea surface height anomaly (SSHA) induced by tropical cyclones (TCs) is closely associated with oscillations and is a crucial proxy for thermocline structure and ocean heat content in the upper ocean. The prediction of TC-induced SSHA, however, has been rarely investigated. This study presents a new composite analysis-based random forest (RF) approach to predict daily TC wind pump induced SSHA. The proposed method utilizes TC's characteristics and prestorm upper oceanic parameters as input features to predict TC-induced SSHA up to 30 days after TC passage. Simulation results suggest that the proposed method is skillful at inferring both the amplitude and temporal evolution of SSHA induced by TCs of different intensity groups. Using a TC-centered 5° × 5° box, the proposed method achieves highly accurate prediction of TC-induced SSHA over the Western North Pacific with root mean square error of 0.024 m, outperforming alternative machine learning methods and the numerical model. Moreover, the proposed method also demonstrated good prediction performance in different geographical regions, i.e., the South China Sea and the Western North Pacific subtropical ocean. The study provides insight into the application of machine learning in improving the prediction of SSHA influenced by extreme weather conditions. Accurate prediction of TC-induced SSHA allows for better preparedness and response, reducing the impact of extreme events (e.g., storm surge) on people and property.
更多查看译文
关键词
Composite analysis,machine learning,random forest,sea surface height anomaly,tropical cyclones
AI 理解论文
溯源树
样例
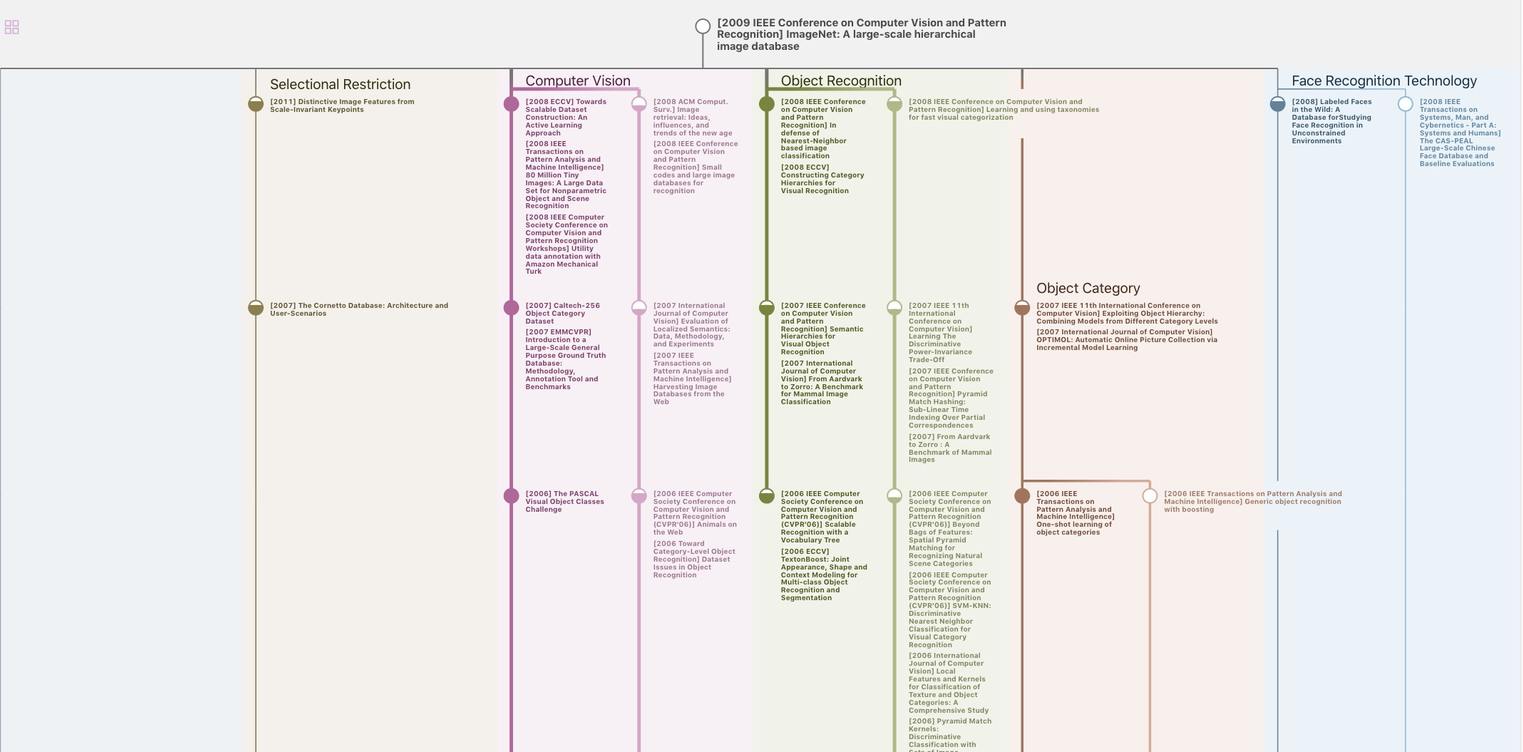
生成溯源树,研究论文发展脉络
Chat Paper
正在生成论文摘要