Adaptive Lightweight License Plate Image Recovery Using Deep Learning Based on Generative Adversarial Network
IEEE Access(2023)
摘要
Many Convolutional Neural Networks (CNNs) methods have already surpassed traditional approaches to image restoration tasks. Those CNNs models were usually designed to enhance single tasks such as an image resolution (super-resolution) or image denoising, but we came up with unconventional goals, that is, multiple recovery tasks from a single network design. Although the Transformer design has recently gained attention in image recovery tasks, they are too slow. In order to work with license plate images from a traffic camera stream, the system has to be responsive. So, we proposed a fast and lightweight deep learning-based data recovery system using a Generative Adversarial Network (GAN) principle named License Plate Recovery GAN (LPRGAN). The design has a proposed encoder-decoder style inspired by an autoencoder aided by dual classification networks. This style suits problem-characteristic learning because strong contextual information is retrieved from the down-scaled representations. This proposed system has three main features such as identifying a problem, data recovery, and fail-safe mechanism. The core of system is a data recovery unit (LPRGAN), is used to recover license plate images from multiple degraded input images. Most existing image restoration systems do not have self-awareness, leading to an inefficiency problem. Unlike existing works, this system has anomaly detection and will only process on a degraded input, reducing workload overhead, improving efficiency and a fail-safe feature that prevents an unexpected bad output. Hence, it is light enough to deploy on a low-power machine such as edge computing devices, opening up new possibilities in on-device computing. Our proposed research can recover several degraded problems up to 720p resolution at 15 frames per second on a single graphic card, $256\times 128$ resolution at 17 frames per second on a CPU-only workstation machine, or 7 frames per second on an ultra-low-power tablet PC.
更多查看译文
关键词
Data recovery,deep learning,generative adversarial networks,image and video recovery,machine learning,neural networks
AI 理解论文
溯源树
样例
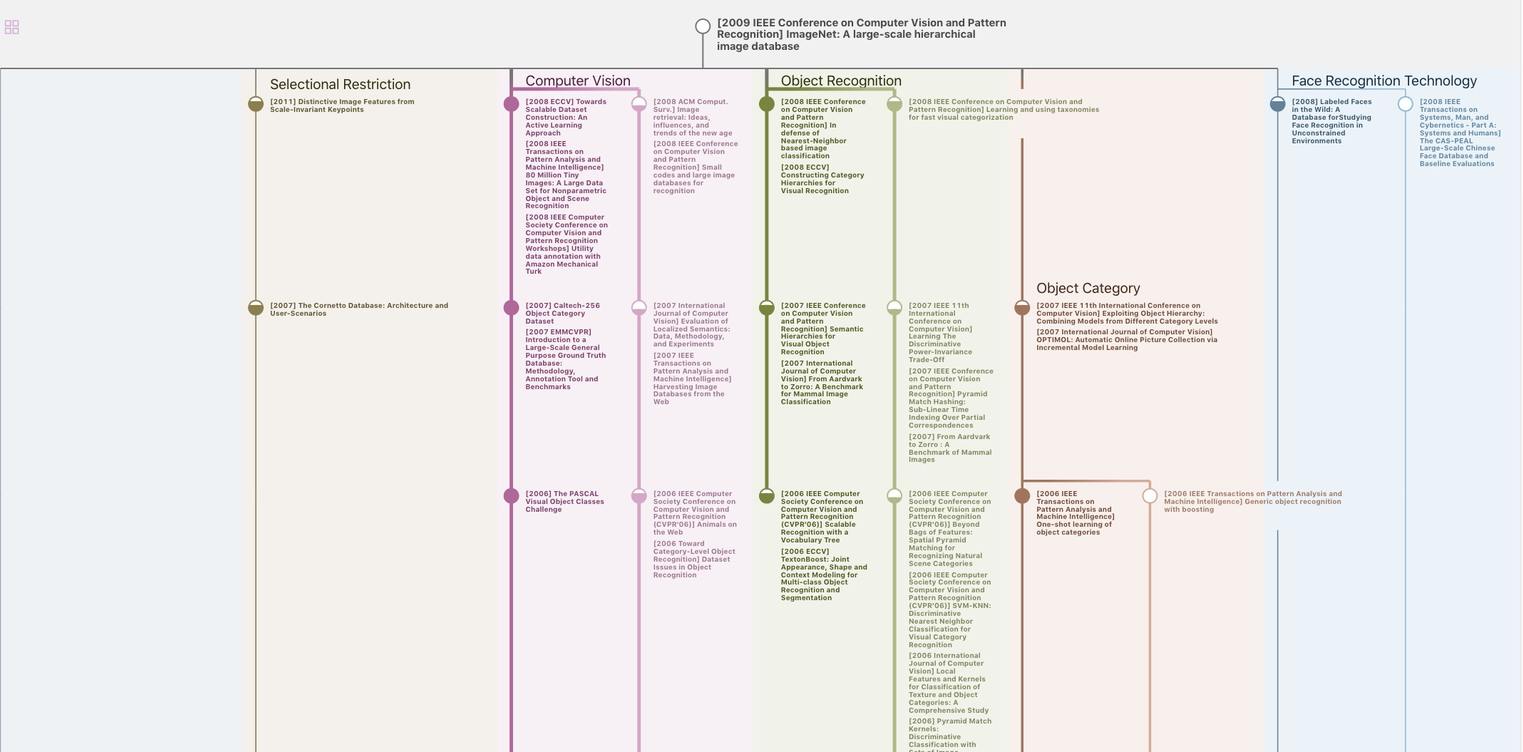
生成溯源树,研究论文发展脉络
Chat Paper
正在生成论文摘要