Machine Learning to Identify Molecular Markers for Metabolic Disease Development Using Mouse Models
biorxiv(2023)
摘要
Background Aging, Western diet (WD) intake, and bile acid (BA) receptor farnesoid X receptor (FXR) inactivation are risk factors for metabolic disease development including nonalcoholic fatty liver disease (NAFLD) and chronic inflammation-related health issues such as dementia. The progression of the metabolic disease can be escalated when those risks are combined. Inactivation of FXR is cancer prone in both humans and mice. The current study used omics data generated within the gut-liver axis to classify those risks using bioinformatics and machine learning approaches.
Methods Different ages (5, 10, and 15 months) of wild-type (WT) and FXR knockout (KO) male mice were fed with either a healthy control diet (CD) or a WD since weaning. Hepatic transcripts, liver, serum, and urine metabolites, hepatic bile acids (BAs), as well as gut microbiota were used for risk prediction. A linear support vector machine with K -fold cross-validation was used for classification and feature selection.
Results Increased urine sucrose alone achieved 91% accuracy in predicting WD intake. Hepatic lithocholic acid (LCA) and serum pyruvate had 100% and 95% accuracy, respectively to classify age. Association analyses showed hepatic LCA was positively associated with serum concentrations of acetone, a ketone body, and 1,3-dihydroxyacetone (DHA), but negatively correlated with serum pyruvate. Urine metabolites (decreased creatinine and taurine as well as increased succinate) or gut microbiota (increased Dorea, Dehalobacterium , and Oscillospira ) could predict FXR functional status with greater than 90% accuracy. Integrated pathway analyses revealed that the predictors for diet and FXR expression were implicated in the central carbon metabolism in cancer. To assess the translational relevance, mouse hepatic transcripts were crosschecked with human NAFLD and hepatocellular carcinoma (HCC) datasets. WD-affected hepatic Cyp39a1 and Gramd1b expression were associated with human HCC and NAFLD, respectively. The metabolites and diseases interaction analyses uncovered that the identified features are implicated in human metabolic diseases, mental disorders, and cancer.
Conclusion The risk prediction using mouse models contributes to the identification of noninvasive biomarkers for early diagnosis of metabolic disease development.
### Competing Interest Statement
The authors have declared no competing interest.
* Acmsd
: aminocarboxymuconate semialdehyde decarboxylase
Adam11
: ADAM metallopeptidase domain 11
A4gnt
: alpha-1,4-N-acetylglucosaminyltransferase
CD
: control diet
Cdh19
: cadherin 19
Cyp39a1
: cytochrome P450 family 39 subfamily a member 1
Cyp3a11
: cytochrome P450 family 3 subfamily a member
Cyp46a1
: cytochrome P450 family 46 subfamily a member 1
Csad
: cysteine sulfinic acid decarboxylase
DEGs
: differentially expressed genes
FXR
: farnesoid X receptor
GEO
: Gene Expression Omnibus
Gramd1b
: GRAM domain containing 1B
Hamp2
: hepcidin antimicrobial peptide 2
HCC
: hepatocellular carcinoma
Hsf2bp
: heat shock transcription factor 2 binding protein
Kcnj16
: potassium inwardly rectifying channel subfamily J member 16
Loxl4
: lysyl oxidase like 4
ML
: machine learning
Mfsd2a
: major facilitator superfamily domain containing 2A
Mmd2
: monocyte to macrophage differentiation associated 2
NAFLD
: nonalcoholic fatty liver disease
NAFL
: nonalcoholic fatty liver
NASH
: non-alcoholic steatohepatitis
Naip2
: NLR family apoptosis inhibitory protein
Pclo
: piccolo presynaptic cytomatrix protein
Pde5a
: phosphodiesterase 5A
Rec8
: REC8 meiotic recombination protein
Rps27rt
: Ribosomal Protein S27
SVM
: support vector machine
Slc39a4
: solute carrier family 39 member 4
Tdg
: thymine DNA gycosylase
WD
: Western diet
Zbtb16
: zinc finger and BTB domain containing 16
Zfp677
: zinc finger protein
更多查看译文
关键词
metabolic disease development,molecular markers,mouse models
AI 理解论文
溯源树
样例
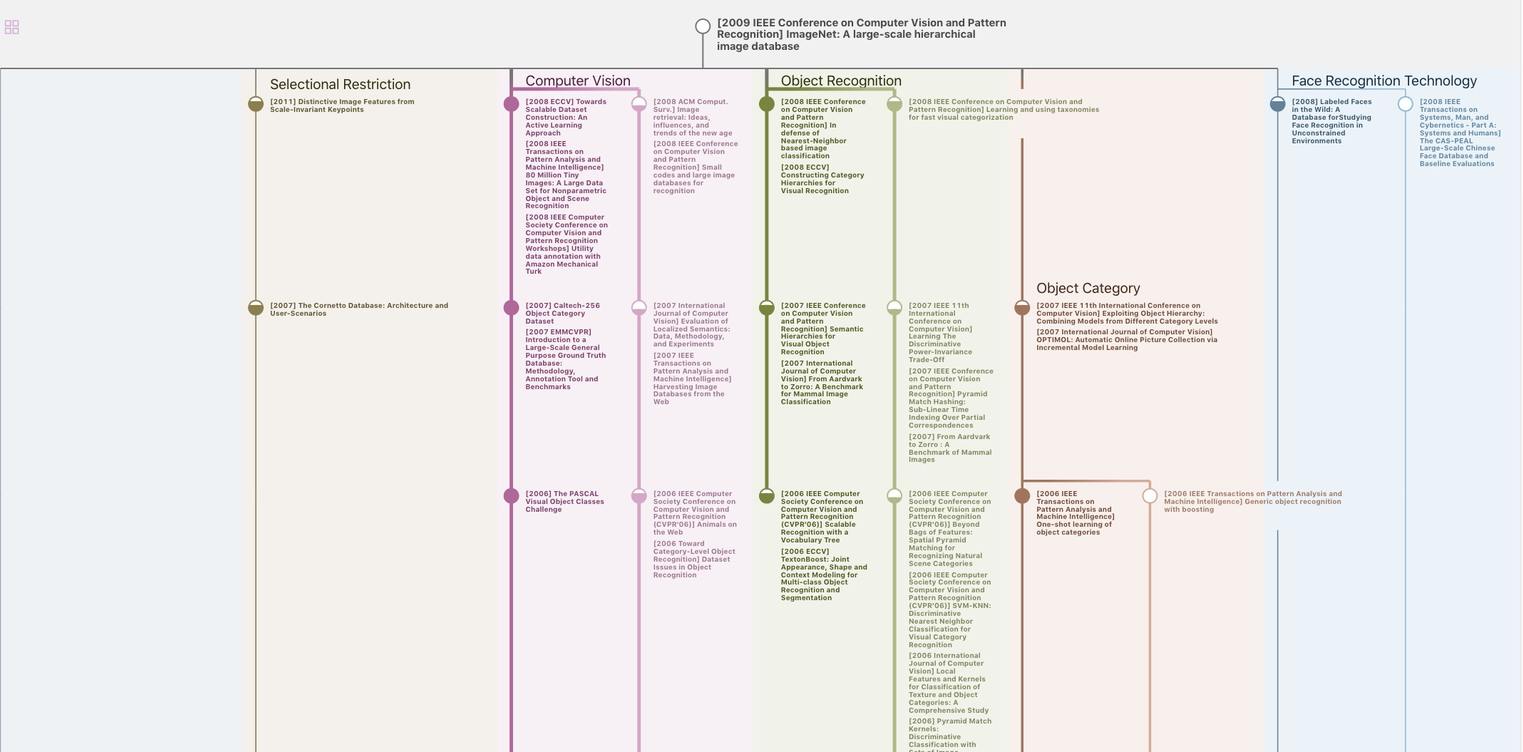
生成溯源树,研究论文发展脉络
Chat Paper
正在生成论文摘要