Towards Interpretable Video Anomaly Detection
WACV(2023)
摘要
Most video anomaly detection approaches are based on data-intensive end-to-end trained neural networks, which extract spatiotemporal features from videos. The extracted feature representations in such approaches are not interpretable, which prevents the automatic identification of anomaly cause. To this end, we propose a novel framework which can explain the detected anomalous event in a surveillance video. In addition to monitoring objects independently, we also monitor the interactions between them to detect anomalous events and explain their root causes. Specifically, we demonstrate that the scene graphs obtained by monitoring the object interactions provide an interpretation for the context of the anomaly while performing competitively with respect to the recent state-of-the-art approaches. Moreover, the proposed interpretable method enables cross-domain adaptability (i.e., transfer learning in another surveillance scene), which is not feasible for most existing end-to-end methods due to the lack of sufficient labeled training data for every surveillance scene. The quick and reliable detection performance of the proposed method is evaluated both theoretically (through an asymptotic optimality proof) and empirically on the popular benchmark datasets.
更多查看译文
关键词
Algorithms: Video recognition and understanding (tracking,action recognition,etc.),Machine learning architectures,formulations,and algorithms (including transfer,low-shot,semi-,self-,and un-supervised learning)
AI 理解论文
溯源树
样例
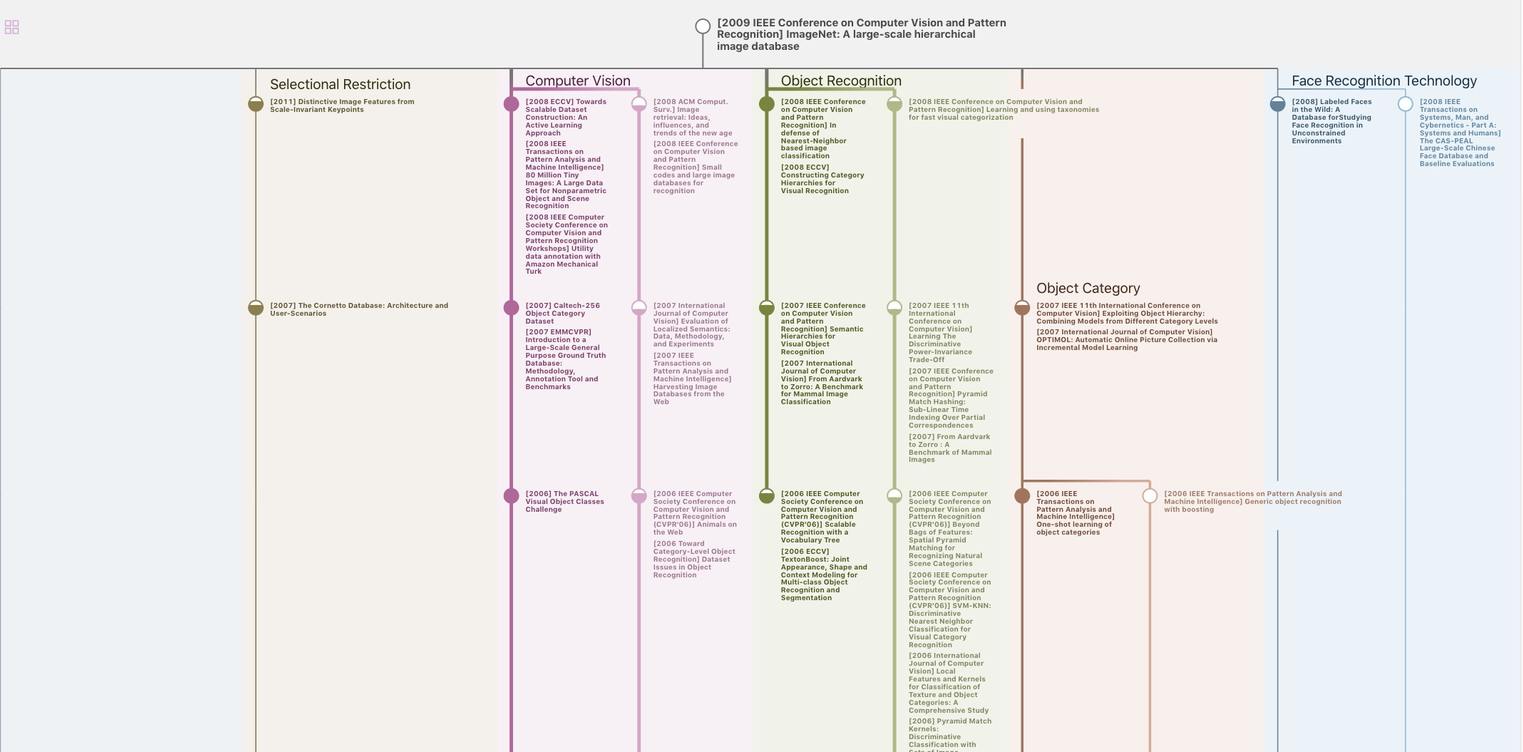
生成溯源树,研究论文发展脉络
Chat Paper
正在生成论文摘要