AnoLeaf: Unsupervised Leaf Disease Segmentation via Structurally Robust Generative Inpainting
WACV(2023)
摘要
Plant diseases severely limits agriculture production, necessitating the high-throughput monitoring of plant leaves. Currently, this is formulated as an automatic disease segmentation task addressed via deep learning frameworks. These deep leaning frameworks trained with leaf image data in a supervised paradigm have few limitations, mainly: (1) training datasets are heavily imbalanced towards healthy leaf images, (2) disease region annotation is labour-intensive and (3) due to the heterogeneity of disease symptoms, these frameworks lacks generalisability. In this paper, we reformulate disease segmentation as an anomaly localisation task. Specifically, we introduce a novel unsupervised framework (AnoLeaf) based on an edge-guided inpainting that optimises the learning of contextual attention on only healthy leaf images. The network utilisation on diseased leaf images results in reconstruction of its healthy counterparts, generating an inpainting error. The contextual attention maps reinforce the inpainting error to effectively localise the disease. Thus, AnoLeaf alleviates the acquisition and annotation of rare disease images. Additional experiments on MVTec anomaly detection dataset further demonstrate its generalisability.
更多查看译文
关键词
unsupervised anoleaf disease segmentation
AI 理解论文
溯源树
样例
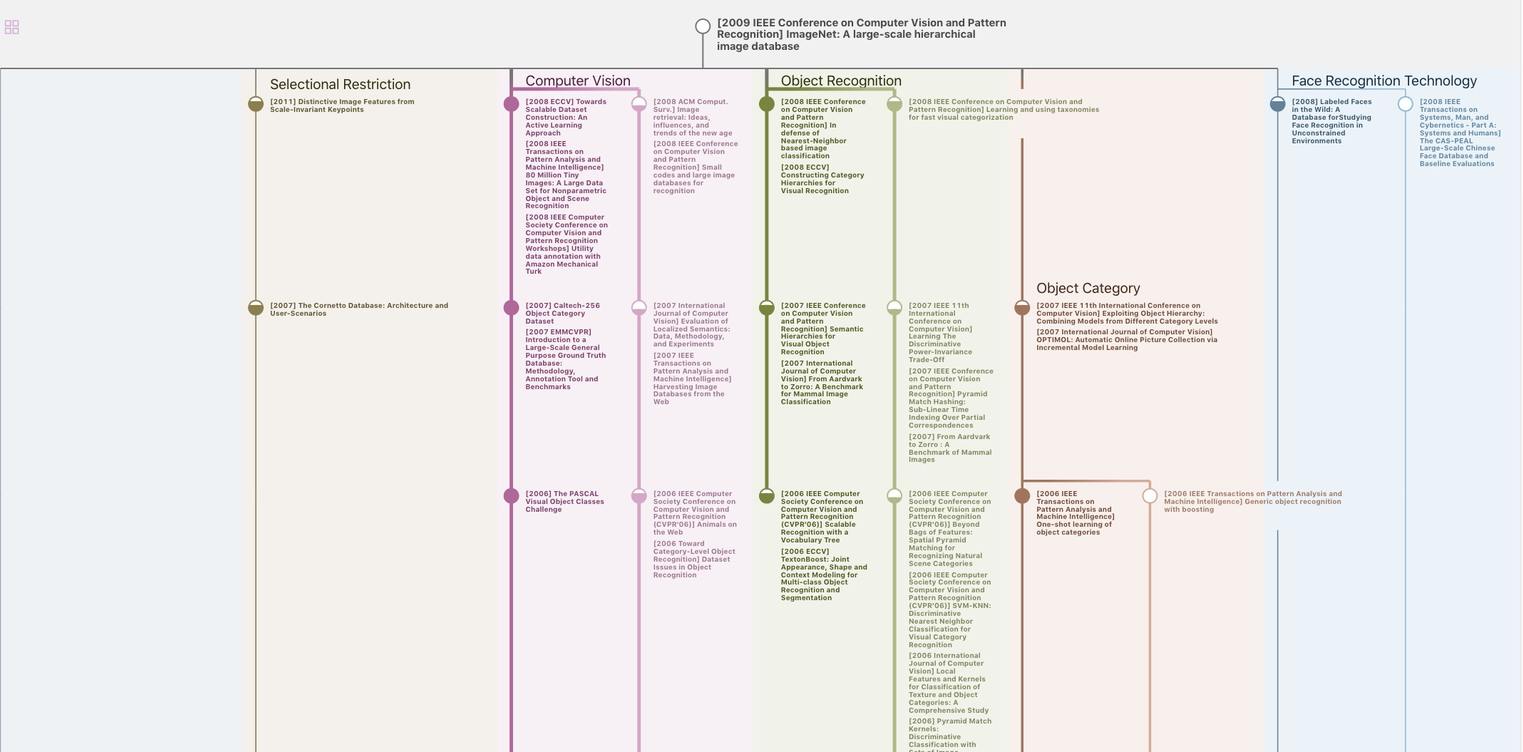
生成溯源树,研究论文发展脉络
Chat Paper
正在生成论文摘要