No Reference Opinion Unaware Quality Assessment of Authentically Distorted Images
WACV(2023)
摘要
The quality assessment (QA) of camera captured authentically distorted images is important on account of its ubiquitous applications and challenging due to the lack of a reference. While there exists a plethora of supervised no reference (NR) image QA (IQA) algorithms, there is a need to study unsupervised or opinion unaware algorithms on account of their superior generalization performance. We explore self-supervised learning (SSL) for the feature design on authentically distorted images to predict quality without training on human labels. While SSL on synthetic distortions has recently shown promise, there is a need to enrich the feature learning on authentic distortions. The key challenge in achieving this is in the learning of quality sensitive features with mitigated content dependence. We design a self-supervised contrastive learning approach which only requires positives and introduce a content separation loss by estimating a bound on the mutual information between the features learnt and the content information. We show on multiple authentically distorted datasets that our selfsupervised features can predict image quality by comparing with a corpus of pristine images and achieve state-of-the-art performance.
更多查看译文
关键词
quality,images
AI 理解论文
溯源树
样例
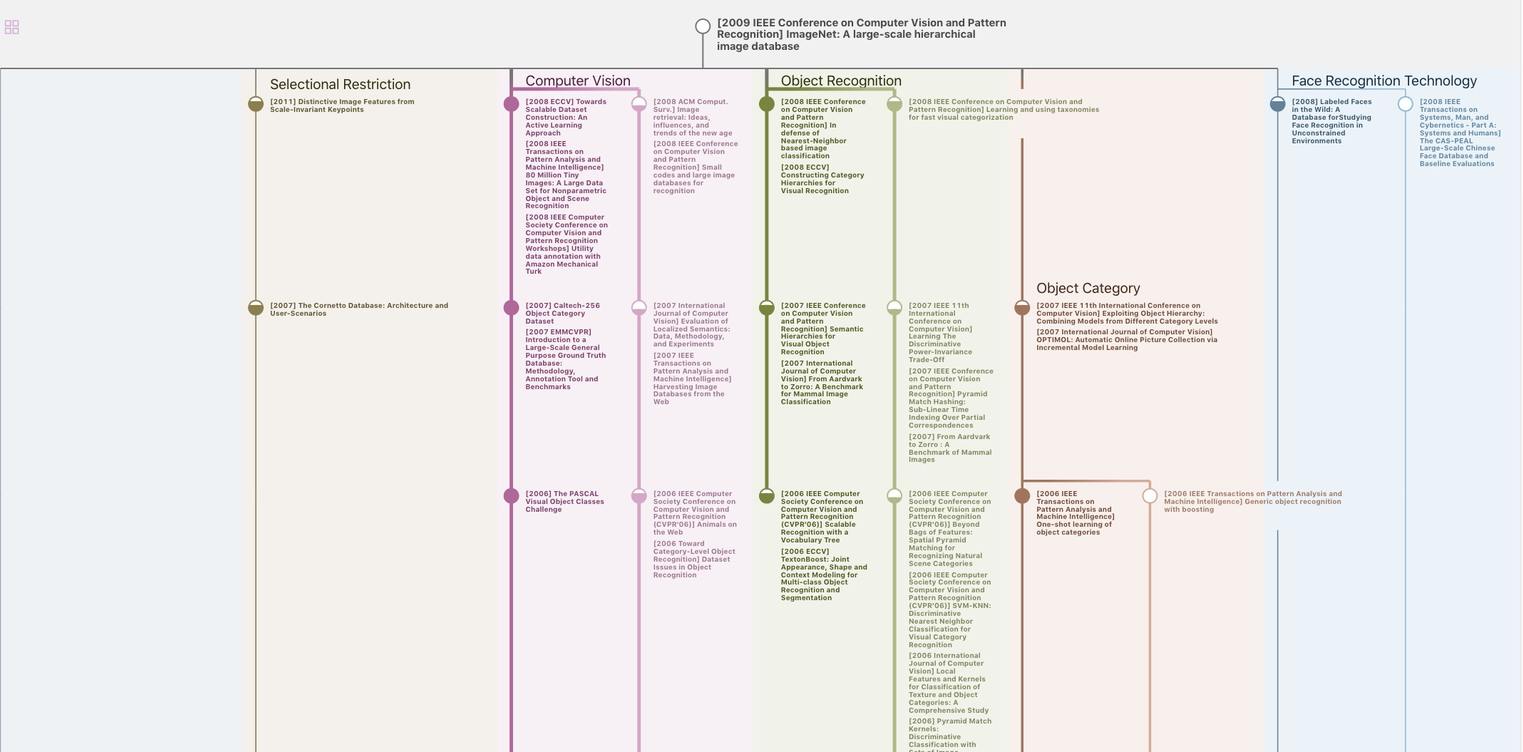
生成溯源树,研究论文发展脉络
Chat Paper
正在生成论文摘要