Meta-OLE: Meta-learned Orthogonal Low-Rank Embedding
WACV(2023)
摘要
We introduce Meta-OLE, a new geometry-regularized method for fast adaptation to novel tasks in few-shot image classification. The proposed method learns to adapt for each few-shot classification task a feature space with simultaneous inter-class orthogonality and intra-class low-rankness. Specifically, a deep feature extractor is trained by explicitly imposing orthogonal low-rank subspace structures among features corresponding to different classes within a given task. To adapt to novel tasks with unseen categories, we further meta-learn a light-weight transformation to enhance the inter-class margins. As an additional benefit, this light-weight transformation lets us exploit the query data for label propagation from labeled to unlabeled data without any auxiliary network components. The explicitly geometry-regularized feature subspaces allow the classifiers on novel tasks to be inferred in a closed form, with an adaptive subspace truncation that selectively discards non-discriminative dimensions. We perform experiments on standard few-shot image classification tasks, and observe performance superior to state-of-the-art meta-learning methods.
更多查看译文
关键词
Algorithms: Machine learning architectures,formulations,and algorithms (including transfer),Image recognition and understanding (object detection,categorization,segmentation,scene modeling,visual reasoning)
AI 理解论文
溯源树
样例
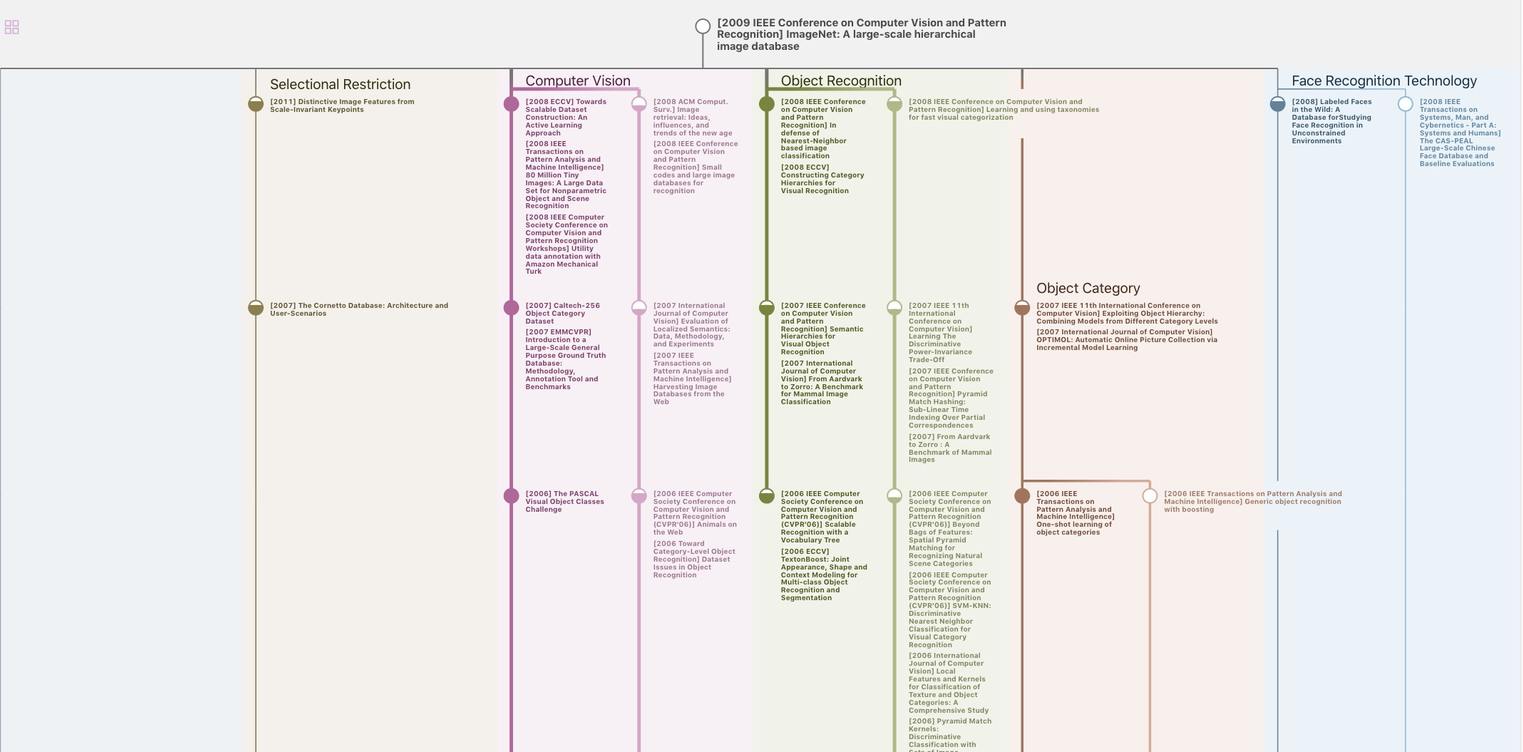
生成溯源树,研究论文发展脉络
Chat Paper
正在生成论文摘要