Single StageWeakly Supervised Semantic Segmentation of Complex Scenes
WACV(2023)
摘要
The costly process of obtaining semantic segmentation labels has driven research towards weakly supervised semantic segmentation (WSSS) methods, using only image-level, point, or box labels. Such annotations introduce limitations and challenges that results in overly-tuned methods specialized in specific domains or scene types. The over reliance of image-level based methods on generation of high quality class activation maps (CAMs) results in limited applicable dataset complexity range, mostly focusing on object centric scenes. Additionally, the lack of dense annotations requires methods to increase network complexity to obtain additional semantic information, often done through multiple stages of training and refinement. Here, we present a single-stage approach generalizable to a wide range of dataset complexities, that is trainable from scratch, without any dependency on pre-trained backbones, classification, or separate refinement tasks. We utilize point annotations to generate reliable, on-the-fly pseudo-masks through refined and spatially filtered features. We are to demonstrate SOTA performance on benchmark datasets (PascalVOC 2012), as well as significantly outperform other SOTA WSSS methods on recent real-world datasets (CRAID, CityPersons, IAD, ADE20K, CityScapes) with up to 28.1% and 22.6% performance boosts compared to our single-stage and multi-stage baselines respectively.
更多查看译文
关键词
segmentation,scenes,stage
AI 理解论文
溯源树
样例
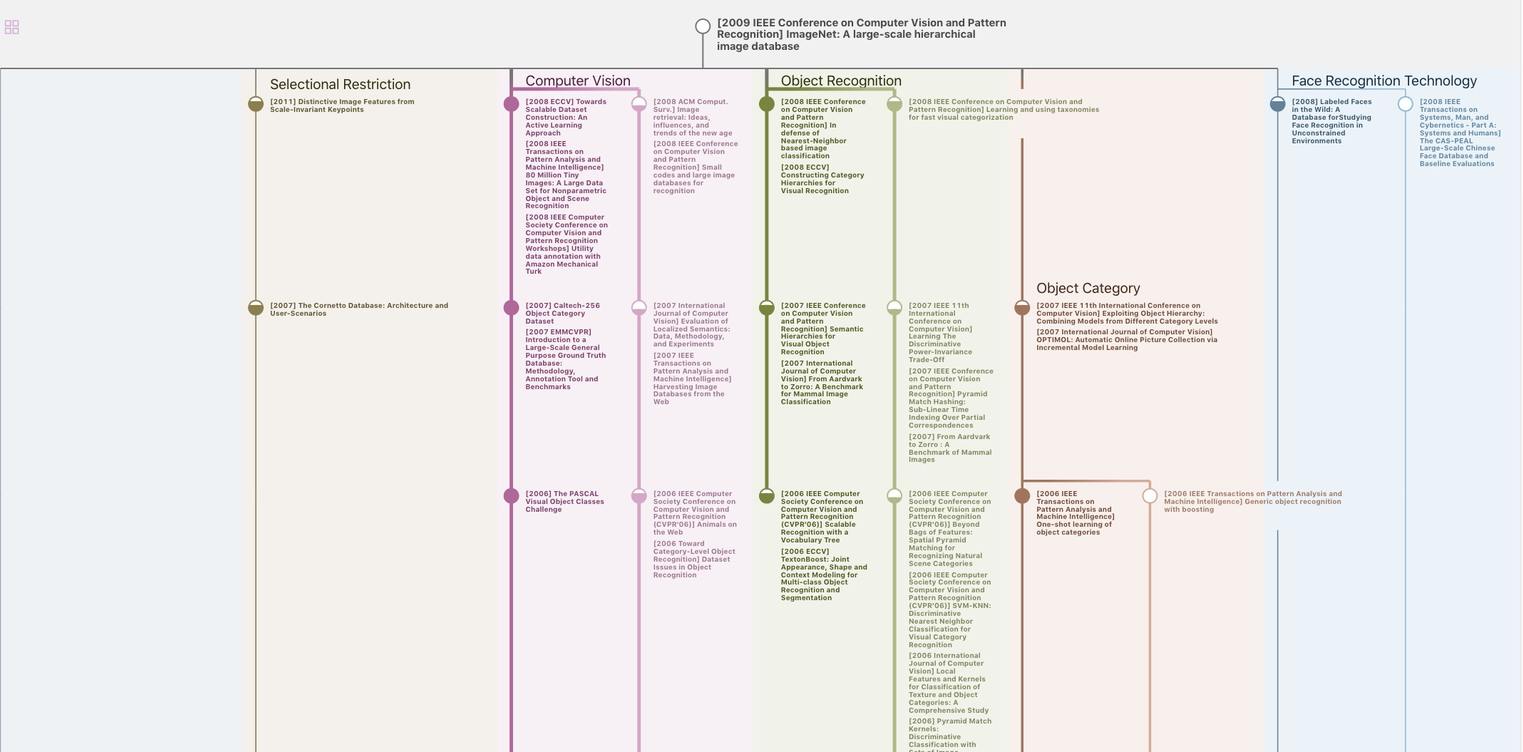
生成溯源树,研究论文发展脉络
Chat Paper
正在生成论文摘要