A Systematic Literature Review on Multimodal Machine Learning: Applications, Challenges, Gaps and Future Directions.
IEEE Access(2023)
摘要
Multimodal machine learning (MML) is a tempting multidisciplinary research area where heterogeneous data from multiple modalities and machine learning (ML) are combined to solve critical problems. Usually, research works use data from a single modality, such as images, audio, text, and signals. However, real-world issues have become critical now, and handling them using multiple modalities of data instead of a single modality can significantly impact finding solutions. ML algorithms play an essential role in tuning parameters in developing MML models. This paper reviews recent advancements in the challenges of MML, namely: representation, translation, alignment, fusion and co-learning, and presents the gaps and challenges. A systematic literature review (SLR) was applied to define the progress and trends on those challenges in the MML domain. In total, 1032 articles were examined in this review to extract features like source, domain, application, modality, etc. This research article will help researchers understand the constant state of MML and navigate the selection of future research directions.
更多查看译文
关键词
Market research,Visualization,Systematics,Representation learning,Medical services,Biomedical imaging,Bibliographies,Multimodal machine learning,systematic literature review,representation,translation,alignment,fusion,co-learning
AI 理解论文
溯源树
样例
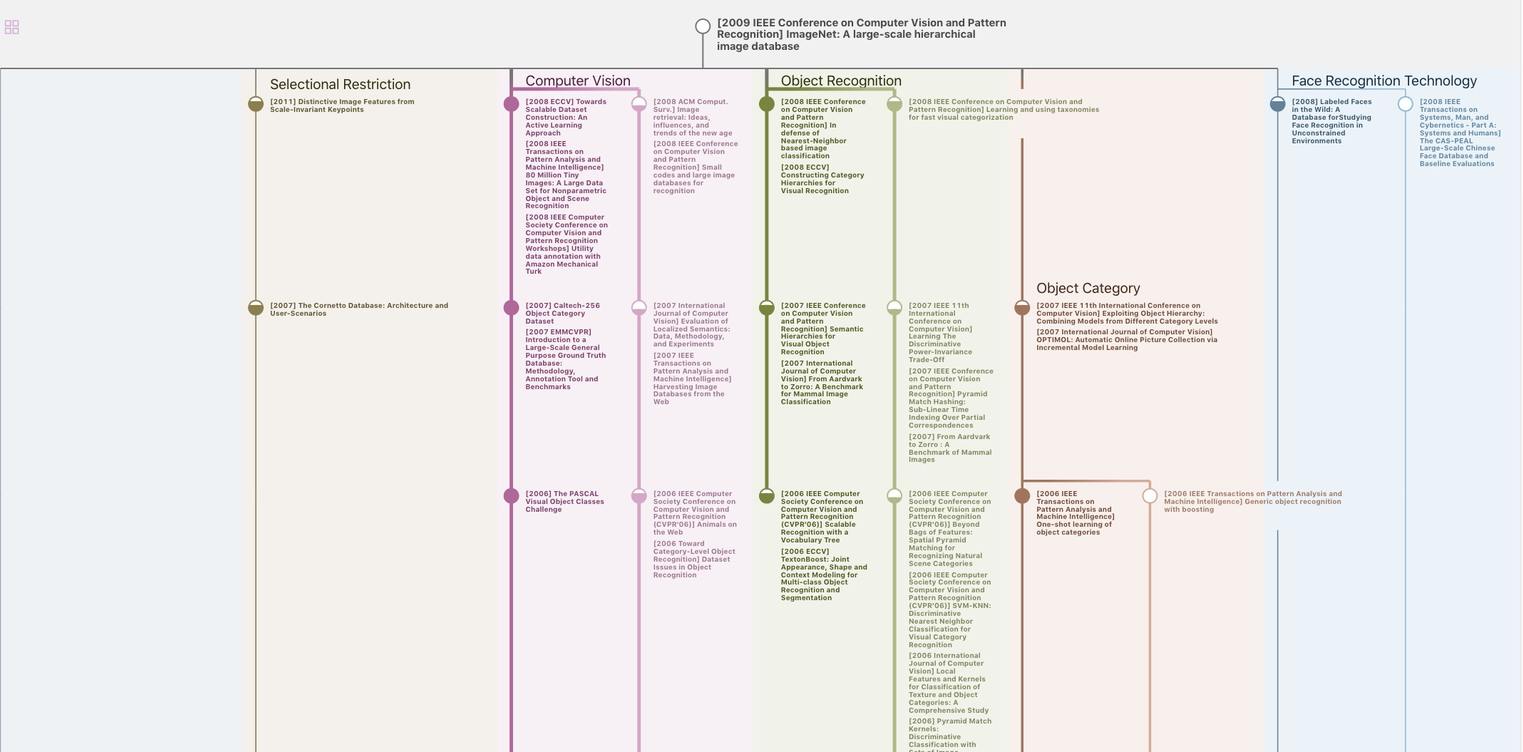
生成溯源树,研究论文发展脉络
Chat Paper
正在生成论文摘要