Optimized Model Selection for Concurrent Decoding of Finger Kinetics and Kinematics.
IEEE Access(2023)
摘要
Myoelectric-based motor intent detection is typically used to interface with assistive devices. However, the intent detection performance is sensitive to interference of electromyogram (EMG) signals. Recently, EMG signals are decomposed into motor units (MU) firing activities, and neuron binary firing activities can be used to predict motor output in a continuous manner. Different functions that map MU firings to motor output have been implemented, and both composite MU firing frequency and individual MU firing frequency have been used. It is unclear whether one mapping function outperform others. Accordingly, we evaluated three MU-based finger kinetic and kinematic prediction models, by varying the number of MUs and the method of including MU firings into the regression model. We also compared the performance of three EMG amplitude-based models with varying number of channels. We performed MU decomposition in advance for real-time implementations. Our results showed that individual firing frequency of five MUs provided the lowest estimation error (force: 4.66 +/- 0.36 %MVC; joint angle: 4.81 +/- 0.49(?)) and highest correlation (force: 0.86 +/- 0.01; joint angle: 0.93 +/- 0.01) with the measured motor outputs, when compared with mapping method using the populational firing frequency of all MUs or the populational firing frequency of a group of MUs with similar firing activities. The results indicated that firing information at the population level may mask critical information of individual MU firings. These findings allowed us to identify the optimal models for concurrent and continuous finger force and joint angle estimation. A combination of the minimal level of complexity and high accuracy make these models suitable for real-time control of assistive robotic devices.
更多查看译文
关键词
Assistive technologies,Kinematics,Fingerprint recognition,Electromyography,Force,Predictive models,Haptic interfaces,Finger force,finger kinematics,hand function,motor unit
AI 理解论文
溯源树
样例
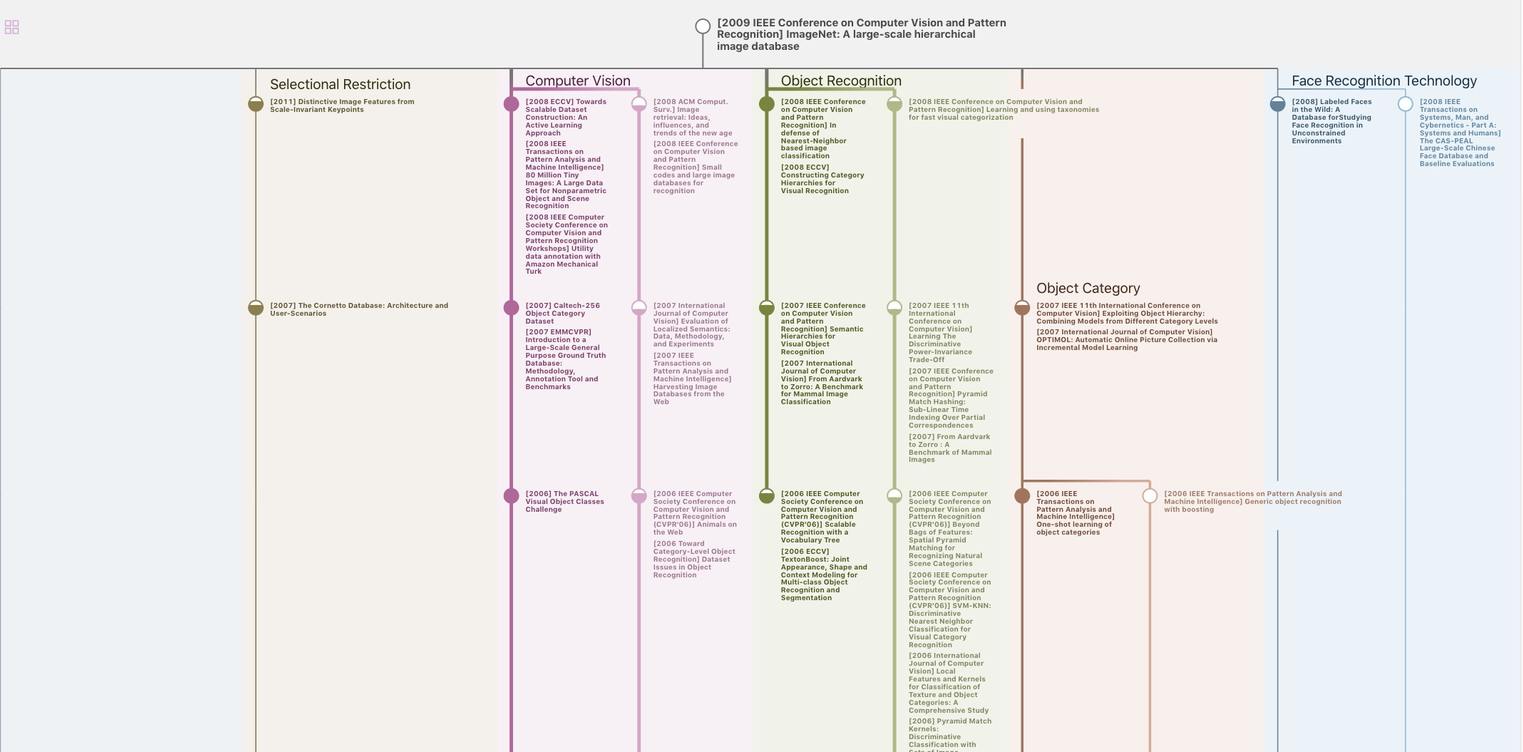
生成溯源树,研究论文发展脉络
Chat Paper
正在生成论文摘要