PICS: Paradigms Integration and Contrastive Selection for Semisupervised Remote Sensing Images Semantic Segmentation.
IEEE Trans. Geosci. Remote. Sens.(2023)
摘要
Remote sensing images semantic segmentation is a fundamental yet challenging task, which has long relied heavily on sufficient pixelwise annotations. Semisupervised learning is proposed to address the problem of high dependence on labeled data by exploiting more learnable samples generated from the large amounts of accessible unlabeled data. However, affected by the complexity and diversity of remote sensing images, various misclassifications often occur and lead to errors accumulation during model training. Errors accumulation will destroy the consistency of model training and lead to degradation of final segmentation performance. In this article, in order to further alleviate the damage caused by the errors to the consistency of model training and improve final segmentation accuracy, we propose a novel semisupervised segmentation framework, paradigms integration and contrastive selection (PICS). First, multiple proven semisupervised paradigms are integrated to generate pseudolabeled samples with less noise. Second, a loss-based contrastive selection method is explored to distinguish generated samples that contain different degrees of inevitable misclassification, thereby further maintaining the approximation of the generated samples and the ground truth in the sample space. By generating and selecting high-quality pseudolabeled samples for selective self-training, we can better guarantee consistency during model training and obtain better segmentation results. Extensive experiments over the ISPRS Vaihingen, Potsdam, and the challenging iSAID benchmarks demonstrate that our method yields significant accuracy boosting on the segmentation results and achieves on-par performance with the state of the arts.
更多查看译文
关键词
remote sensing,contrastive selection,images
AI 理解论文
溯源树
样例
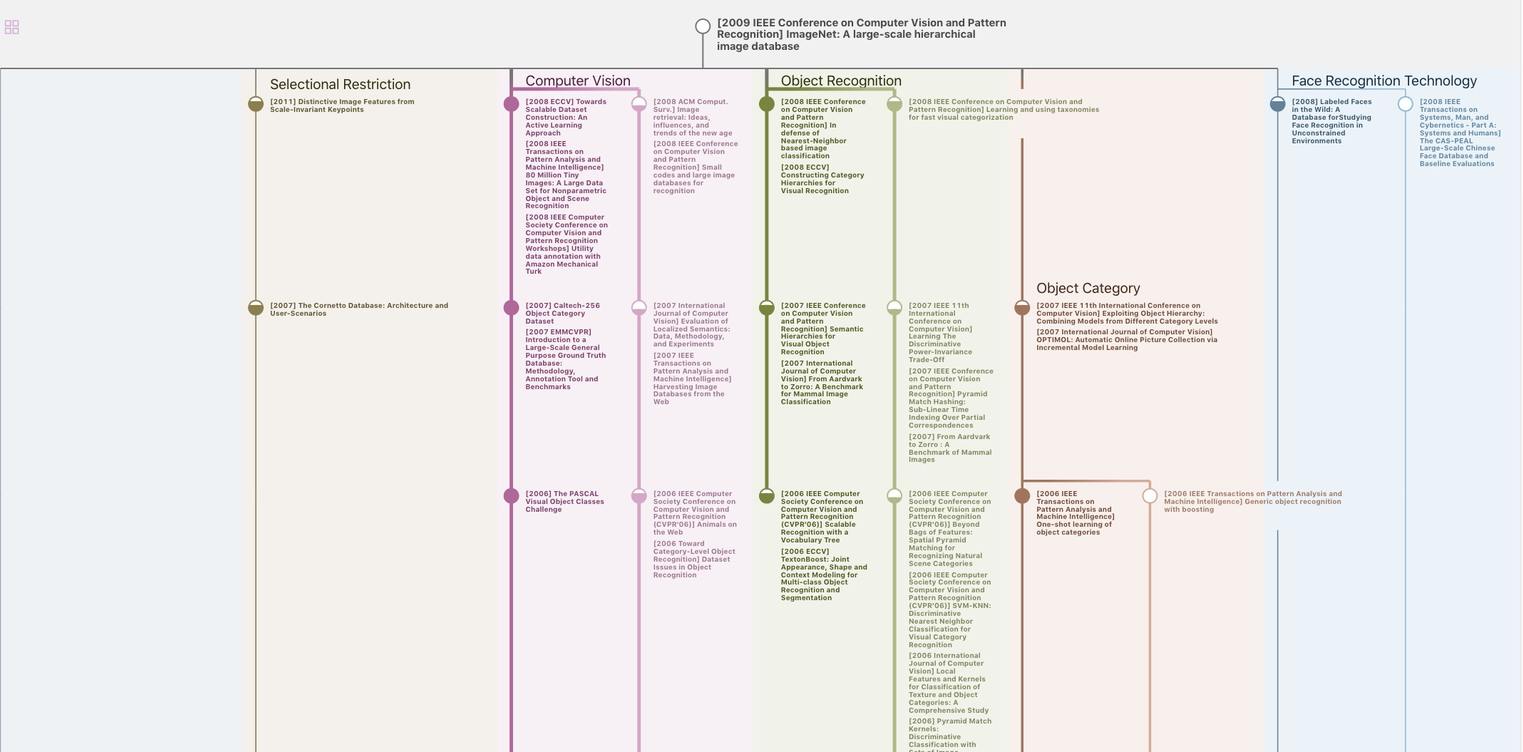
生成溯源树,研究论文发展脉络
Chat Paper
正在生成论文摘要