Automated Extraction of a Depth-Defined Wave Runup Time Series From Lidar Data Using Deep Learning.
IEEE Trans. Geosci. Remote. Sens.(2023)
摘要
Wave runup observations are key data for understanding coastal response to storms. Lidar scanners are capable of collecting swash elevation data at high spatial and temporal resolution in a range of environmental conditions. Efforts to develop automated algorithms that effectively separate returns off of the beach and the sea surface are complicated by environmental noise, thus requiring time-intensive data quality control or manual digitization. In this study, a fully convolutional neural network (FCNN) was trained and validated on 966 30-min lidar linescan time series of the beach and swash zone and tested on an additional 99 30-min linescan time series to improve the automated classification of lidar returns off of the beach and water, facilitating the extraction of a depth-defined wave runup time series. Lidar returns were classified as beach or water, and beach points at each cross-shore location were interpolated through time, creating a time-varying beach elevation surface that was used to calculate instantaneous swash depths. Runup was defined as the most landward position of 3-cm water depth through time. Overall, the runup time series determined using the manually and machine learning (ML)-digitized beach-water interface agreed well (0.02-m root-mean-square difference (RMSD) 3-cm contour location and 0.06-m RMSD 2% runup exceedance elevation R2%). The trained model was found to be robust to noise and moderate data gaps and applicable in a range of wave conditions. Results demonstrate the potential of the ML model to replace manual data processing steps and significantly reduce the time and effort required to extract the instantaneous runup location from lidar linescan time series.
更多查看译文
关键词
Machine learning (ML) algorithms,oceans,remote sensing
AI 理解论文
溯源树
样例
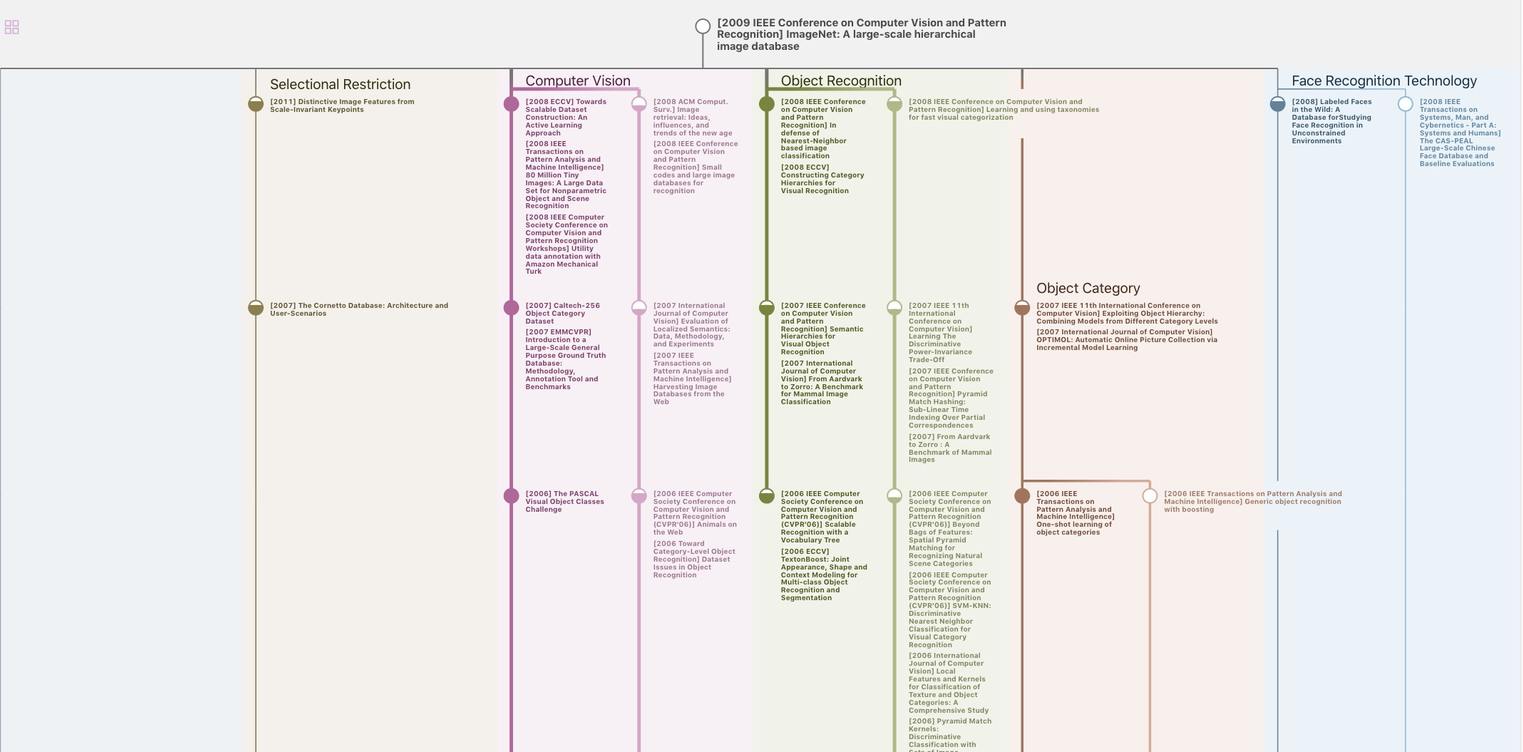
生成溯源树,研究论文发展脉络
Chat Paper
正在生成论文摘要