SAR Image Classification Using Gated Channel Attention Based Convolutional Neural Network.
Remote. Sens.(2023)
摘要
Algorithms combining CNN (Convolutional Neural Network) and super-pixel based smoothing have been proposed in recent years for Synthetic Aperture Radar (SAR) image classification. However, the smoothing may lead to the damage of details. To solve this problem the feature fusion strategy is utilized, and a novel adaptive fusion module named Gated Channel Attention (GCA) is designed in this paper. In this module, the relevance between channels is embedded into the conventional gated attention module to emphasize the variation in contribution on classification results between channels of feature-maps, which is not well considered by the conventional gated attention module. A GCA-CNN network is then constructed for SAR image classification. In this network, feature-maps corresponding to the original image and the smoothed image are extracted, respectively, by feature-extraction layers and adaptively fused. The fused features are used to obtain the results. Classification can be performed by the GCA-CNN in an end-to-end way. By the adaptive feature fusion in GCA-CNN, the smoothing of misclassification and the detail keeping can be realized at the same time. Experiments have been performed on one elaborately designed synthetic image and three real world SAR images. The superiority of the GCA-CNN is demonstrated by comparing with the conventional algorithms and the relative state-of-the-art algorithms.
更多查看译文
关键词
gated channel attention mechanism,adaptive feature fusion,smoothing of misclassification,detail keeping
AI 理解论文
溯源树
样例
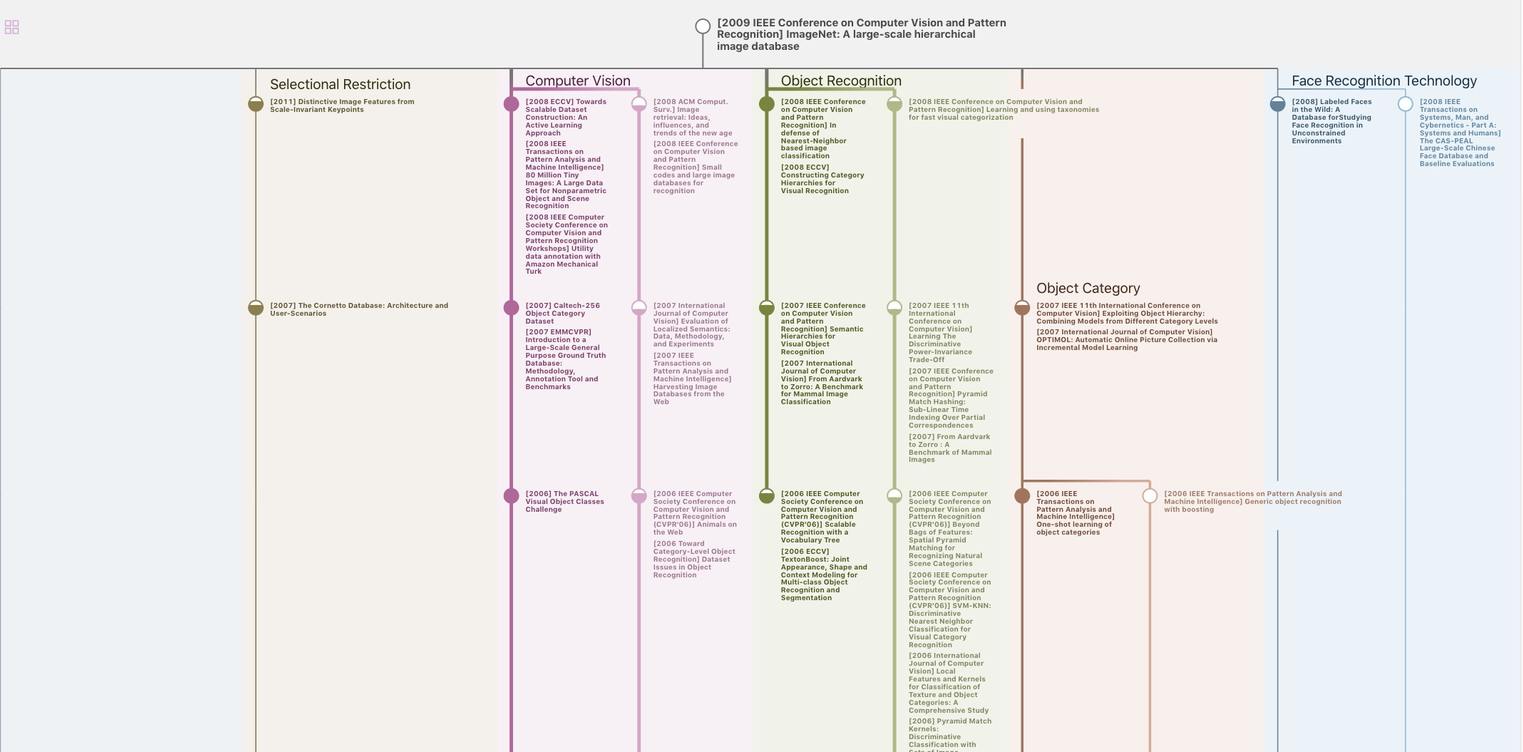
生成溯源树,研究论文发展脉络
Chat Paper
正在生成论文摘要