ModelarDB: Integrated Model-Based Management of Time Series from Edge to Cloud.
Trans. Large Scale Data Knowl. Centered Syst.(2022)
摘要
Abstract To ensure critical infrastructure is operating as expected, high-quality sensors are increasingly installed. However, due to the enormous amounts of high-frequency time series they produce, it is impossible or infeasible to transfer or even store these time series in the cloud when using state-of-the-practice compression methods. Thus, simple aggregates, e.g., 1–10-minutes averages, are stored instead of the raw time series. However, by only storing these simple aggregates, informative outliers and fluctuations are lost. Many Time Series Management System (TSMS) have been proposed to efficiently manage time series, but they are generally designed for either the edge or the cloud. In this paper, we describe a new version of the open-source model-based TSMS ModelarDB. The system is designed to be modular and the same binary can be efficiently deployed on the edge and in the cloud. It also supports continuously transferring high-frequency time series compressed using models from the edge to the cloud. We first provide an overview of ModelarDB, analyze the requirements and limitations of the edge, and evaluate existing query engines and data stores for use on the edge. Then, we describe how ModelarDB has been extended to efficiently manage time series on the edge, a novel file-based data store, how ModelarDB’s compression has been improved by not storing time series that can be derived from base time series, and how ModelarDB transfers high-frequency time series from the edge to the cloud. As the work that led to ModelarDB began in 2015, we also reflect on the lessons learned while developing it.
更多查看译文
关键词
time series,cloud,edge,model-based
AI 理解论文
溯源树
样例
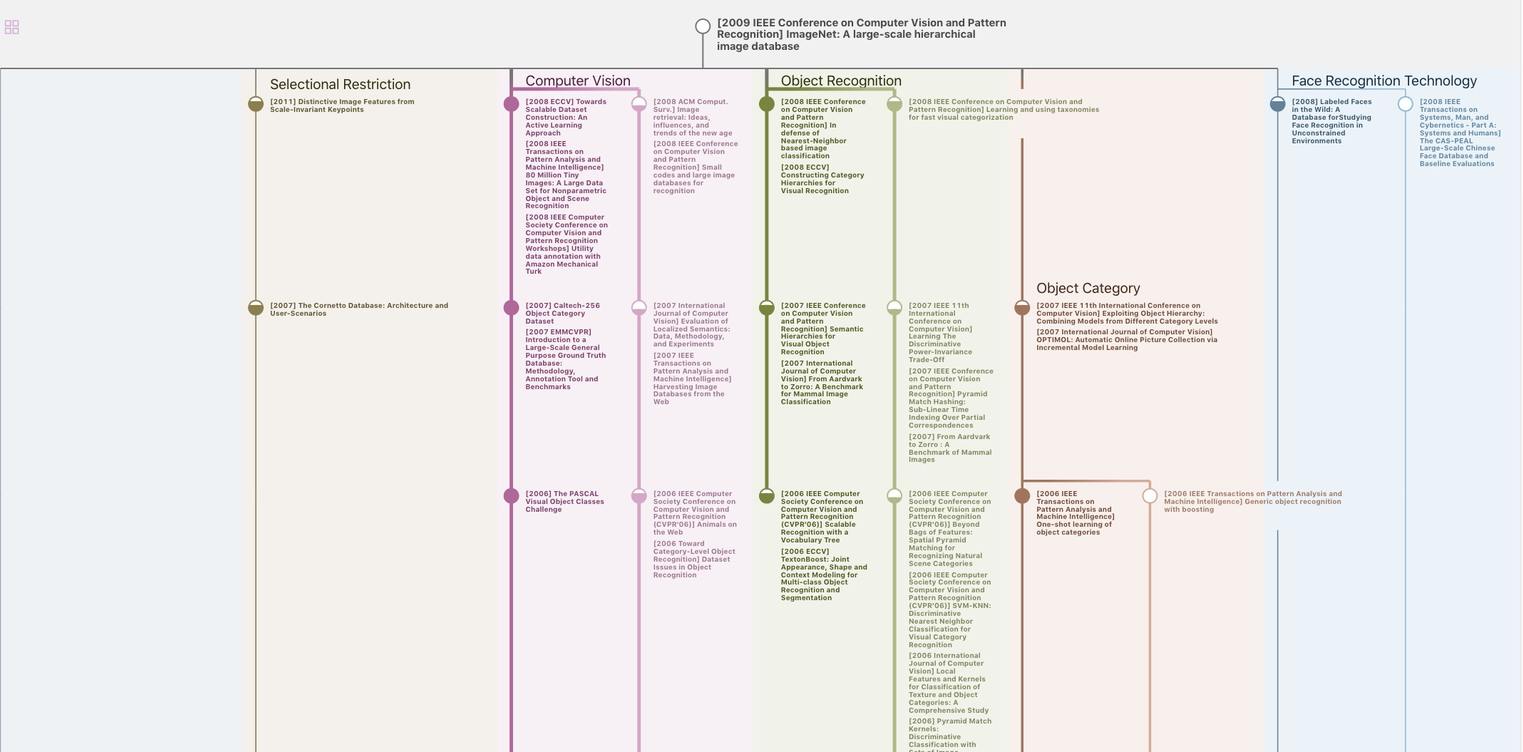
生成溯源树,研究论文发展脉络
Chat Paper
正在生成论文摘要