Cocktail Hubert: Generalized Self-Supervised Pre-Training for Mixture and Single-Source Speech
arxiv(2023)
摘要
Self-supervised learning leverages unlabeled data effectively, improving label efficiency and generalization to domains without labeled data. While recent work has studied generalization to more acoustic/linguistic domains, languages, and modalities, these investigations are limited to single-source speech with one primary speaker in the recording. This paper presents Cocktail HuBERT, a self-supervised learning framework that generalizes to mixture speech using a masked pseudo source separation objective. This objective encourages the model to identify the number of sources, separate and understand the context, and infer the content of masked regions represented as discovered units. Cocktail HuBERT outperforms state-of-the-art results with 69% lower WER on multi-speaker ASR, 31% lower DER on diarization, and is competitive on single-and multi-speaker tasks from SUPERB.
更多查看译文
关键词
Self-supervised pre-training,diarization,multispeaker ASR,source separation,cocktail party,mixture speech
AI 理解论文
溯源树
样例
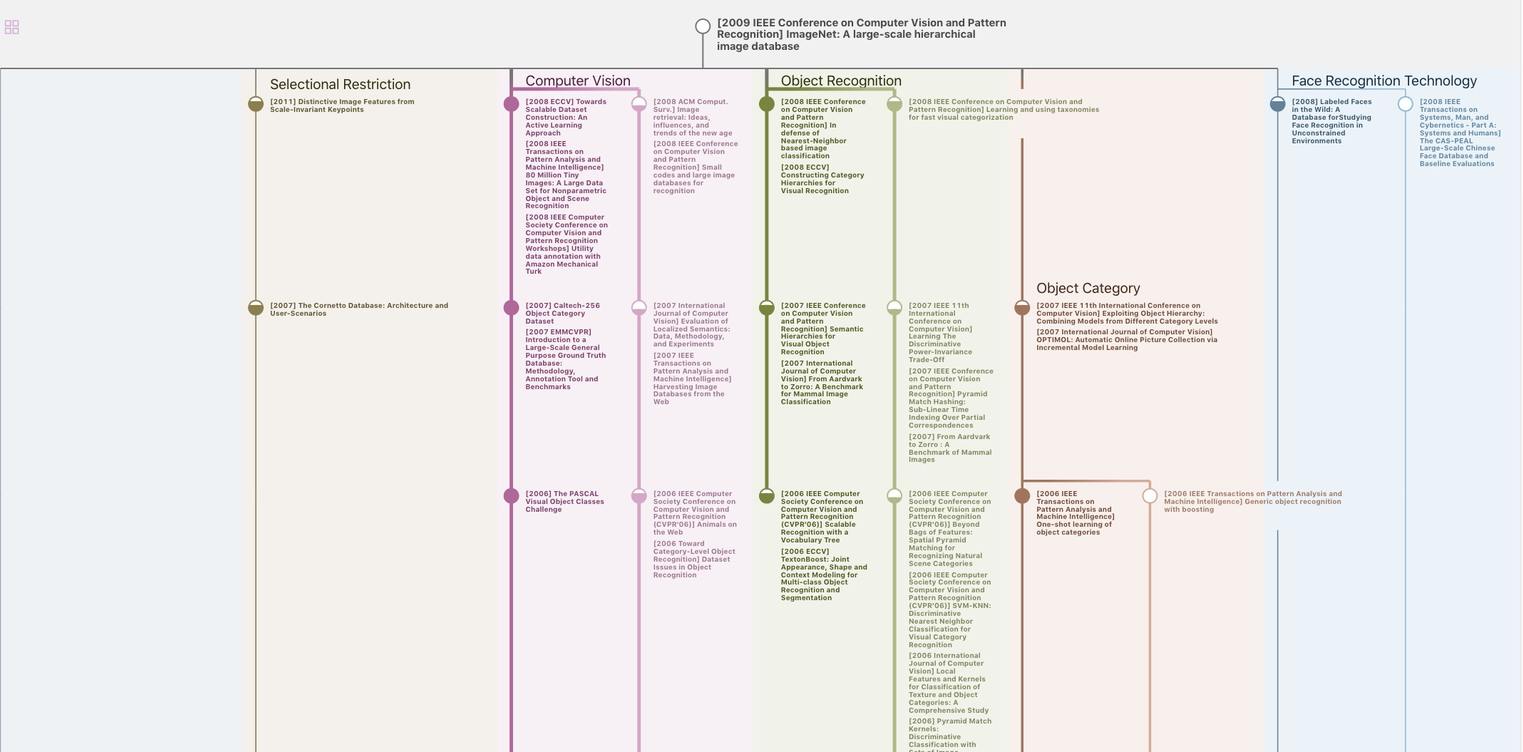
生成溯源树,研究论文发展脉络
Chat Paper
正在生成论文摘要