Differentially Private Algorithms for Synthetic Power System Datasets
arxiv(2023)
摘要
While power systems research relies on the availability of real-world network datasets, data owners (e.g., system operators) are hesitant to share data due to security and privacy risks. To control these risks, we develop privacy-preserving algorithms for the synthetic generation of optimization and machine learning datasets. Taking a real-world dataset as input, the algorithms output its noisy, synthetic version, which preserves the accuracy of the real data on a specific downstream model or even a large population of those. We control the privacy loss using Laplace and Exponential mechanisms of differential privacy and preserve data accuracy using a post-processing convex optimization. We apply the algorithms to generate synthetic network parameters and wind power data.
更多查看译文
关键词
private algorithms,synthetic power system datasets
AI 理解论文
溯源树
样例
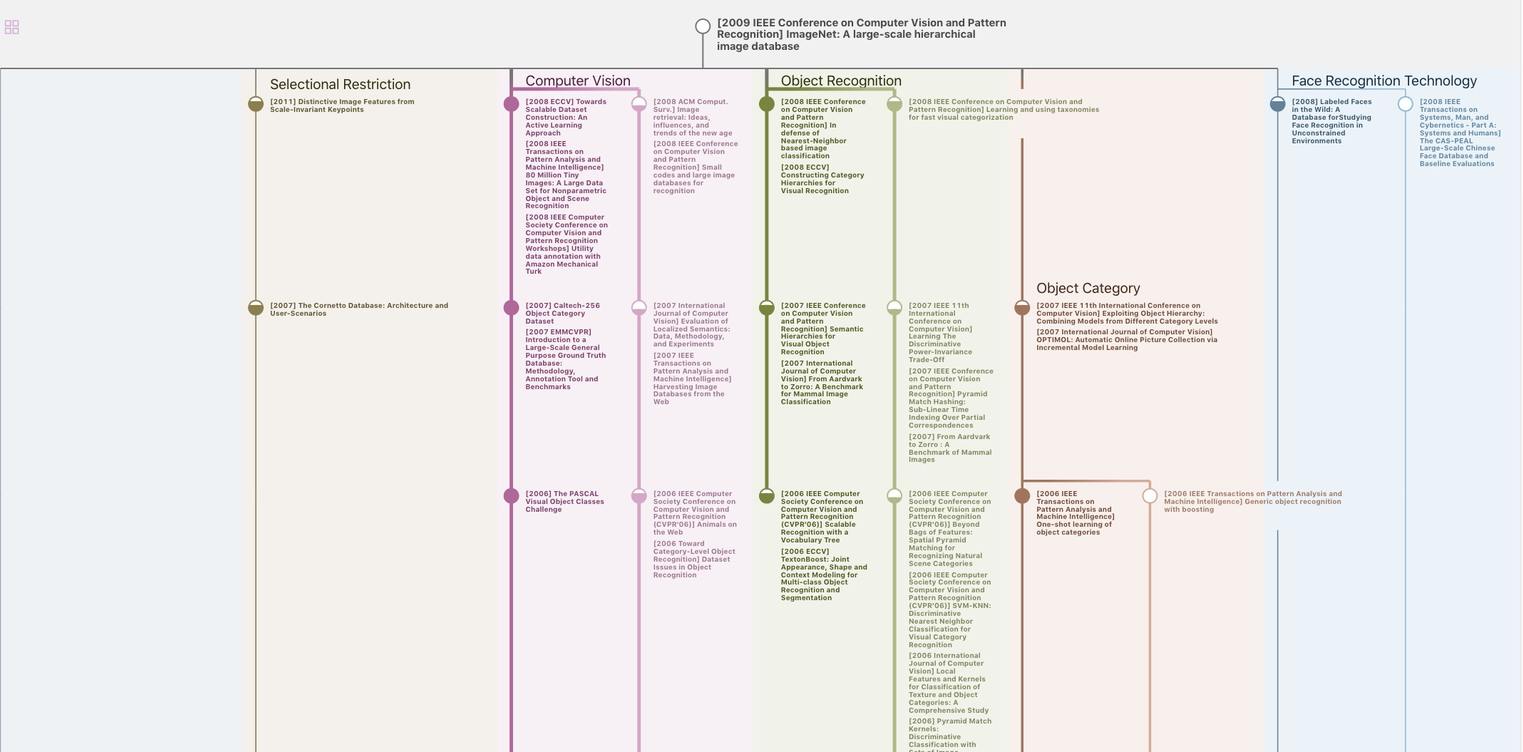
生成溯源树,研究论文发展脉络
Chat Paper
正在生成论文摘要