Learning to Explore Informative Trajectories and Samples for Embodied Perception
2023 IEEE INTERNATIONAL CONFERENCE ON ROBOTICS AND AUTOMATION, ICRA(2023)
摘要
We are witnessing significant progress on perception models, specifically those trained on large-scale internet images. However, efficiently generalizing these perception models to unseen embodied tasks is insufficiently studied, which will help various relevant applications (e.g., home robots). Unlike static perception methods trained on pre-collected images, the embodied agent can move around in the environment and obtain images of objects from any viewpoints. Therefore, efficiently learning the exploration policy and collection method to gather informative training samples is the key to this task. To do this, we first build a 3D semantic distribution map to train the exploration policy self-supervised by introducing the semantic distribution disagreement and the semantic distribution uncertainty rewards. Note that the map is generated from multi-view observations and can weaken the impact of misidentification from an unfamiliar viewpoint. Our agent is then encouraged to explore the objects with different semantic distributions across viewpoints, or uncertain semantic distributions. With the explored informative trajectories, we propose to select hard samples on trajectories based on the semantic distribution uncertainty to reduce unnecessary observations that can be correctly identified. Experiments show that the perception model fine-tuned with our method outperforms the baselines trained with other exploration policies. Further, we demonstrate the robustness of our method in real-robot experiments.
更多查看译文
关键词
Embodied Perception,Trajectory Exploration,Hard Sample Selection
AI 理解论文
溯源树
样例
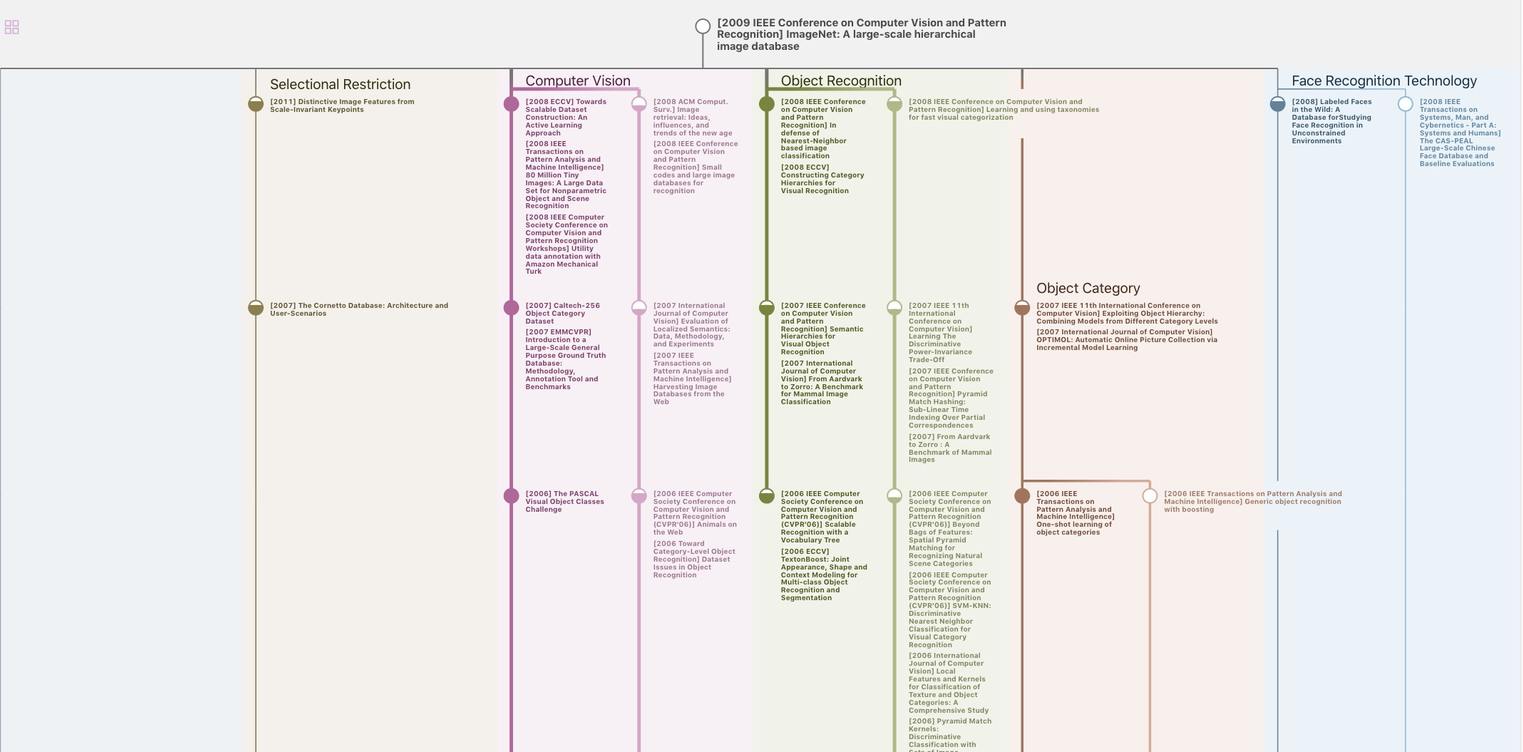
生成溯源树,研究论文发展脉络
Chat Paper
正在生成论文摘要