PheME: A deep ensemble framework for improving phenotype prediction from multi-modal data
arxiv(2023)
摘要
Detailed phenotype information is fundamental to accurate diagnosis and risk estimation of diseases. As a rich source of phenotype information, electronic health records (EHRs) promise to empower diagnostic variant interpretation. However, how to accurately and efficiently extract phenotypes from the heterogeneous EHR data remains a challenge. In this work, we present PheME, an Ensemble framework using Multi-modality data of structured EHRs and unstructured clinical notes for accurate Phenotype prediction. Firstly, we employ multiple deep neural networks to learn reliable representations from the sparse structured EHR data and redundant clinical notes. A multi-modal model then aligns multi-modal features onto the same latent space to predict phenotypes. Secondly, we leverage ensemble learning to combine outputs from single-modal models and multi-modal models to improve phenotype predictions. We chose seven diseases to evaluate the phenotyping performance of the proposed framework. Experimental results show that using multi-modal data significantly improves phenotype prediction in all diseases, the proposed ensemble learning framework can further boost the performance.
更多查看译文
关键词
Phenotype Prediction,Multi-Modal Data,Ensemble Learning,Natural Language Processing
AI 理解论文
溯源树
样例
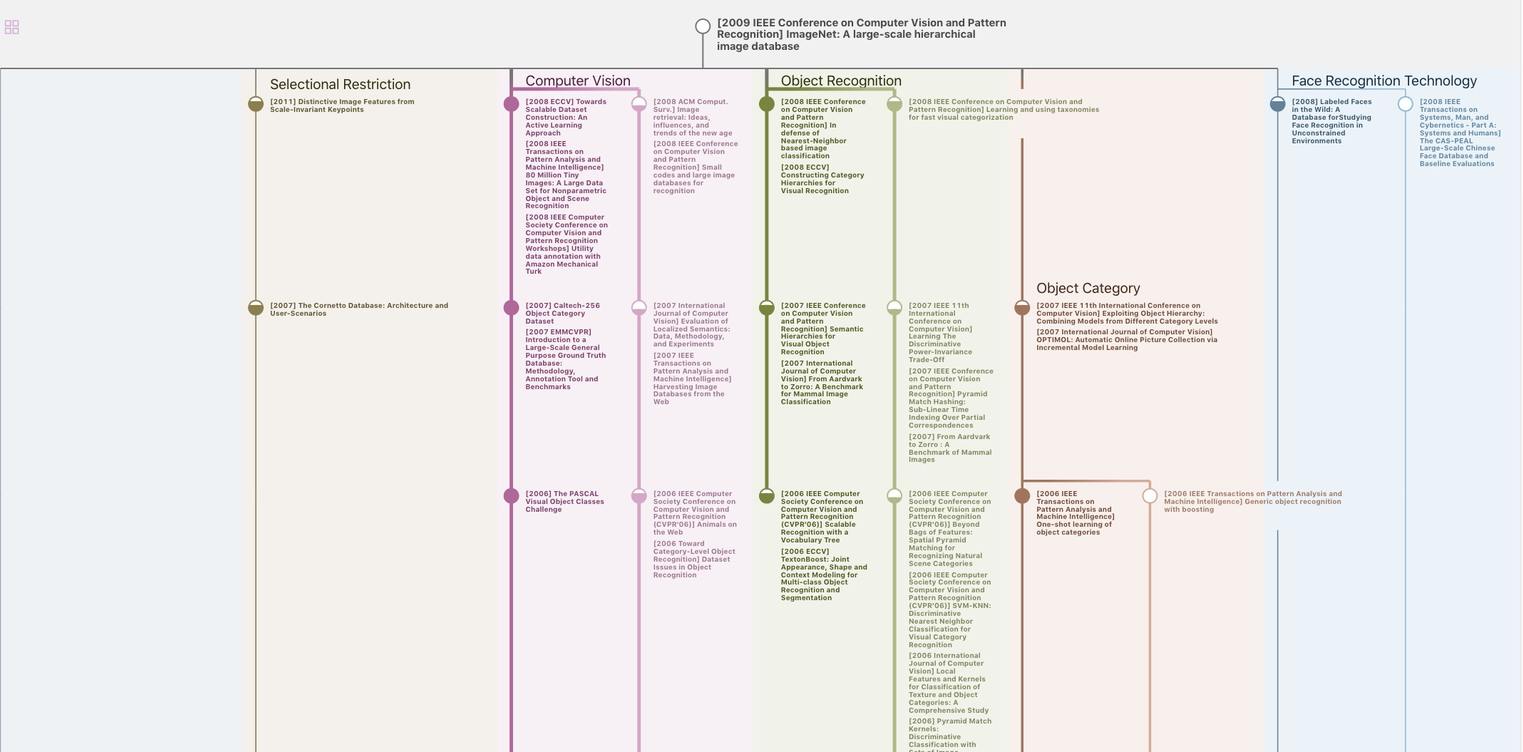
生成溯源树,研究论文发展脉络
Chat Paper
正在生成论文摘要